Towards Differentially Private Contrastive Learning.
ML4CS (2)(2022)
摘要
With the surge of unlabeled data, increasing efforts have been put into unsupervised learning. As one of the most representative branches of unsupervised learning, contrastive learning has achieved great progress by the high efficiency. Unfortunately, privacy threats on contrastive learning have become sophisticated, making it imperative to develop effective technologies that are able to deal with such threats. To alleviate the privacy issue in contrastive learning, we propose some novel techniques based on differential privacy, which aim at reducing the high sensitivity of gradient in the private training caused by interactive contrastive learning. Specifically, we add differentially private protection to the connection point related to different per-example gradients, which decreases the sensitivity of the gradients significantly. Our experiments on SimCLR demonstrate the superiority of our approach with higher accuracy under the same privacy protection.
更多查看译文
关键词
learning
AI 理解论文
溯源树
样例
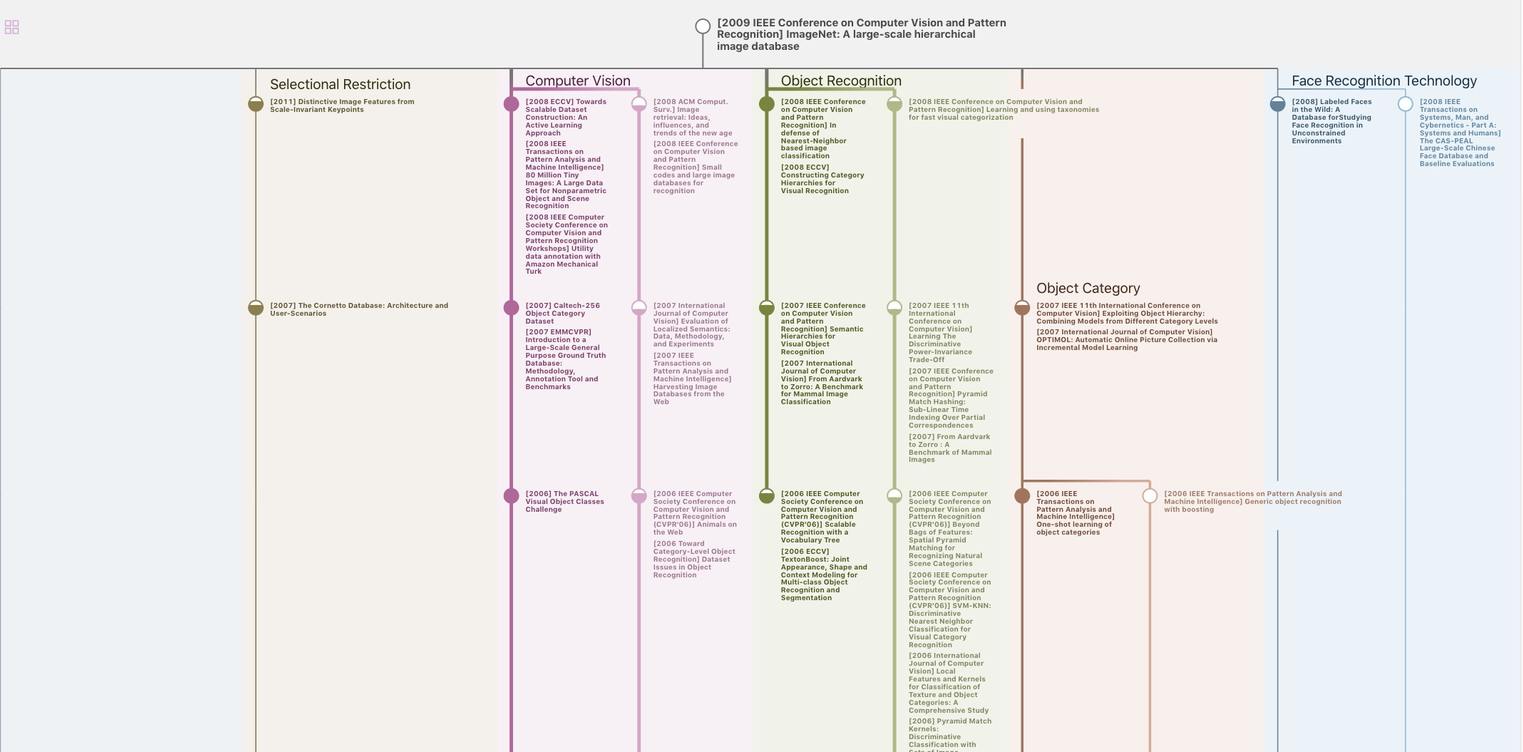
生成溯源树,研究论文发展脉络
Chat Paper
正在生成论文摘要