Edge Federated Learning for Social Profit Optimality: A Cooperative Game Approach.
CollaborateCom (1)(2022)
摘要
As an emerging machine learning paradigm, federated learning satisfies people's privacy protection for private data properties to a certain extent. Especially in the field of Internet of Things (IoT), edge federated learning (EFL) allows edge devices to save private data locally when collaborative training. Most studies regard edge devices as rational and independent individuals, and use non-cooperative game methods to motivate devices to participate in training and maximize individual interests. But few studies have considered the fact that devices belonging to government or social construction agencies are more concerned with overall benefits than individual ones. In this paper, we propose an incentive mechanism for edge cooperative federated learning: ECFL. From the perspective of overall benefit, ECFL will fairly identify the contribution of edge devices and ensure the overall social benefit of the cooperative system. First, we propose a method of Shapley Value contribution degree estimated by Integrated Gradients based on the method of cooperative game (IG-Shapley Value). This method can quantitatively evaluate the contribution that edge devices provide to the model at each round of training in a fine-grained manner. At the same time, based on IG-Shapley Value, we design a collaborative contribution-aware aggregation algorithm IG-Fedavg. In order to maximize the overall social benefit, we consider the communication, storage and computing overhead of edge devices, and make joint optimization with reference to the contribution of edge devices. Extensive experimental results show that our proposed method can still make the model converge faster and achieve better performance when the overall profit is improved by more than 30%.
更多查看译文
关键词
Federated learning, Cooperative game, Internet of Things, Contribution evaluation
AI 理解论文
溯源树
样例
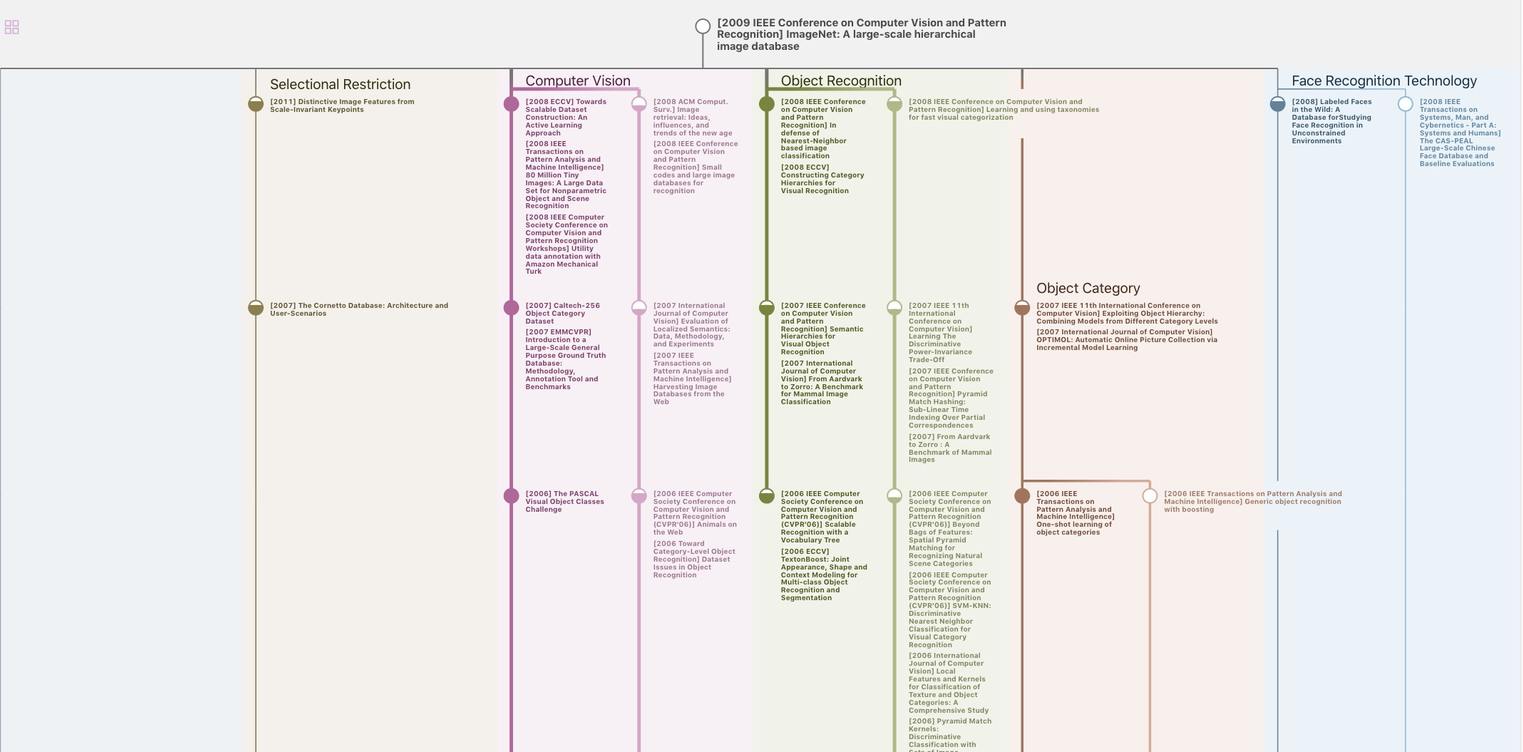
生成溯源树,研究论文发展脉络
Chat Paper
正在生成论文摘要