ITAR: A Method for Indoor RFID Trajectory Automatic Recovery.
CollaborateCom (2)(2022)
Abstract
With the increasing popularity of Radio Frequency Identification (RFID) technology, indoor applications based on RFID trajectory data analysis are becoming more and more extensive, such as personnel location, tracking, and heat map analysis. The effectiveness of indoor applications relies greatly on high-quality trajectory data. However, due to the constraints of the device and environment, RFID readers will miss reading data in real-world practice, which leads to a large number of indoor trajectories that are incomplete. To enhance trajectory data and support indoor applications more efficiently, many trajectory recovery methods to infer trajectories in free space have been proposed. However, existing methods cannot achieve automated inference and have low accuracy in inferring indoor trajectories. In this paper, we propose an Indoor Trajectory Automatic Recovery framework, ITAR, to recover missing points in indoor trajectories. ITAR adopts a sequence-to-sequence learning architecture to generate complete trajectories. We first construct a directed graph for each trajectory and use a graph neural network to capture complex location transition patterns. Then, we propose a multi-head attention mechanism to capture long-term correlations among trajectory points to improve performance. We conduct extensive experiments on synthetic and real datasets, and the results show that ITAR is superior in performance and robustness.
MoreTranslated text
Key words
Trajectory recovery, Sequence-to-sequence model, Radio frequency identification, Graph neural network
AI Read Science
Must-Reading Tree
Example
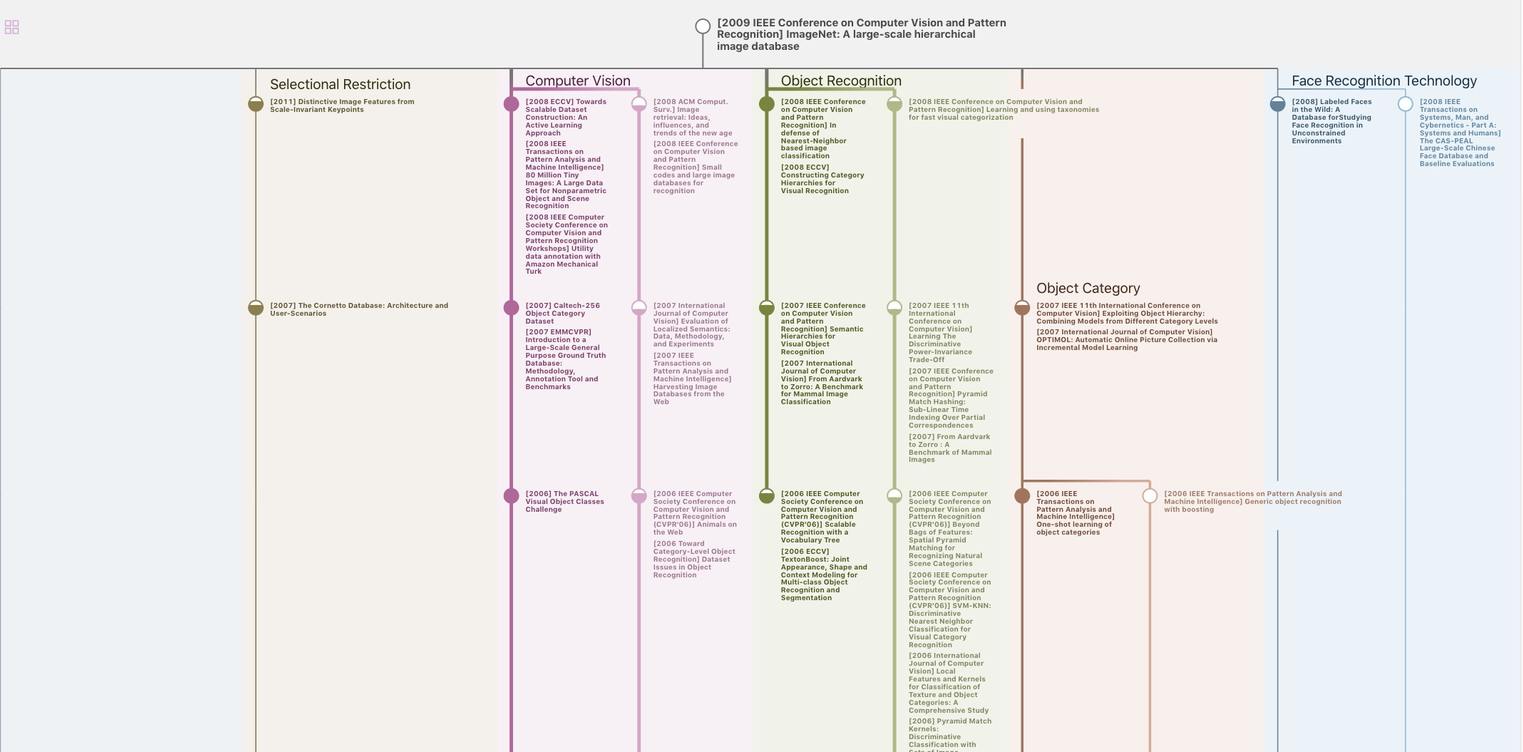
Generate MRT to find the research sequence of this paper
Chat Paper
Summary is being generated by the instructions you defined