Relieving Pixel-Wise Labeling Effort for Pathology Image Segmentation with Self-training.
ECCV Workshops (7)(2022)
摘要
Data scarcity is a common issue when training deep learning models for digital pathology, as large exhaustively-annotated image datasets are difficult to obtain. In this paper, we propose a self-training based approach that can exploit both (few) exhaustively annotated images and (very) sparsely-annotated images to improve the training of deep learning models for image segmentation tasks. The approach is evaluated on three public and one in-house dataset, representing a diverse set of segmentation tasks in digital pathology. The experimental results show that self-training allows to bring significant model improvement by incorporating sparsely annotated images and proves to be a good strategy to relieve labeling effort in the digital pathology domain.
更多查看译文
关键词
Deep learning, Image segmentation, Self-training, Data scarcity, Digital pathology
AI 理解论文
溯源树
样例
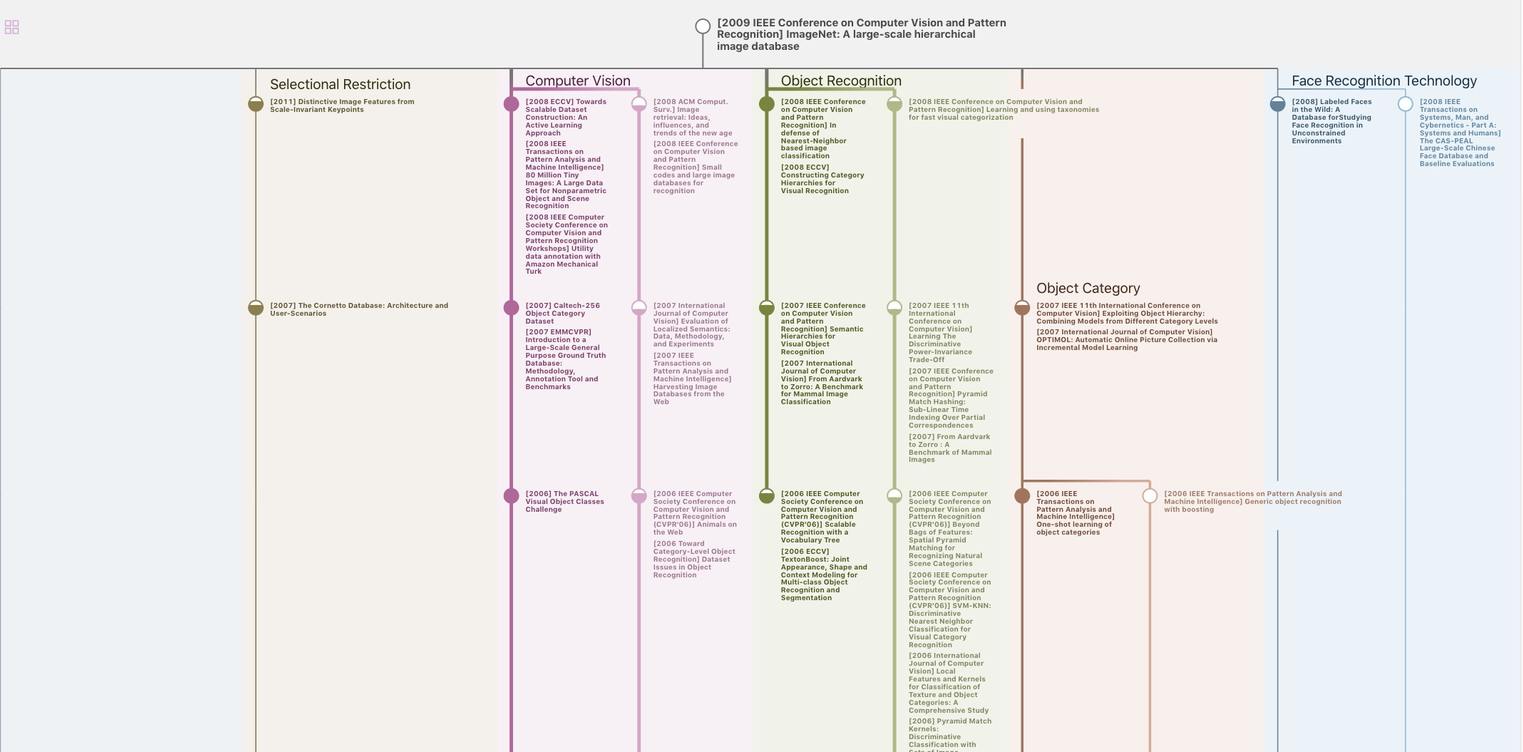
生成溯源树,研究论文发展脉络
Chat Paper
正在生成论文摘要