BRR-QA: Boosting Ranking and Reading in Open-Domain Question Answering.
COMAD/CODS(2023)
摘要
Open-domain question qnswering (OpenQA) involves a retriever for selecting relevant passages from large text corpora (e.g. Wikipedia) and a reading comprehension (RC) model for extracting answers from these retrieved passages. The retrieved passages are often noisy. Since OpenQA relies heavily on efficient passages for better answer prediction, many passage ranker models have been proposed to filter out noisy passages. However, their performance is limited because their ranker model scores each passage separately by modelling only the relationship between query and passage. Thus, they could not capture local context information. Their ranker model also ignored the rich initial rank of passages ranked by a search engine. This paper presents a Passage Ranker model that captures local-context information through cross-passage interaction. Our ranker model integrates initial ranking and uses modified attention in the cross-passage interaction to compute a better confidence score for each passage. Moreover, we integrate SRL into our passage reader and train it on proposed sampled data. Our semantic reader can absorb contextual semantics. Experimental results on four public OpenQA datasets show that our model significantly outperforms recent OpenQA baselines.
更多查看译文
AI 理解论文
溯源树
样例
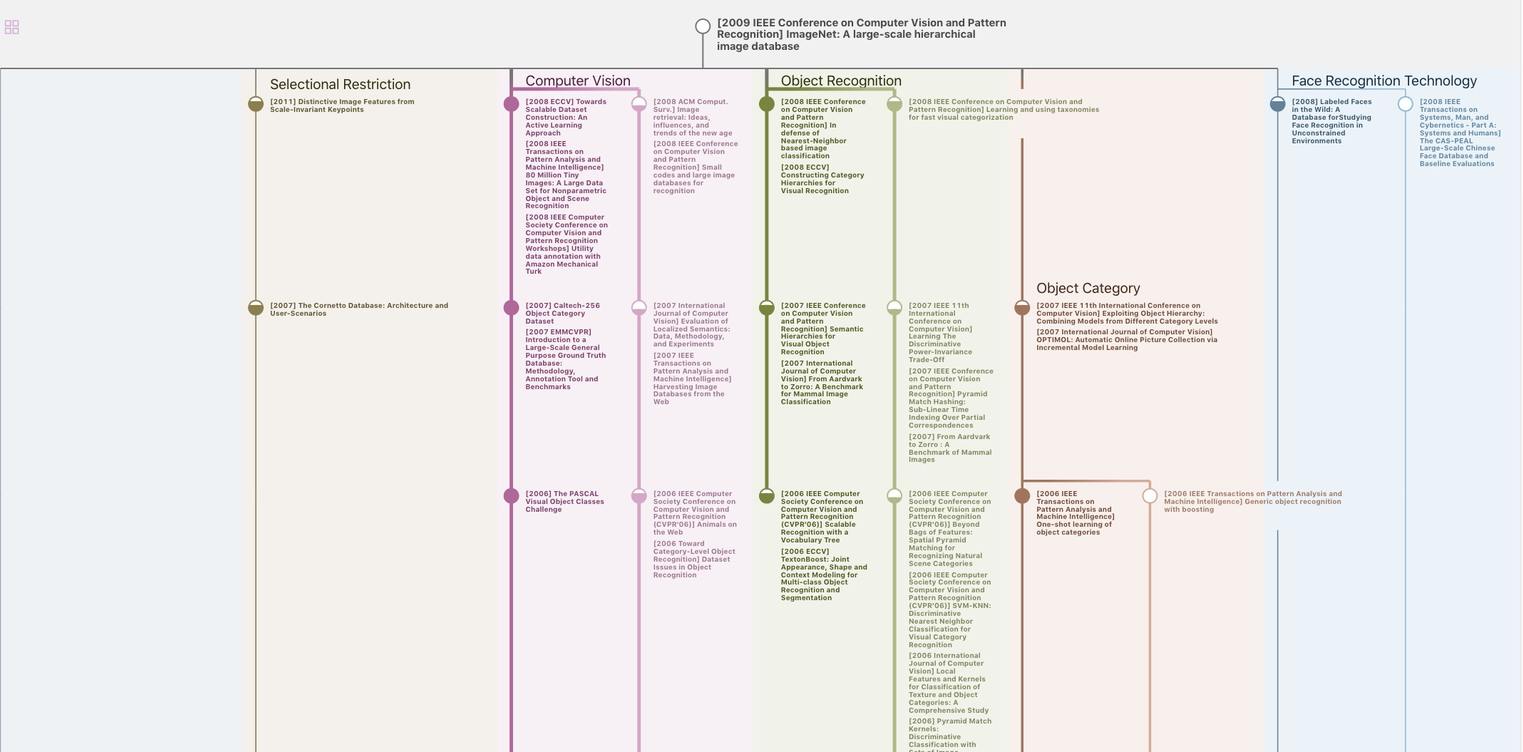
生成溯源树,研究论文发展脉络
Chat Paper
正在生成论文摘要