Discriminative Attribution from Paired Images.
ECCV Workshops (4)(2022)
摘要
We present a method for deep neural network interpretability by combining feature attribution with counterfactual explanations to generate attribution maps that highlight the most discriminative features between classes. Crucially, this method can be used to quantitatively evaluate the performance of feature attribution methods in an objective manner, thus preventing potential observer bias. We evaluate the proposed method on six diverse datasets, and use it to discover so far unknown morphological features of synapses in Drosophila melanogaster. We show quantitatively and qualitatively that the highlighted features are substantially more discriminative than those extracted using conventional attribution methods and improve upon similar approaches for counterfactual explainability. We argue that the extracted explanations are better suited for understanding fine grained class differences as learned by a deep neural network, in particular for image domains where humans have little to no visual priors, such as biomedical datasets.
更多查看译文
关键词
discriminative attribution,images
AI 理解论文
溯源树
样例
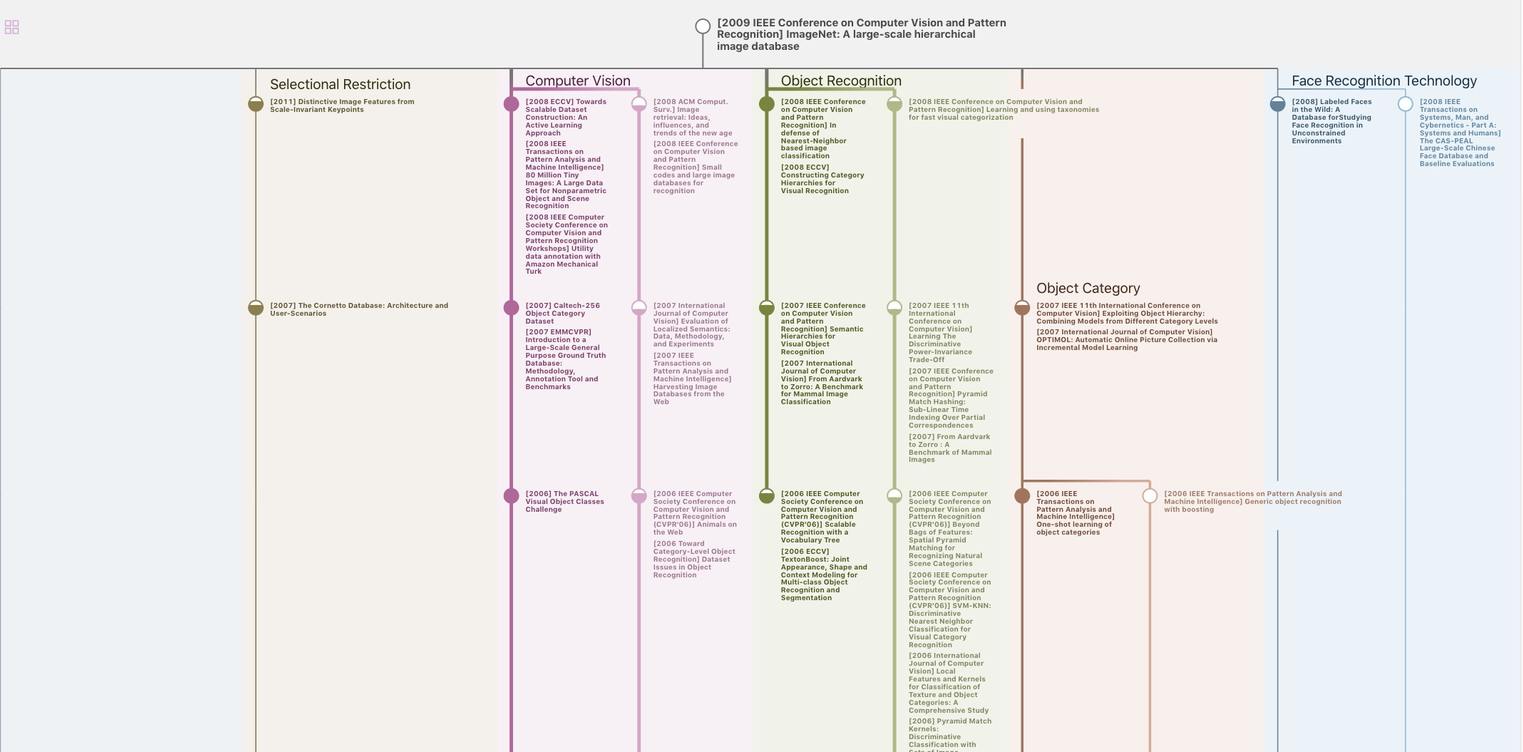
生成溯源树,研究论文发展脉络
Chat Paper
正在生成论文摘要