Towards Structured Noise Models for Unsupervised Denoising.
ECCV Workshops (4)(2022)
摘要
The introduction of unsupervised methods in denoising has shown that unpaired noisy data can be used to train denoising networks, which can not only produce high quality results but also enable us to sample multiple possible diverse denoising solutions. However, these systems rely on a probabilistic description of the imaging noise–a noise model. Until now, imaging noise has been modelled as pixel-independent in this context. While such models often capture shot noise and readout noise very well, they are unable to describe many of the complex patterns that occur in real life applications. Here, we introduce a novel learning-based autoregressive noise model to describe imaging noise and show how it can enable unsupervised denoising for settings with complex structured noise patterns. We show that our deep autoregressive noise models have the potential to greatly improve denoising quality in structured noise datasets. We showcase the capability of our approach on various simulated datasets and on real photo-acoustic imaging data.
更多查看译文
关键词
structured noise models
AI 理解论文
溯源树
样例
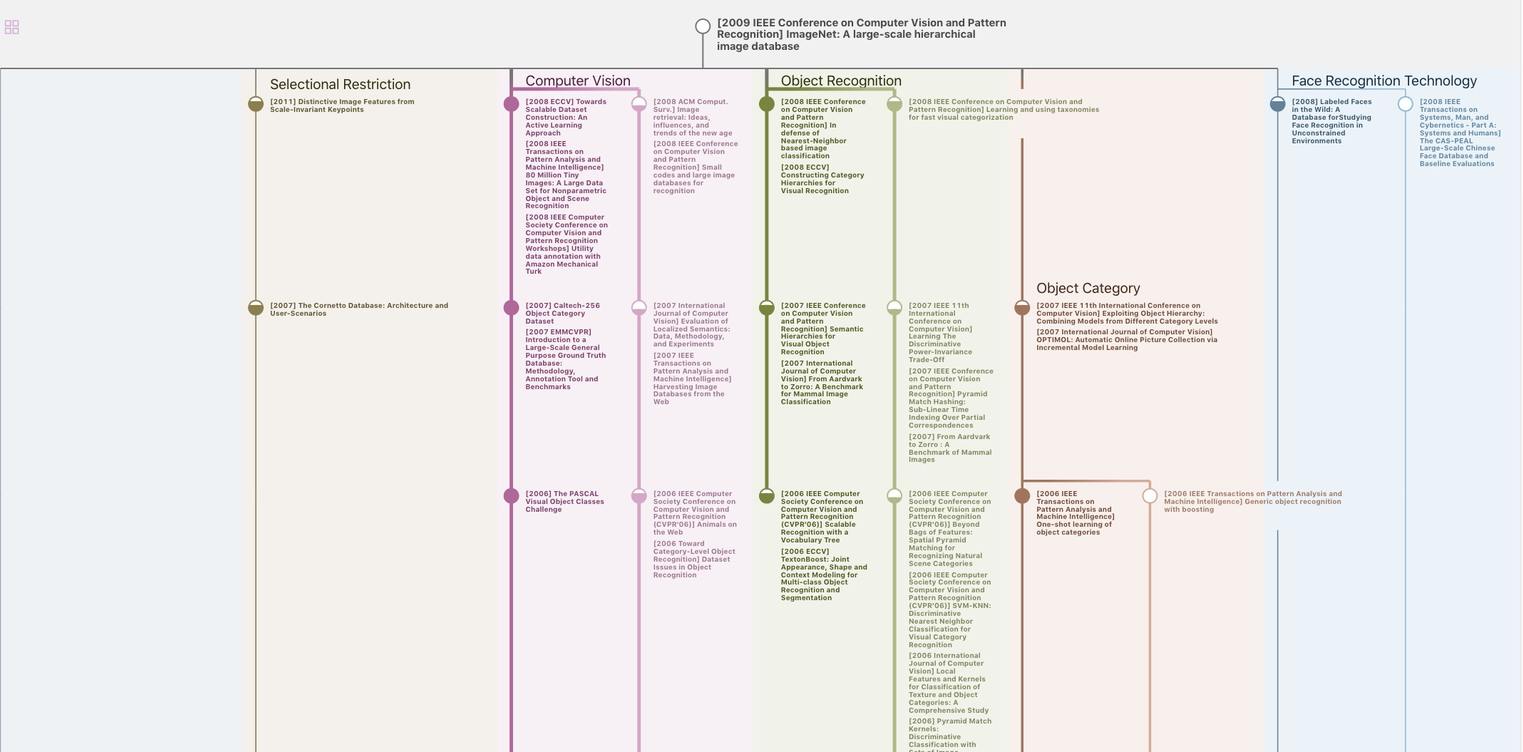
生成溯源树,研究论文发展脉络
Chat Paper
正在生成论文摘要