Predicting drug transcriptional response similarity using Signed Graph Convolutional Network.
BIBM(2022)
摘要
Exploring the transcriptional response after employing chemical compounds assists in treating gene-related diseases and understanding biological activity of compounds. Calculating the similarity of drug transcriptional response can help to discover novel compounds that have the similar biological activity to known drugs for treating the same disease. Considering the transcriptional profiles of compounds are limited and harder to get than the structure of compounds, it is worth modeling the structure-transcriptional response similarity relationship. In this paper, we propose a signed graph convolutional network (SGCN)-based method, namely SGCN-DTRS, to predict drug transcriptional response similarity, which is quantitatively measured by Connectivity Map (CMap) scores, from their structures. SGCNDTRS constructs a CMap signed network from compounds and their CMap scores in the training data, which takes compounds as nodes, molecular structural representations of compounds as the attributes of nodes, and similarity relations between compounds as edges. Then SGCN-DTRS learns the CMap compound embeddings to predict CMap scores of pairwise compounds. Extensive experiments verify the superiority of the proposed method against the compared state-of-the-art methods and reveal that the relational information of the CMap data, which is learned from CMap signed network, is important for the CMap score prediction. SGCN-DTRS can not only work for the compounds in the training set but also is applicable to unseen compounds.
更多查看译文
关键词
drug transcriptional response,similarity,CMap score,signed graph neural network
AI 理解论文
溯源树
样例
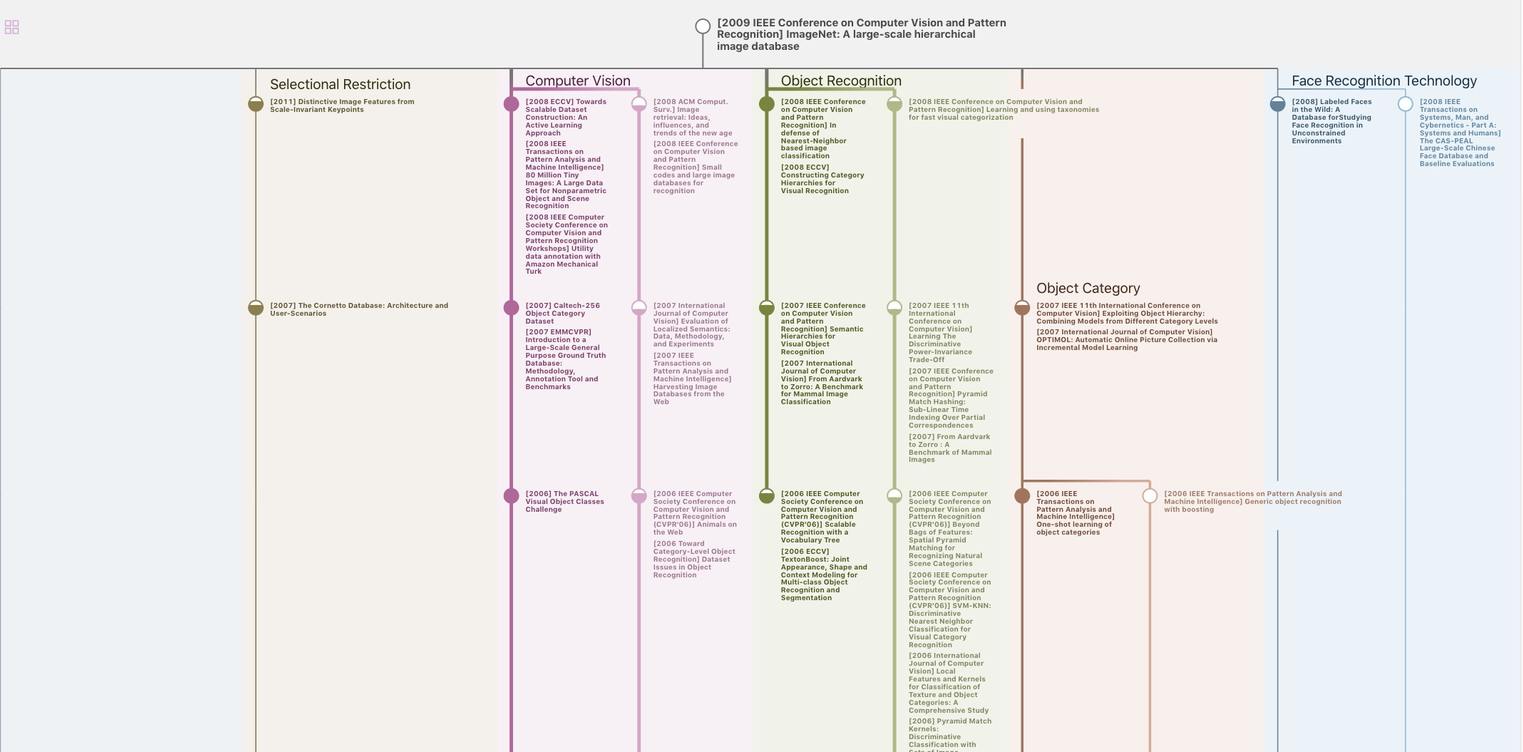
生成溯源树,研究论文发展脉络
Chat Paper
正在生成论文摘要