Occlusion-Invariant Representation Alignment for Entity Re-Identification.
ICIP(2022)
摘要
Entity re-identification is the foundation of tracking- and matching-based computer vision tasks, which are widely employed in a variety of applications. However, when trained exclusively on clear images, the models capacity to generalize is significantly affected by the presence of occlusion at referencing time, whereas data argumentation-based approaches are costly to construct without guaranteeing a test-time improvement. To tackle this problem, we propose a domain adaptation framework based on learning representations that generates occlusion-invariant feature representations by aligning the clean image embedding distribution with the occluded one, using a disparity discrepancy metric derived from the siamese network architecture. Without the need for additional processing modules during the inference stage or an expensive occlusion-augmentation-enlarged dataset during the training stage, we could obtain occlusion invariant embeddings that are free of the impact of occluders. Extensive experimental results for two tasks across three datasets indicate the proposed method's robustness and effectiveness to a variety of occlusions at all levels.
更多查看译文
关键词
Siamese network, Domain adaptation, Re-identification, Occlusion invariant
AI 理解论文
溯源树
样例
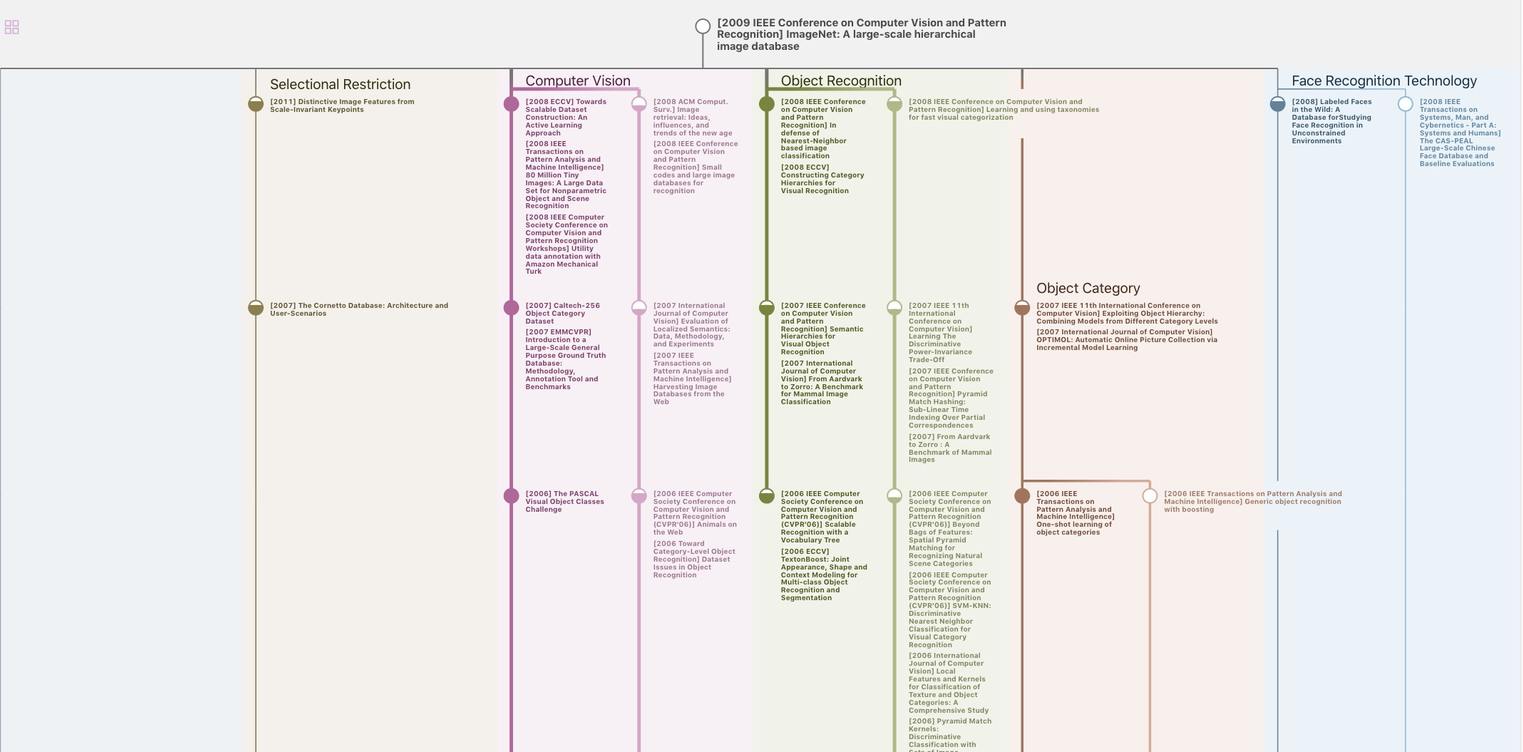
生成溯源树,研究论文发展脉络
Chat Paper
正在生成论文摘要