Semi-Supervised 3D Medical Image Segmentation Via Boundary-Aware Consistent Hidden Representation Learning.
ICIP(2022)
Abstract
This paper proposes a novel Boundary-aware Consistent Hidden Representation Learning Network (BA-CHRLN), which contains two branches for semi-supervised 3D medical image segmentation. Inspired by the contrastive learning, the two branches share the same encoder and each has its individual decoder, namely supervised decoder and unsupervised one. A stop-gradient operation is also utilized to prevent collapsing of solutions. Taking the unlabeled images as references, BA-CHRLN imposes the consistency by applying a perturbation on the high-level hidden feature representations, which significantly improves the encoder's representation and the network's robustness. A boundary-aware map is further introduced to capture the organ's boundary without any prior knowledge and additional parameters. Experiments on the Left Atrium (LA) benchmark dataset demonstrate the effectiveness of the BA-CHRLN.
MoreTranslated text
Key words
Boundary-aware, Hidden representation, Medical image, Semi-supervised segmentation
AI Read Science
Must-Reading Tree
Example
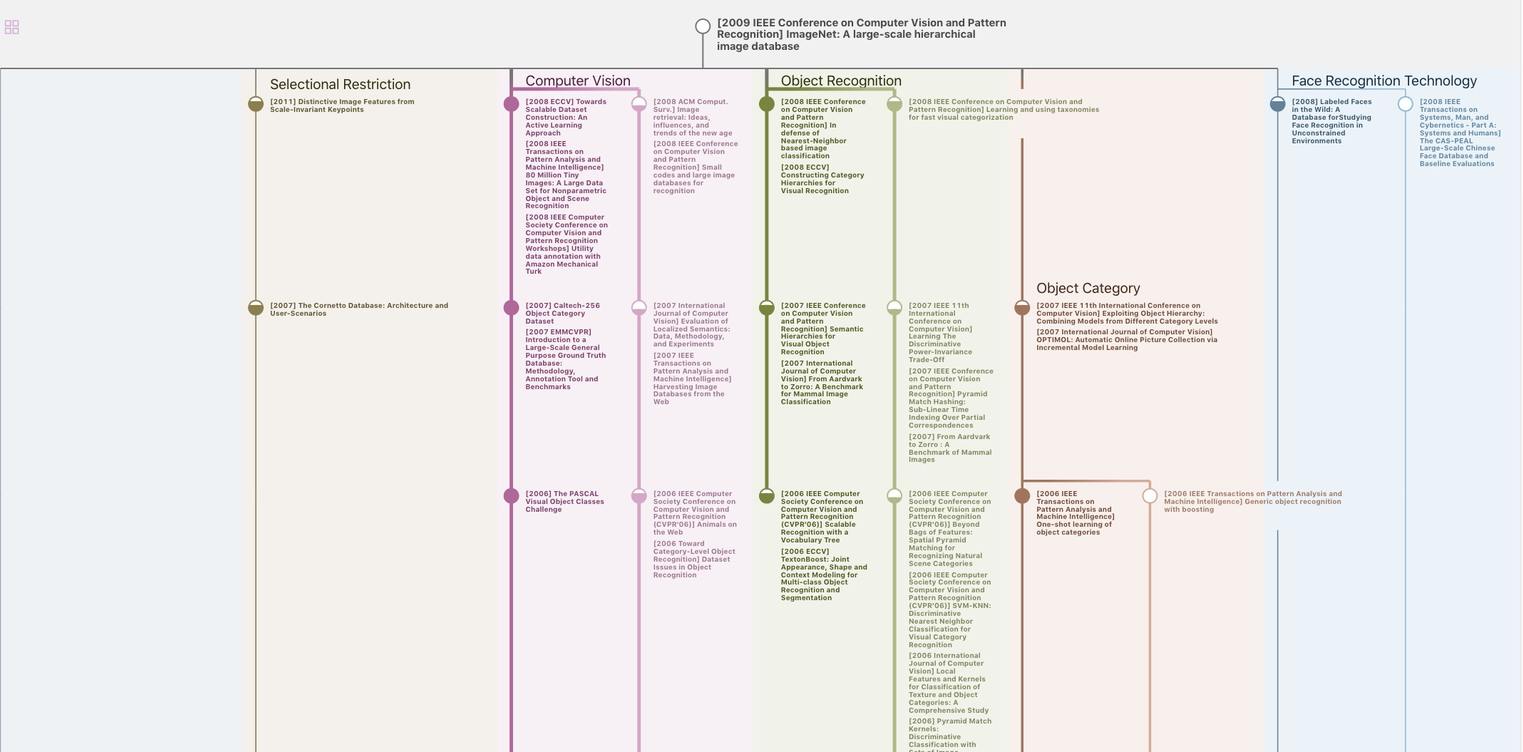
Generate MRT to find the research sequence of this paper
Chat Paper
Summary is being generated by the instructions you defined