MLS-GAN: Multi-Level Semantic Guided Image Colorization.
ICIP(2022)
Abstract
Image colorization predicts plausible color versions of given grayscale images. Recently, several methods incorporate image semantics to assist image colorization and have shown impressive performance. To further exploit and take full advantage of more semantic information, in this paper, we propose a Multi-Level Semantic guided Generative Adversarial Network (MLS-GAN) for image colorization. Specifically, we utilize three different levels of semantics to guide the colorization process: image level, segmentation level and contextual level. Image-level classification semantics is used to learn category and high-level semantics, ensuring the reasonability of color results. At the segmentation level, multi-scale saliency map semantics is extracted to provide figure-background separation information, which can efficiently alleviate semantic confusion, especially for images with complex backgrounds. Furthermore, we novelly use non-local blocks to capture long-range semantic dependencies at the contextual level. Experiments show that our method enhances color consistency and can produce more vivid color in visually important regions, outperforming state-of-the-art methods qualitatively and quantitatively.
MoreTranslated text
Key words
Image colorization, multi-level semantic guided, saliency map semantics, contextual semantics, classification semantics
AI Read Science
Must-Reading Tree
Example
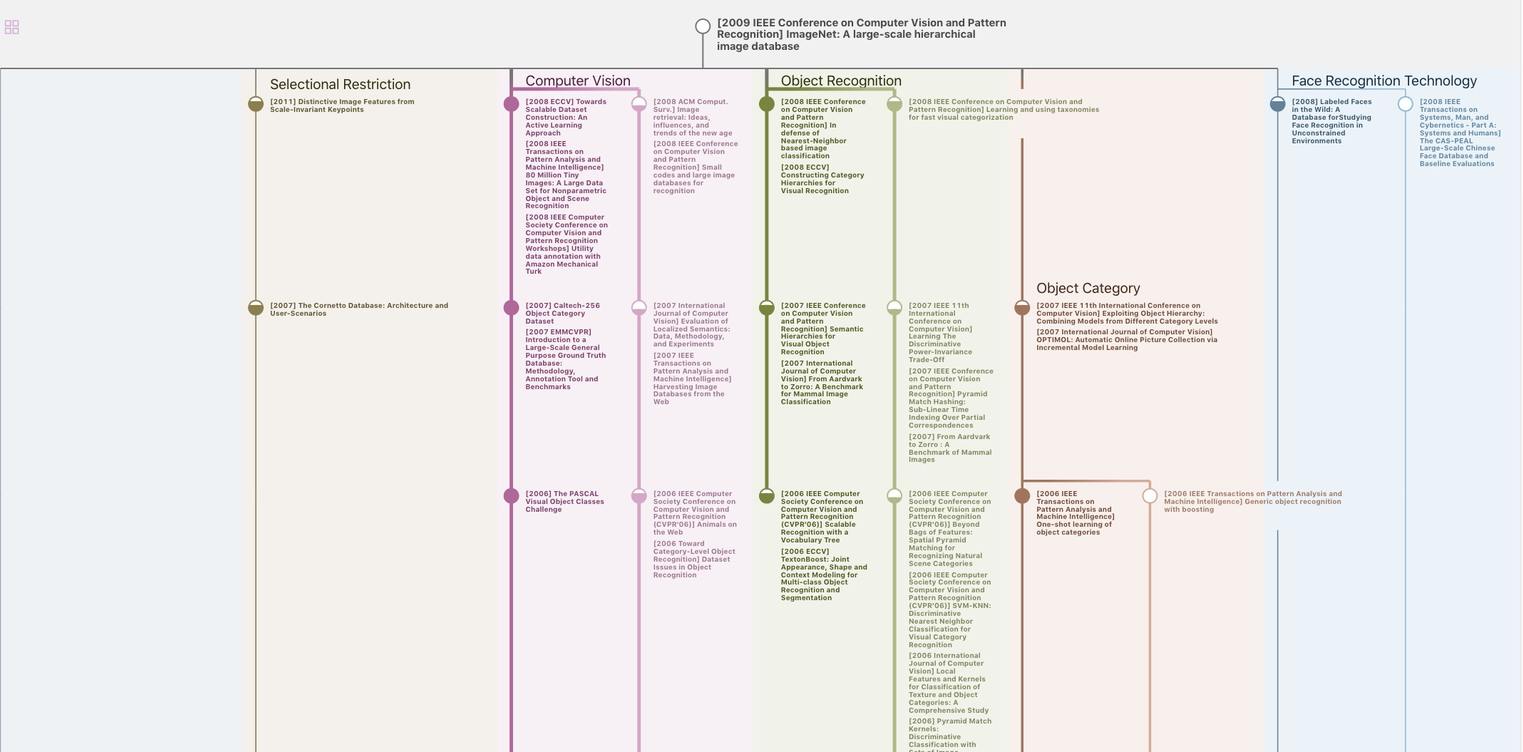
Generate MRT to find the research sequence of this paper
Chat Paper
Summary is being generated by the instructions you defined