TSFed: A Two-Stage Federated Learning Framework via Cloud-Edge Collaboration for Services QoS Prediction.
ICWS(2022)
摘要
Federated learning-based quality of service (QoS) prediction methods are regularly used to protect user privacy in smart cities. However, federated learning (FL) is fragile for heterogeneous QoS data, and these FL methods usually update a single global model by aggregating diverging gradients, which cannot effectively capture the heterogeneous data features of different users, resulting in less than optimal model convergence speed. Moreover, the existing FL methods do not pay attention to the positive effect of regional similarity of QoS data on model convergence. To address these issues, we propose a two-stage federated learning QoS prediction framework (TSFed) based on cloud-edge collaboration. In the first stage, the cloud server coordinates the user to train a partially optimized pre-training model. In the second stage, the edge server coordinates users to fine-tune the pre-training model. Experiments on real-world datasets show that TSFed can achieve a 21.54%–46.73% reduction in the number of communication rounds and a 29.83%–50.73% reduction in communication delay required to achieve the target prediction accuracy compared to existing approaches.
更多查看译文
关键词
prediction,two-stage,cloud-edge
AI 理解论文
溯源树
样例
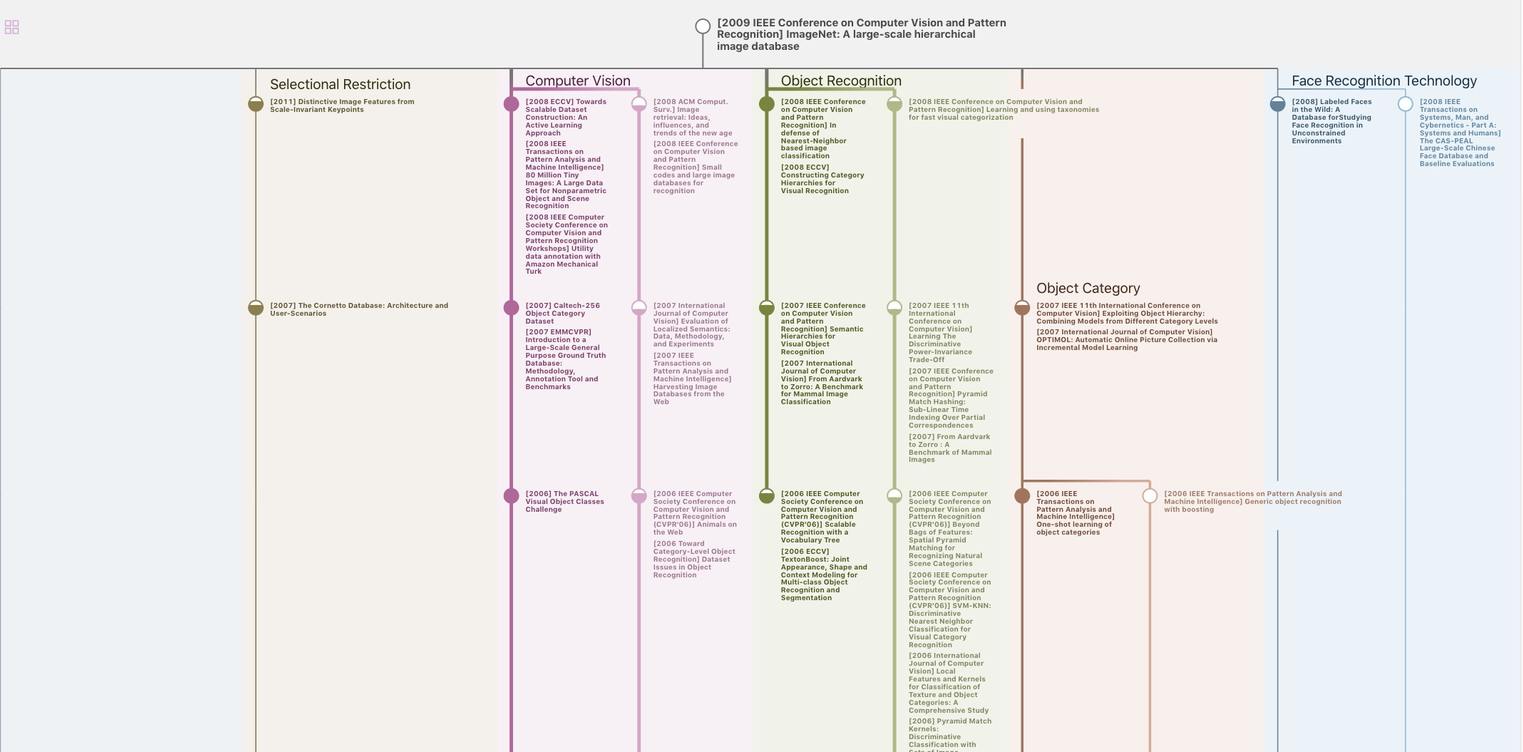
生成溯源树,研究论文发展脉络
Chat Paper
正在生成论文摘要