Exploring Active Learning for Semiconductor Defect Segmentation.
ICIP(2022)
摘要
The development of X-Ray microscopy (XRM) technology has enabled non-destructive inspection of semiconductor structures for defect identification. Deep learning is widely used as the state-of-the-art approach to perform visual analysis tasks. However, deep learning based models require large amount of annotated data to train. This can be time-consuming and expensive to obtain especially for dense prediction tasks like semantic segmentation. In this work, we explore active learning (AL) as a potential solution to alleviate the annotation burden. We identify two unique challenges when applying AL on semiconductor XRM scans: large domain shift and severe class-imbalance. To address these challenges, we propose to perform contrastive pretraining on the unlabelled data to obtain the initialization weights for each AL cycle, and a rareness-aware acquisition function that favors the selection of samples containing rare classes. We evaluate our method on a semiconductor dataset that is compiled from XRM scans of high bandwidth memory structures composed of logic and memory dies, and demonstrate that our method achieves state-of-the-art performance.
更多查看译文
关键词
active learning,semiconductor
AI 理解论文
溯源树
样例
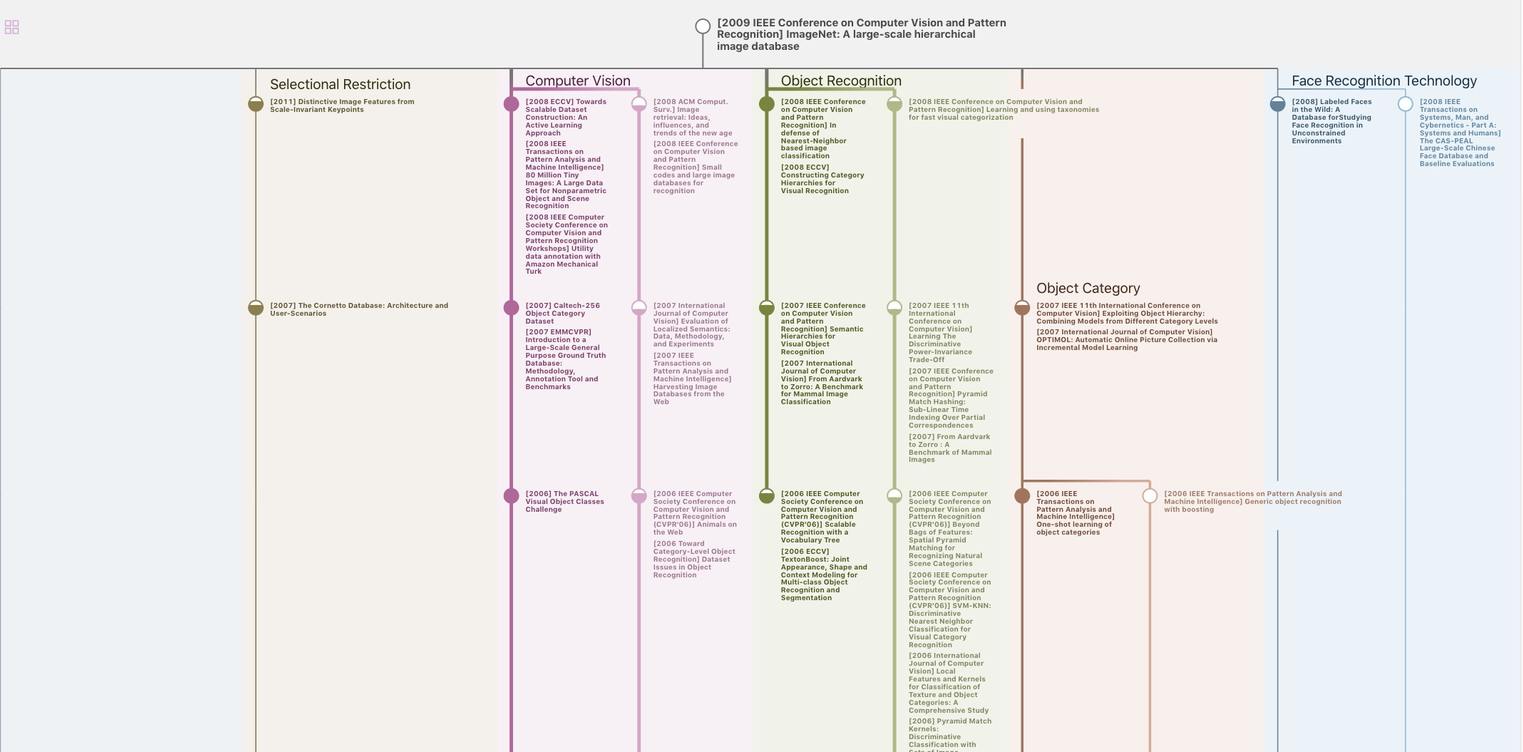
生成溯源树,研究论文发展脉络
Chat Paper
正在生成论文摘要