Skip-MLP Network for Point Cloud Classification.
ICIP(2022)
摘要
The encoding method for local neighborhood of sampling points is the bottleneck of point cloud learning. Existing encoding methods do not perform well on point clouds with low density, which seriously affects its application in real-world scenarios. In this paper, we propose a novel encoding method for local neighborhood of sampling points, named Skip-MLP. It can establish the spatial relationship among sampling points while encoding the local neighborhood. We simultaneously adopt a point sampling scheme based on learning, in order to enhance the adaptability of the sampling points selection strategy with point cloud density changing. Moreover, an attention module is introduced to perform fine-grained encoding of the key features of the embedded sampling points neighborhoods. Experimental results on benchmarks demonstrate that Skip-MLP network is more efficient and robust than the previous methods for point cloud classification.
更多查看译文
关键词
Point clouds, residual structure, adaptive sample, classification
AI 理解论文
溯源树
样例
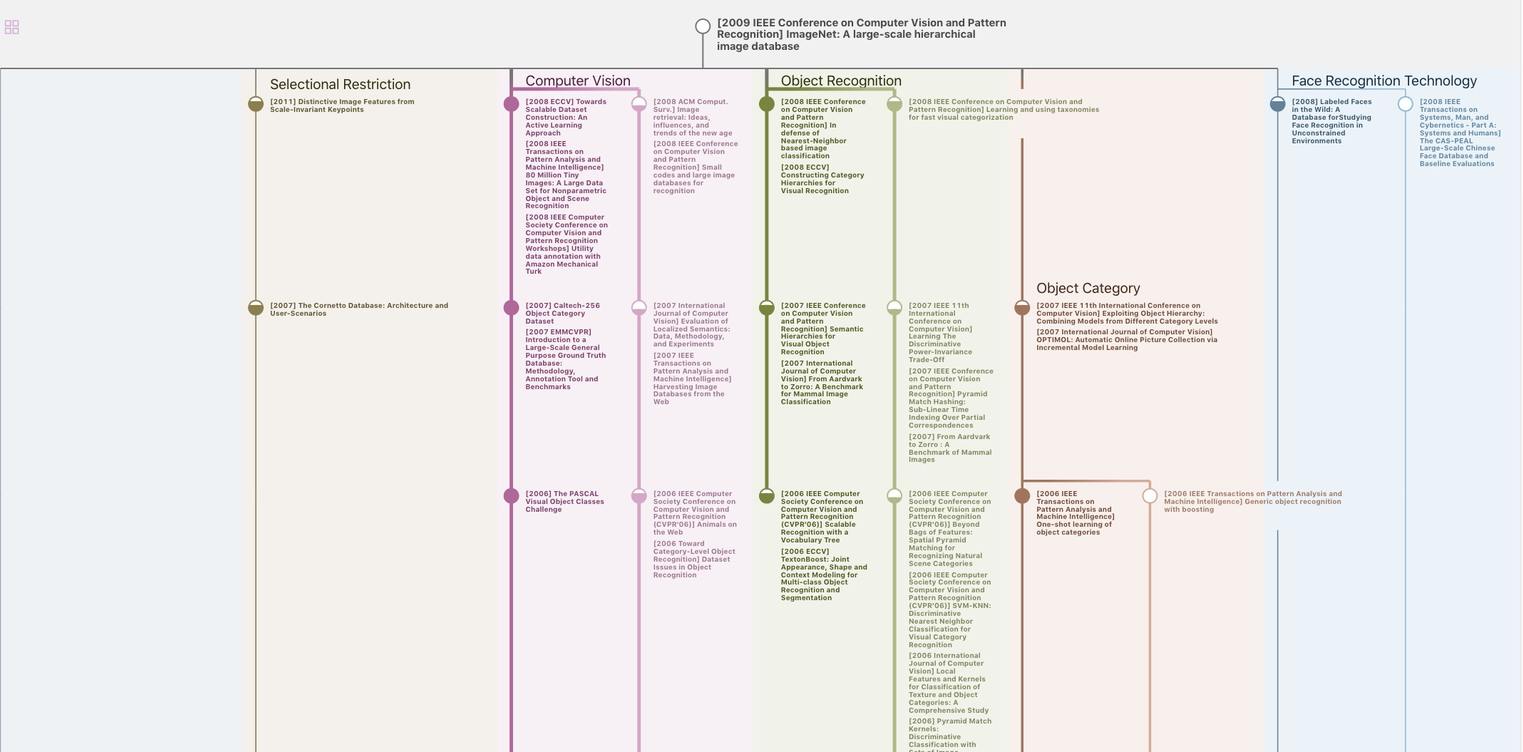
生成溯源树,研究论文发展脉络
Chat Paper
正在生成论文摘要