Deep Learning-Based Assessment of Facial Periodic Affect in Work-Like Settings.
ECCV Workshops (5)(2022)
摘要
Facial behaviour forms an important cue for understanding human affect. While a large number of existing approaches successfully recognize affect from facial behaviours occurring in daily life, facial behaviours displayed in work settings have not been investigated to a great extent. This paper presents the first study that systematically investigates the influence of spatial and temporal facial behaviours on human affect recognition in work-like settings. We first introduce a new multi-site data collection protocol for acquiring human behavioural data under various simulated working conditions. Then, we propose a deep learning-based framework that leverages both spatio-temporal facial behavioural cues and background information for workers’ affect recognition. We conduct extensive experiments to evaluate the impact of spatial, temporal and contextual information for models that learn to recognize affect in work-like settings. Our experimental results show that (i) workers’ affective states can be inferred from their facial behaviours; (ii) models pre-trained on naturalistic datasets prove useful for predicting affect from facial behaviours in work-like settings; and (iii) task type and task setting influence the affect recognition performance.
更多查看译文
关键词
facial periodic affect,learning-based,work-like
AI 理解论文
溯源树
样例
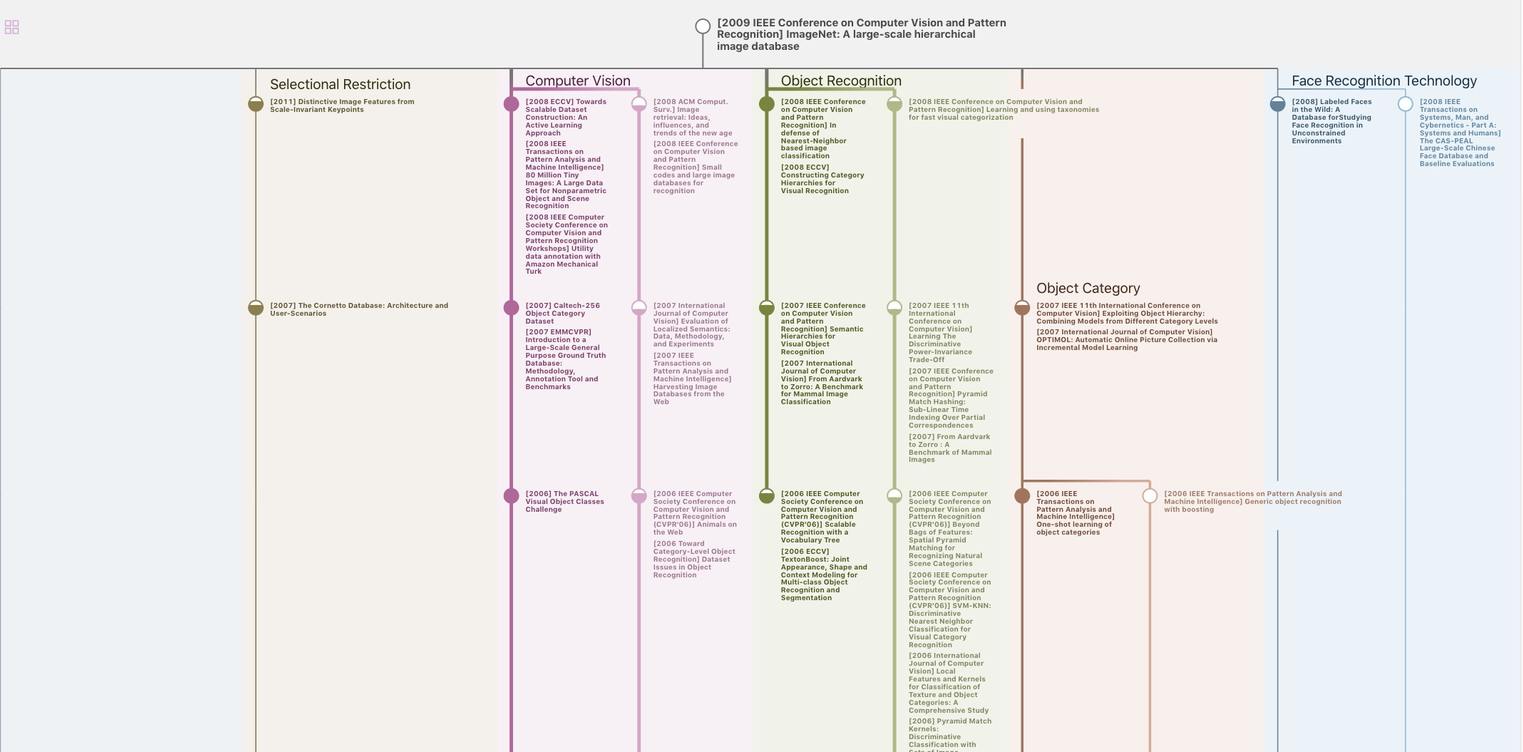
生成溯源树,研究论文发展脉络
Chat Paper
正在生成论文摘要