Subpopulation Analysis in Causal Inference: A Healthcare Case Study
BIBM(2022)
摘要
Treatment interventions are usually targeted to improve a specific outcome on as elected group of patients who are eligible to receive the treatment. The success of such treatments is determined by the post-intervention treatment effect on the population under consideration. There are cases when the treatment group contains multiple categories of eligible populations, with various effects, especially when the study’s criteria are loosely defined. I n such s tudies (non-targeted trials) non-eligible subjects may be treated, producing heterogeneous treatment effects within the treated group. Inferring the effectiveness of the treatment under this scenario is difficult since the average treatment effect on the treated is a combination of multiple effect levels. This can bias the resulting conclusion of the causal studies. We propose an end-to-end framework based on matching and unsupervised clustering for extracting population sub-groups based on their effect levels. We demonstrate our methods on a real-world healthcare application, highlighting the value of subpopulation analysis for recovering multiple effect groups.
更多查看译文
关键词
Causal Inference,Observational Studies,Subgroup Analysis
AI 理解论文
溯源树
样例
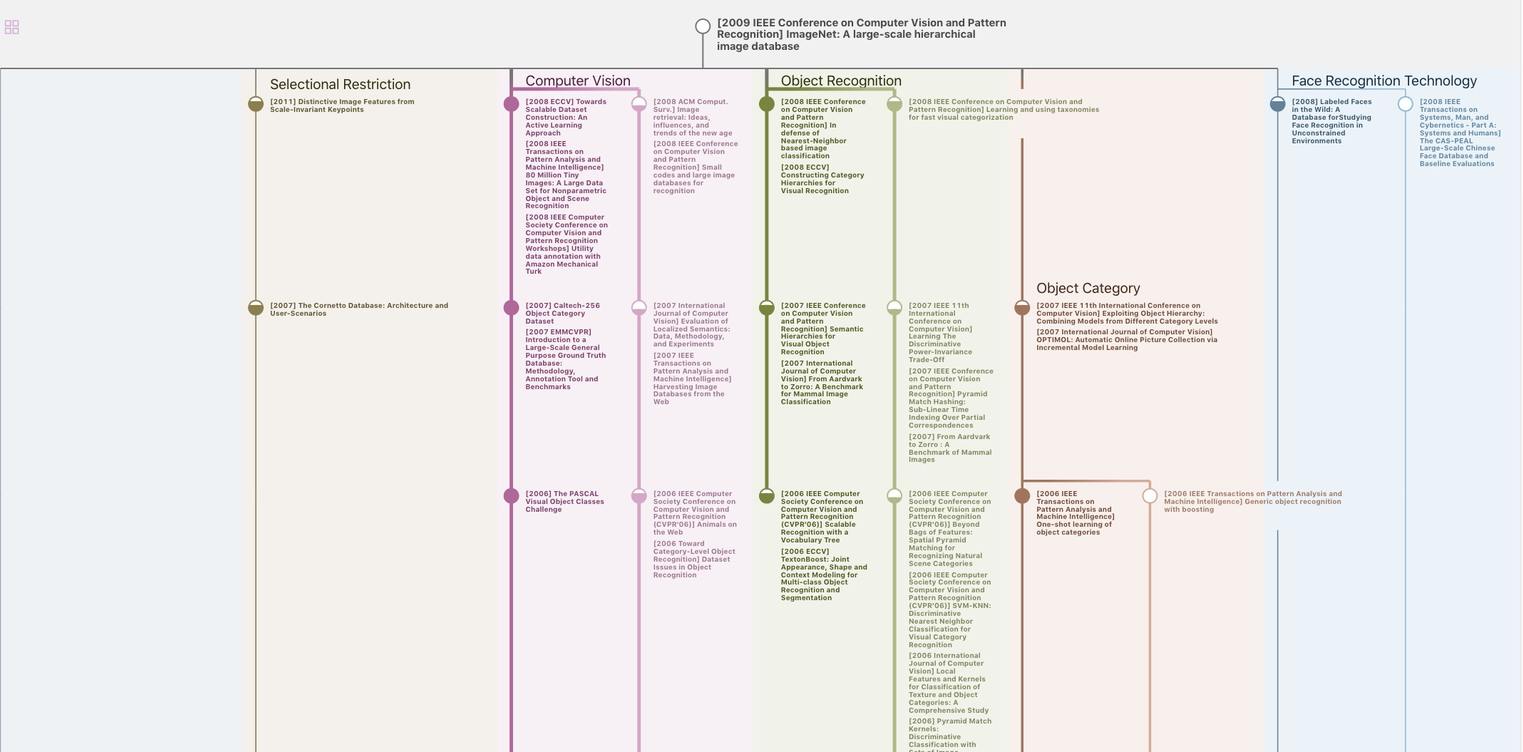
生成溯源树,研究论文发展脉络
Chat Paper
正在生成论文摘要