Gradient Perturbation-based Efficient Deep Ensembles.
COMAD/CODS(2023)
摘要
Modern deep neural networks achieve excellent predictive performance due to their massive scale, flexible architecture design and availability of large training datasets. However, several applications additionally demand reliable estimates of model and predictive uncertainty that help in making robust predictions with limited training data, enabling out-of-distribution generalization, etc. Neural networks do not offer such uncertainly estimates out-of-the-box. Although Bayesian approaches to deep learning do provide a natural way to quantify model and predictive uncertainty by inferring the posterior distribution of the model weights and averaging the model’s predictions over the entire posterior distribution, standard Bayesian inference methods such as MCMC and variational inference are difficult to design and scale to massive networks. An appealing and popular alternative is to learn an ensemble of model weights (popularly known as deep ensembles) and averaging the model’s predictions over the ensemble. However, due to the need for multiple training runs, this approach also tends to be computationally expensive. In this work, we present PEG (Perturbed Ensemble via Gradient updates), a simple and efficient approach to constructing deep ensembles using gradients computed over validation data. Experiments show that PEG can not only create an ensemble provided a pre-trained model, but it can also further enrich pre-trained models that are deep ensembles themselves. On several benchmark datasets and architectures, PEG was found to perform favorably in comparison to state-of-the-art baselines in terms of predictive performance as well as other uncertainty quantification metrics.
更多查看译文
AI 理解论文
溯源树
样例
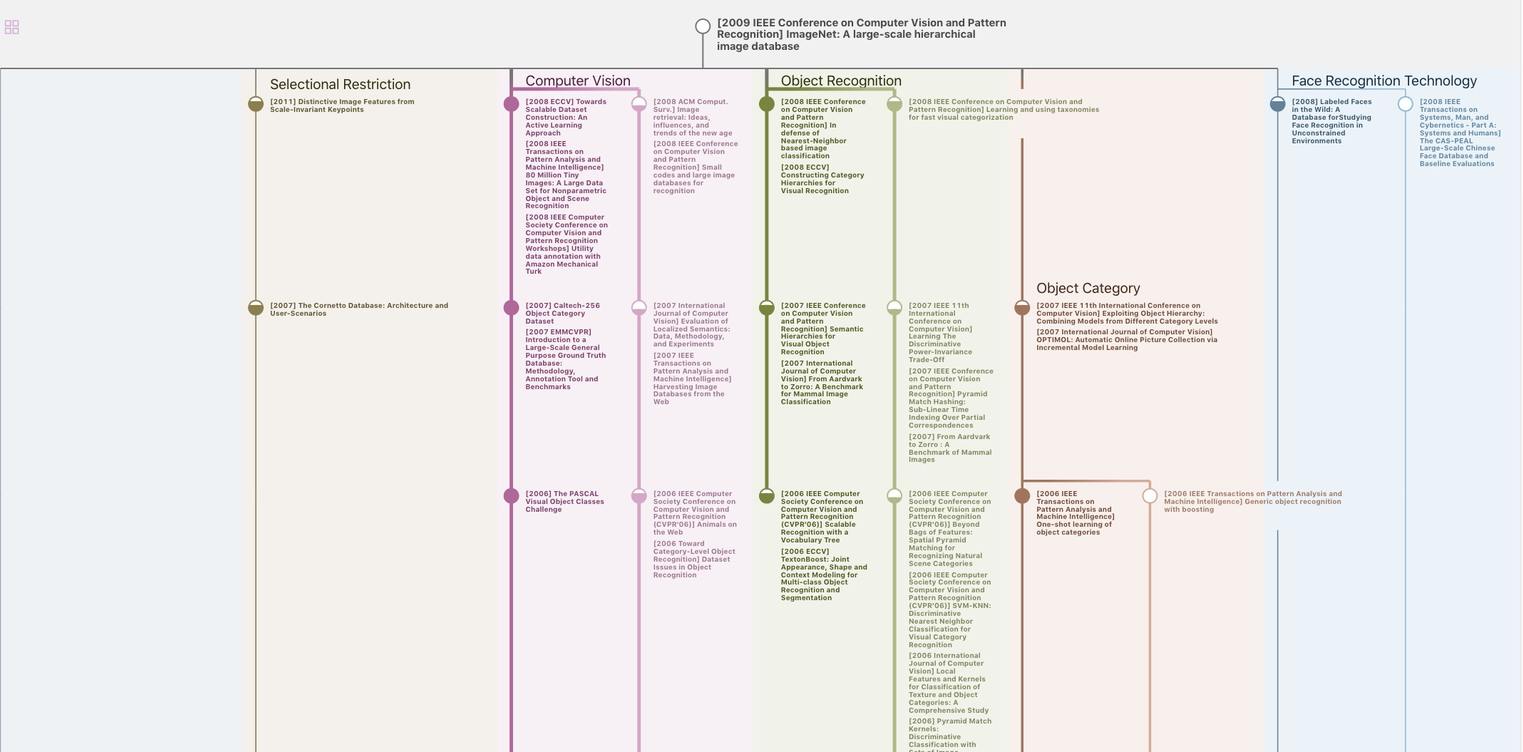
生成溯源树,研究论文发展脉络
Chat Paper
正在生成论文摘要