Gradient-Based Severity Labeling for Biomarker Classification in Oct.
ICIP(2022)
摘要
In this paper, we propose a novel selection strategy for contrastive learning for medical images. On natural images, contrastive learning uses augmentations to select positive and negative pairs for the contrastive loss. However, in the medical domain, arbitrary augmentations have the potential to distort small localized regions that contain the biomarkers we are interested in detecting. A more intuitive approach is to select samples with similar disease severity characteristics, since these samples are more likely to have similar structures related to the progression of a disease. To enable this, we introduce a method that generates disease severity labels for unlabeled OCT scans on the basis of gradient responses from an anomaly detection algorithm. These labels are used to train a supervised contrastive learning setup to improve biomarker classification accuracy by as much as 6% above self-supervised baselines for key indicators of Diabetic Retinopathy.
更多查看译文
关键词
Retinal Biomarkers, Contrastive Learning, Gradients
AI 理解论文
溯源树
样例
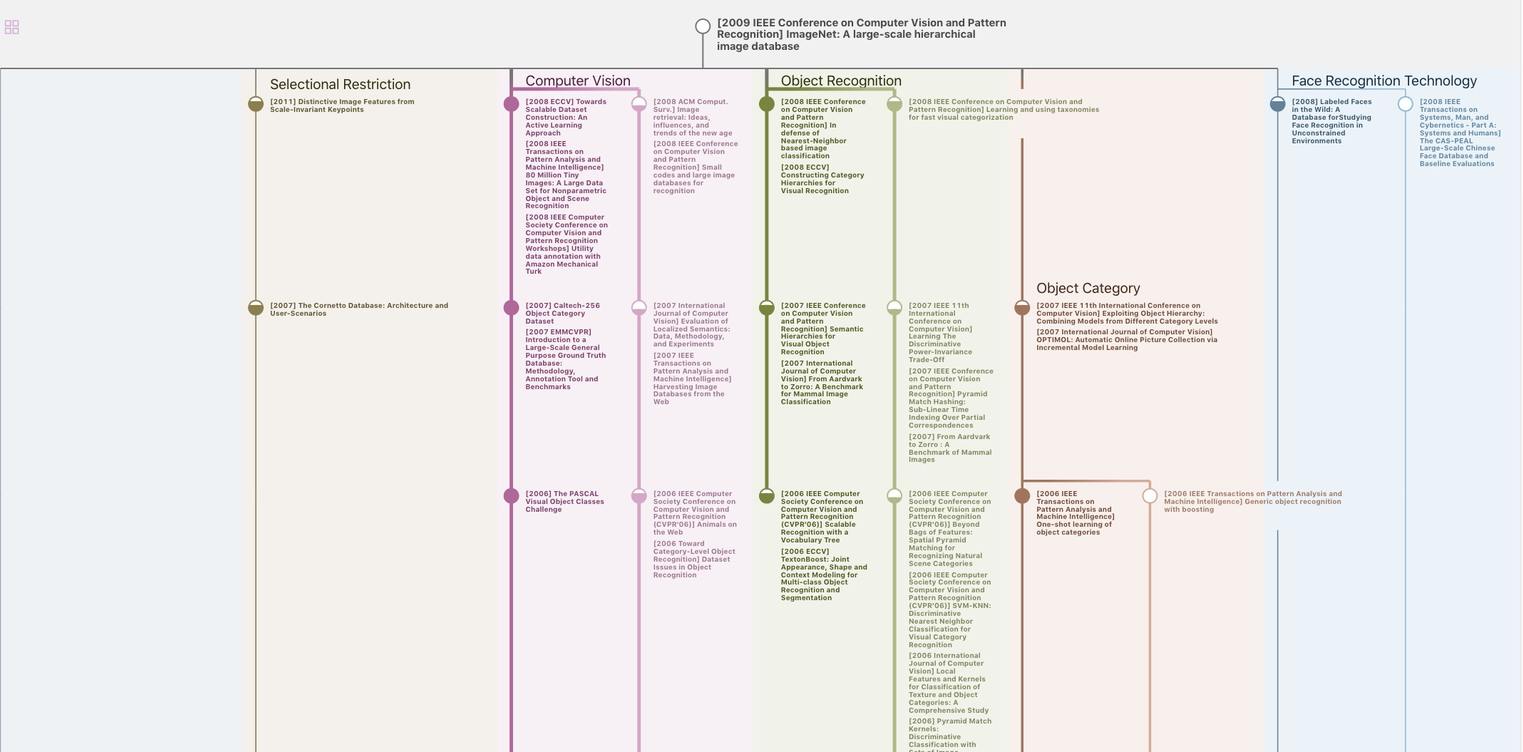
生成溯源树,研究论文发展脉络
Chat Paper
正在生成论文摘要