A Dynamic Attention Based Graph Neural Network for Anomaly Prediction in Multi-Variate Time-Series & Its Application in Network Monitoring.
COMAD/CODS(2023)
摘要
Prediction of anomaly in time-varying datasets is at the core of many real-world applications. For example, prediction of anomalous events in different time-varying business metrics and proactively mitigating any underlying problem in the system is of utmost importance to any online business to evade any kind of disruption in smooth customer experience. A large body of literature exists which adopted different techniques for anomaly prediction in multi-variate time-series data. Recent advancements in deep-learning have resulted in multiple literature proposing different variants of graph neural network (GNN) for anomaly prediction in multi-variate time-series data. However, one major gap area that remained in this domain is the sensitivity of such networks to inherent noise in the data. This is where we make our contribution by proposing a graph neural network with dynamic attention which not only offers more robustness of the network to noise but also makes the network more trainable and accurate in prediction in the presence of noise. We carried out extensive experimentation against both publicly available datasets and real-world industrial datasets. The outcome of the experimentation is consistently in support of the superiority of our proposed network over the state-of-the-art GNN network for anomaly prediction.
更多查看译文
AI 理解论文
溯源树
样例
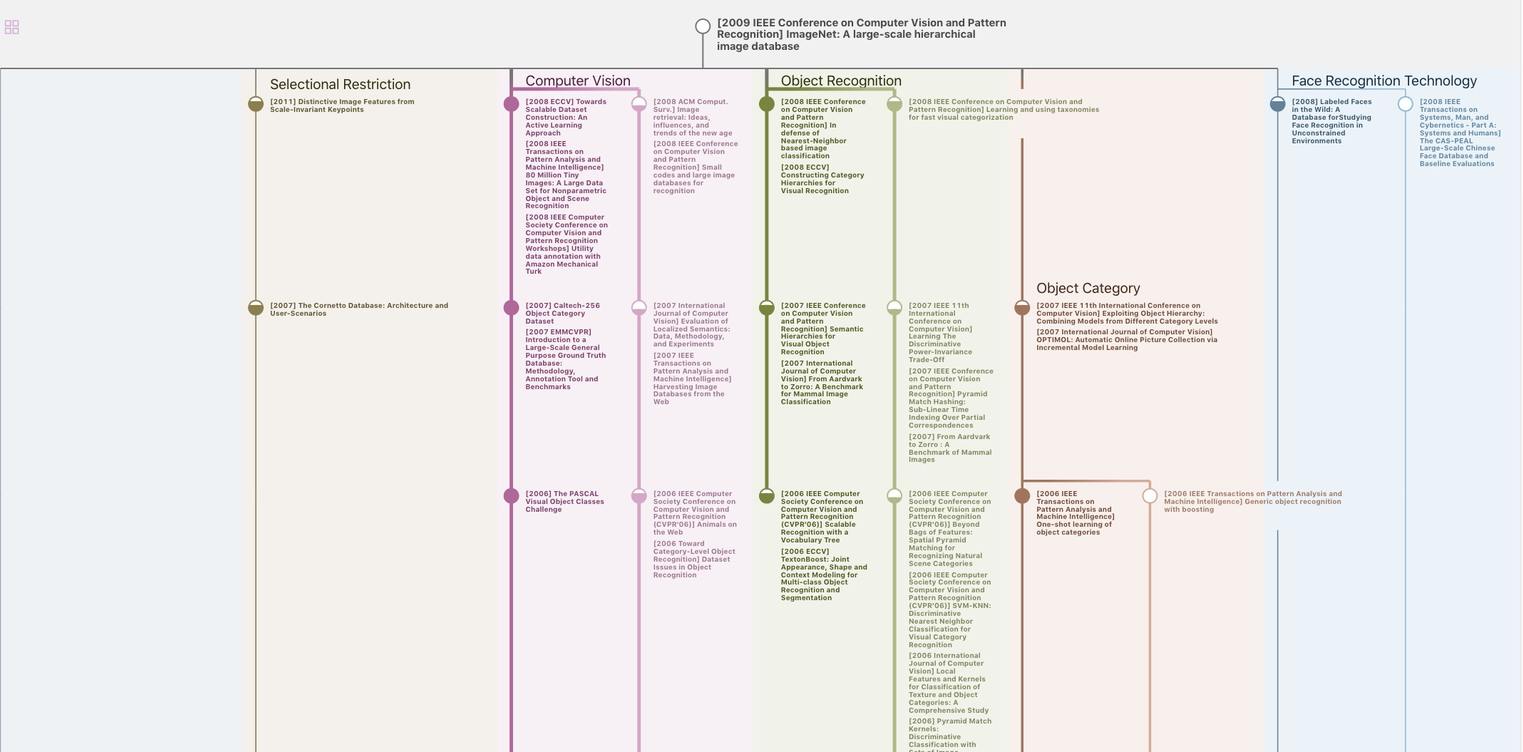
生成溯源树,研究论文发展脉络
Chat Paper
正在生成论文摘要