Multi-Scale Transformer-Based Feature Combination for Image Retrieval.
ICIP(2022)
摘要
Image retrieval consists in the selection of a set of images from a database, based on their visual similarity to a given query image. To compute this visual similarity, a global or multiple local features are required per image. In this work, we present a system that uses multiple high-level semantic feature maps from a single image for global feature generation. Our method uses feature maps extracted from a backbone architecture at different spatial resolutions, enhancing the semantic information relevant at each scale using a transformer-based approach. Then, these feature maps are combined using a self-attention mechanism generating a global feature. The main contribution of this work is a novel global feature generation method, which outperforms current state-of-the-art techniques that use either global-only or a combination of global and local features. This result is assessed using the Google Landmarks v2 resulting in an improvement of 3.8% in mAP@100. Also, we assess our system using Revisiting Oxford and Paris datasets, obtaining an improvement up to 3.7% in mAP compared with current methods.
更多查看译文
关键词
feature combination,multi-scale,transformer-based
AI 理解论文
溯源树
样例
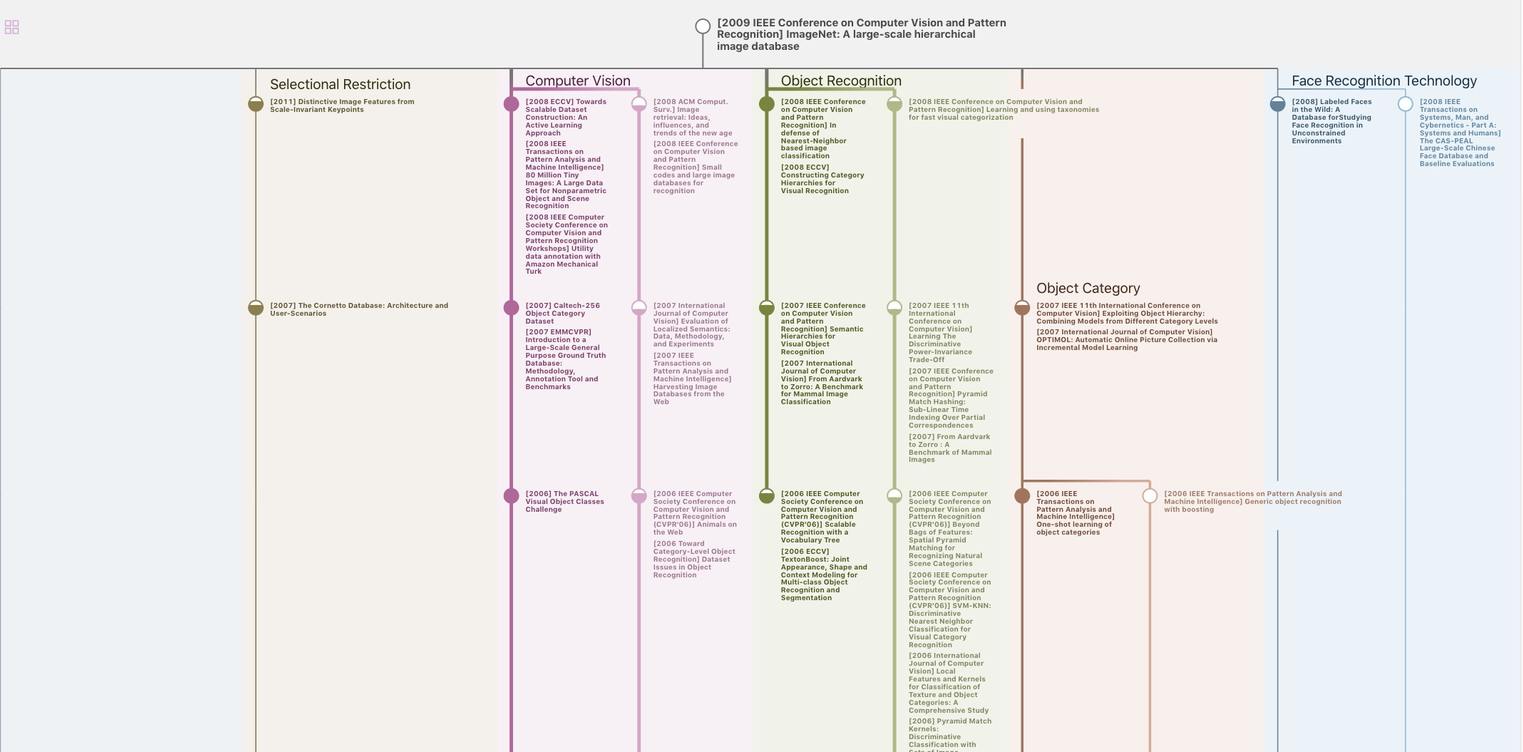
生成溯源树,研究论文发展脉络
Chat Paper
正在生成论文摘要