Pyramid Knowledge Distillation for Efficient Human Pose Estimation.
ICIP(2022)
摘要
Human pose estimation is an important task in many real-time applications. Existing methods directly slim the CNN by deploying well-designed lightweight modules. However, these methods lack privileged information guidance and the knowledge distillation technique stays less explored. In this work, we propose a novel method, namely Pyramid Knowledge Distillation (PKD) for efficient human pose estimation. Specifically, PKD composes of Pyramid Structured Map Distillation (PSMD) and Pyramid Feature Map Distillation (PFMD). In PSMD, we formulate a structured map encoding robust interjoint correlation. Based on structured map, the spatial dependencies between keypoints can be better transferred from a cumbersome teacher network to a compact student model. To further promote the efficiency of student, PFMD is used to distill rich local and global features from teacher. Experiments demonstrate that PKD achieves an optimal trade-off between cost and accuracy on COCO and MPII benchmarks, even with a much faster inference speed.
更多查看译文
关键词
Knowledge distillation,Deep learning,Human pose estimation
AI 理解论文
溯源树
样例
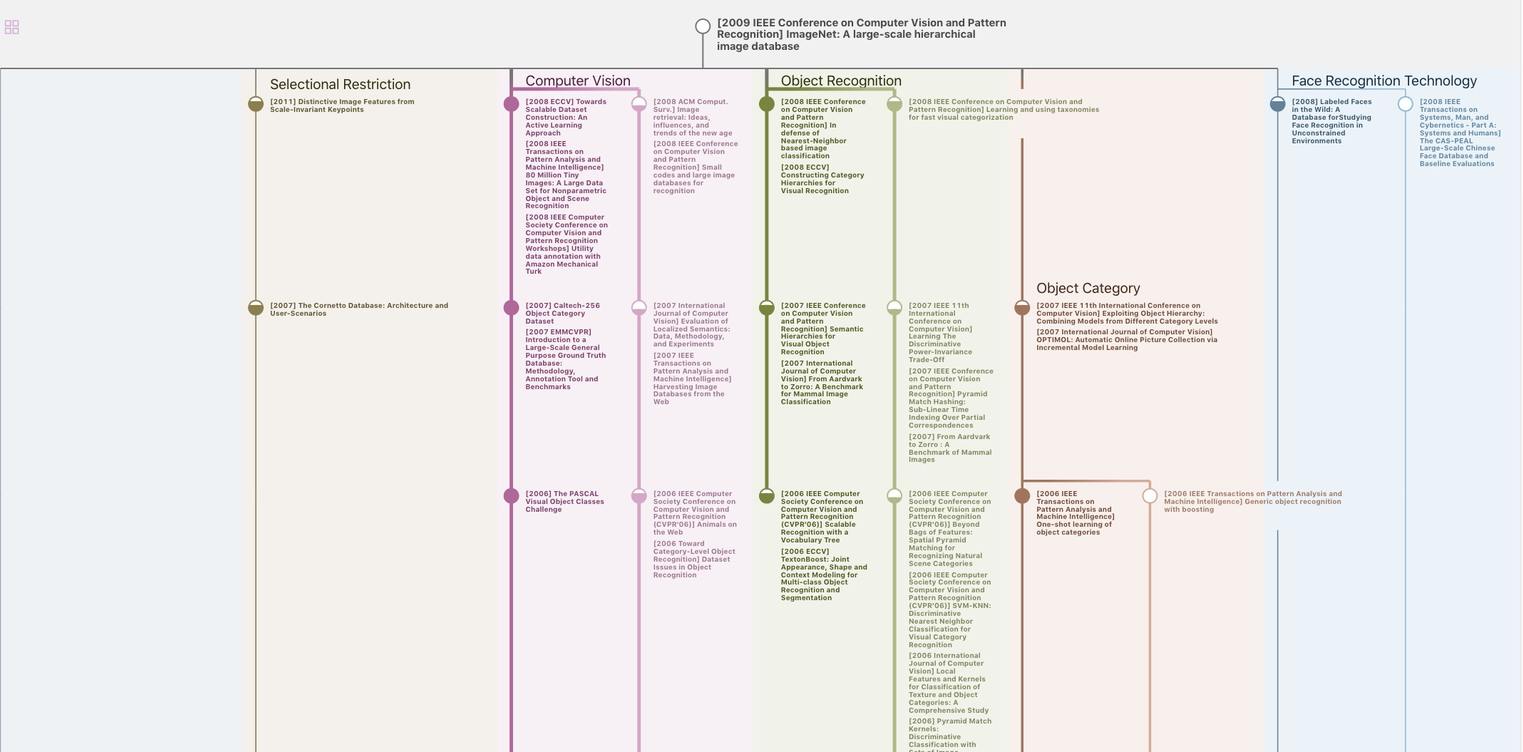
生成溯源树,研究论文发展脉络
Chat Paper
正在生成论文摘要