Sequential pattern detection for identifying courses of treatment and anomalous claim behaviour in medical insurance
BIBM(2022)
摘要
Fraud and waste is a costly problem in medical insurance. Utilising sequence information for anomaly detection is under-explored in this domain. We present a multi-part method employing sequential pattern mining for identifying and grouping comparable courses of treatment, finding patterns within those courses, calculating the cost of possible additional or upcoded claims in unusual patterns, and ranking the providers based on potential recoverable costs. We applied this method to real-world radiation therapy data. Results were assessed by experts at the Australian Government Department of Health, and were found to be interpretable and informative. Previously unknown anomalous claim patterns were discovered, and confirmation of a previously suspected anomalous claim pattern was also obtained. Outlying providers each claimed up to ${\$}$486,617.60 in potentially recoverable costs. Our method was able to identify anomalous claims as well as the patterns in which they were anomalous, making the results easily interpretable. The method is currently being implemented for another problem involving sequential data at the Department of Health.
更多查看译文
关键词
unsupervised learning,anomaly detection,medical information systems,data mining,decision support systems
AI 理解论文
溯源树
样例
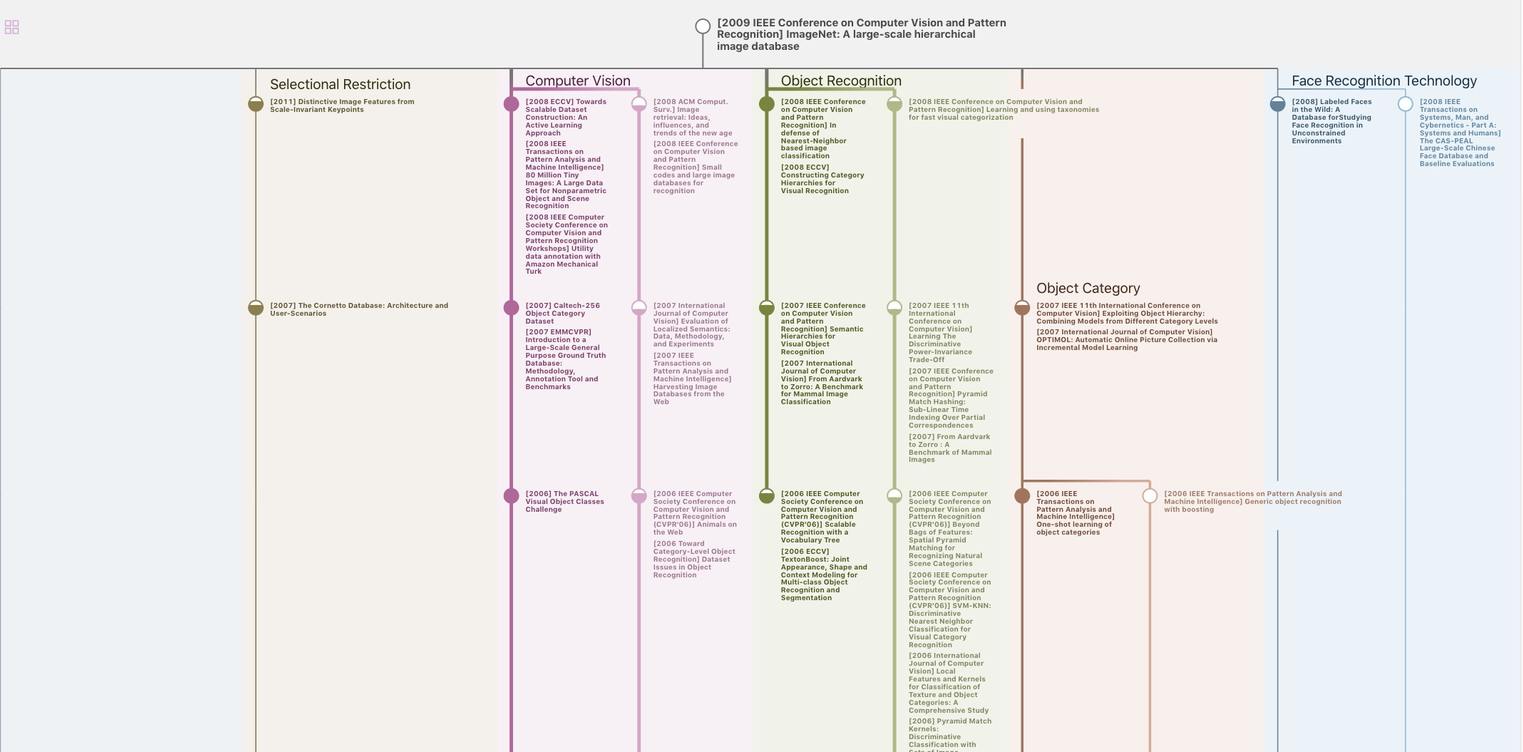
生成溯源树,研究论文发展脉络
Chat Paper
正在生成论文摘要