Dose-Blind Denoising With Deep Learning in Cardiac Spect.
ICIP(2022)
Abstract
Deep learning denoising methods have been found to be effective for noise suppression in reduced-dose studies in medical imaging applications. In this work, we investigate the feasibility of improving the generalizability of a denoising network by using a dose-blind training approach, in which the network is trained with a loss function defined to accommodate the varying data statistics associated with multiple reduced-dose levels. In the experiments, we demonstrated this approach on quarter- and eighth-dose data from a set of 895 clinical cardiac SPECT perfusion imaging acquisitions. The quantitative results show that a dose-blind denoising network could generalize well over both dose levels, and outperformed dose-specific training in detection of perfusion defects at both quarter- and eighth-dose data (p-values < 10(-4); paired t-test).
MoreTranslated text
Key words
SPECT-MPI, dose reduction, deep learning generalizability, dose-blind training
AI Read Science
Must-Reading Tree
Example
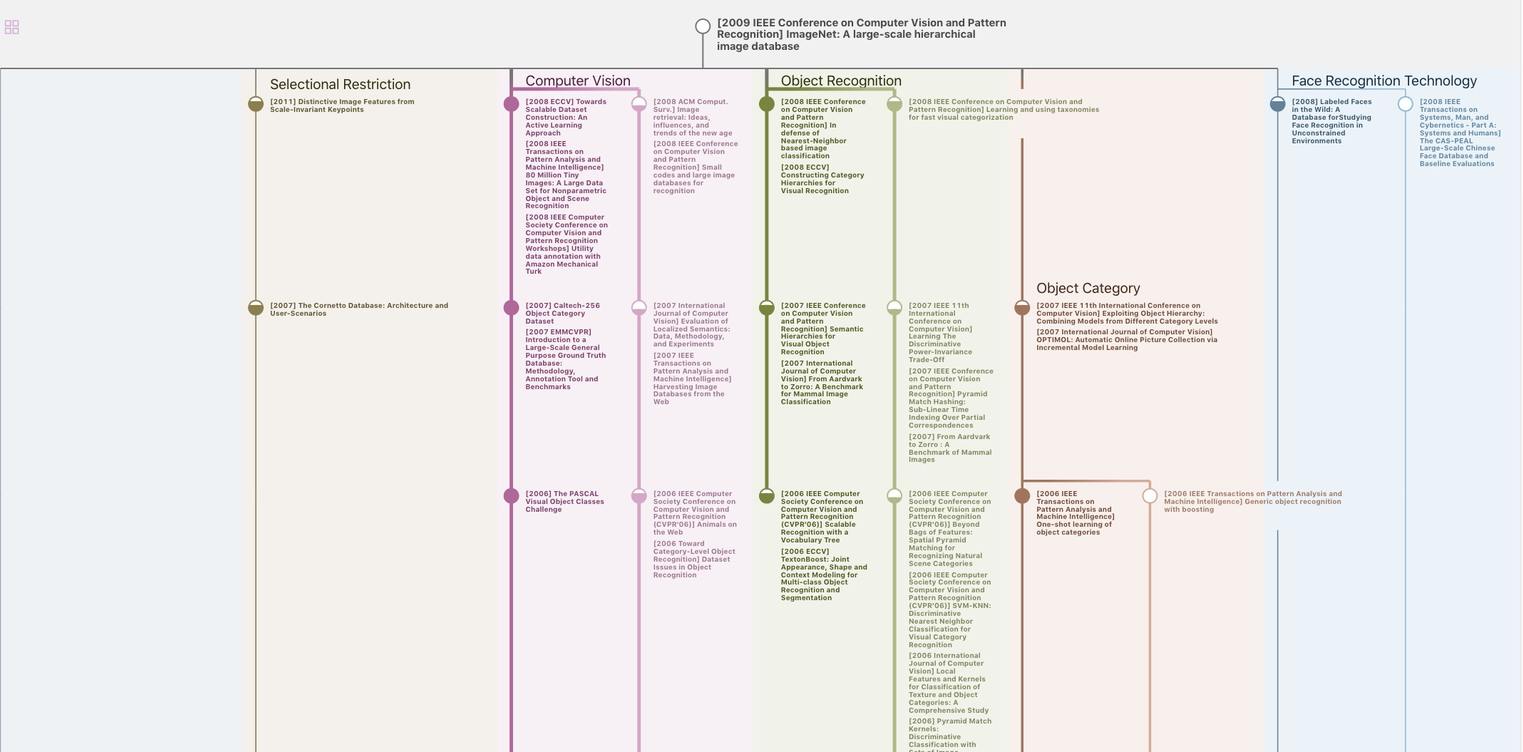
Generate MRT to find the research sequence of this paper
Chat Paper
Summary is being generated by the instructions you defined