Effective Blockchain-Based Asynchronous Federated Learning for Edge-Computing.
CollaborateCom (1)(2022)
摘要
Since massive data are generated at the network's edge, the Internet of Things devices can exploit edge computing and federated learning to train artificial intelligence (AI) models while protecting data privacy. However, heterogeneous devices lead to low efficiency and single-point-of-failure. Moreover, malicious nodes may affect training accuracy. Therefore, we propose FedLyra, an effective blockchain-based asynchronous federated learning architecture, to improve the efficiency of aggregation and resist malicious nodes in a trusted and decentralized manner. We then propose a reputation mechanism that combines historical behaviors and the quality of local updates to resist disagreements and adversaries. With the help of the reputation mechanism, we propose a council-based decentralized aggregation mechanism to exclude malicious nodes. Experiments show that FedLyra can resist malicious nodes and ensure the accuracy of training results.
更多查看译文
关键词
Federated learning, Blockchain, Edge-computing, Asynchronous architecture, Decentralization
AI 理解论文
溯源树
样例
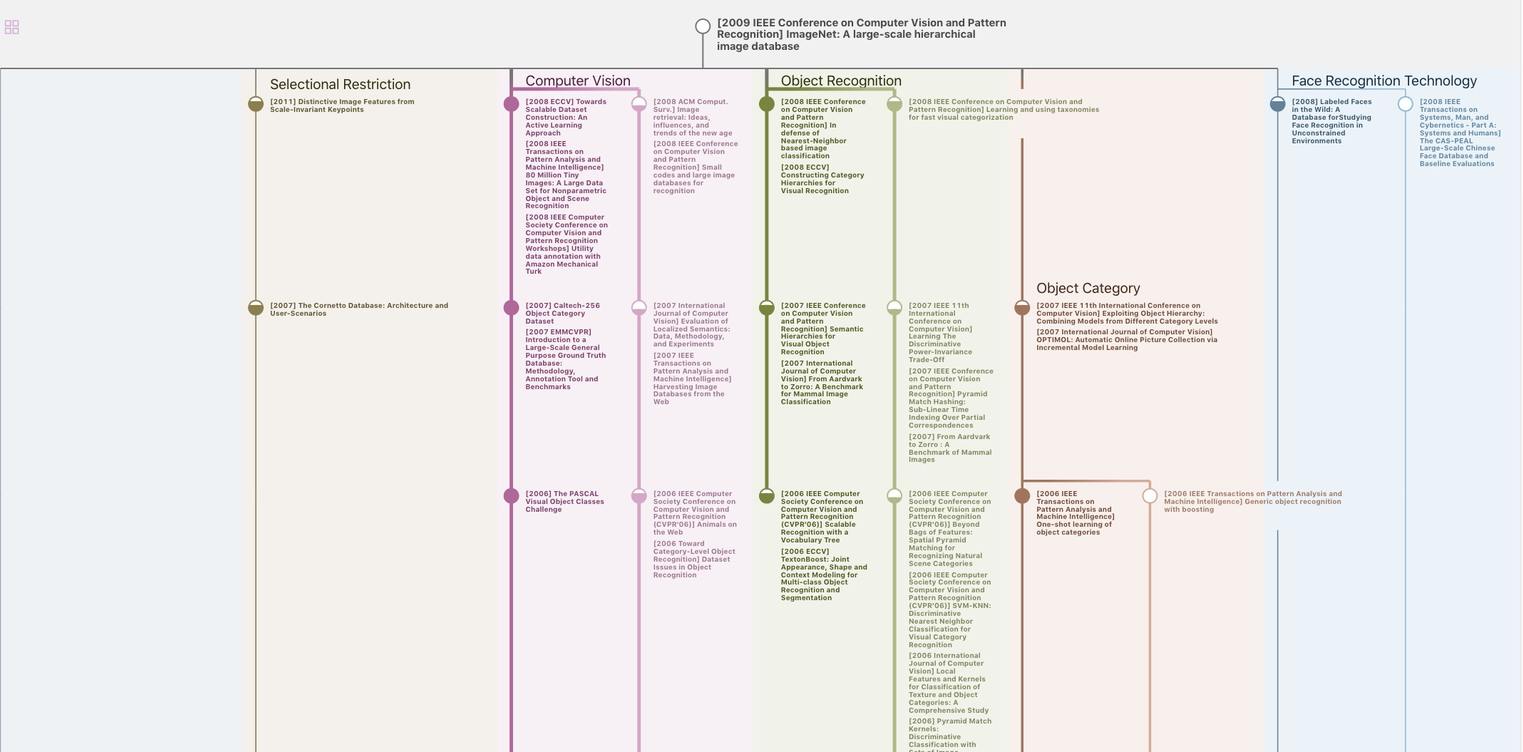
生成溯源树,研究论文发展脉络
Chat Paper
正在生成论文摘要