Healthcare Monitoring for SCI Individuals: Learning Activities of Daily Living through a SlowFast Neural Network.
SII(2023)
Abstract
Continuous healthcare monitoring is a prevalent need for the spinal cord injured (SCI) population to mitigate the risk of secondary health conditions and develop preventive methods. In this paper, we developed an embodied system for remote monitoring and classification of activities of daily living (ADL). We propose a framework for sensor transfer learning to recognize these activities from simple and short samples through label transferring from a deep SlowFast network on video data. Multiple wearable devices were included and synchronized in this study to capture physiological data as well as posture and movement from acceleration and pressure distribution. Furthermore, we propose a pipeline of data capturing for automatically creating a ground truth dataset of different ADLs. Results from a pilot training with eight users in 15 minutes task recordings over eight different tasks produced a weighted accuracy on F1-score of 77% in correctly classifying ADLs. Although extending the data is required to increase precision, we showed a successful method for monitoring and classification of specific activities in SCI with up to 91% in accuracy. Further work will focus on extending to multi-class soft labelling for achieving usability in complex situations.
MoreTranslated text
Key words
neural network,sci individuals,healthcare,monitoring,learning
AI Read Science
Must-Reading Tree
Example
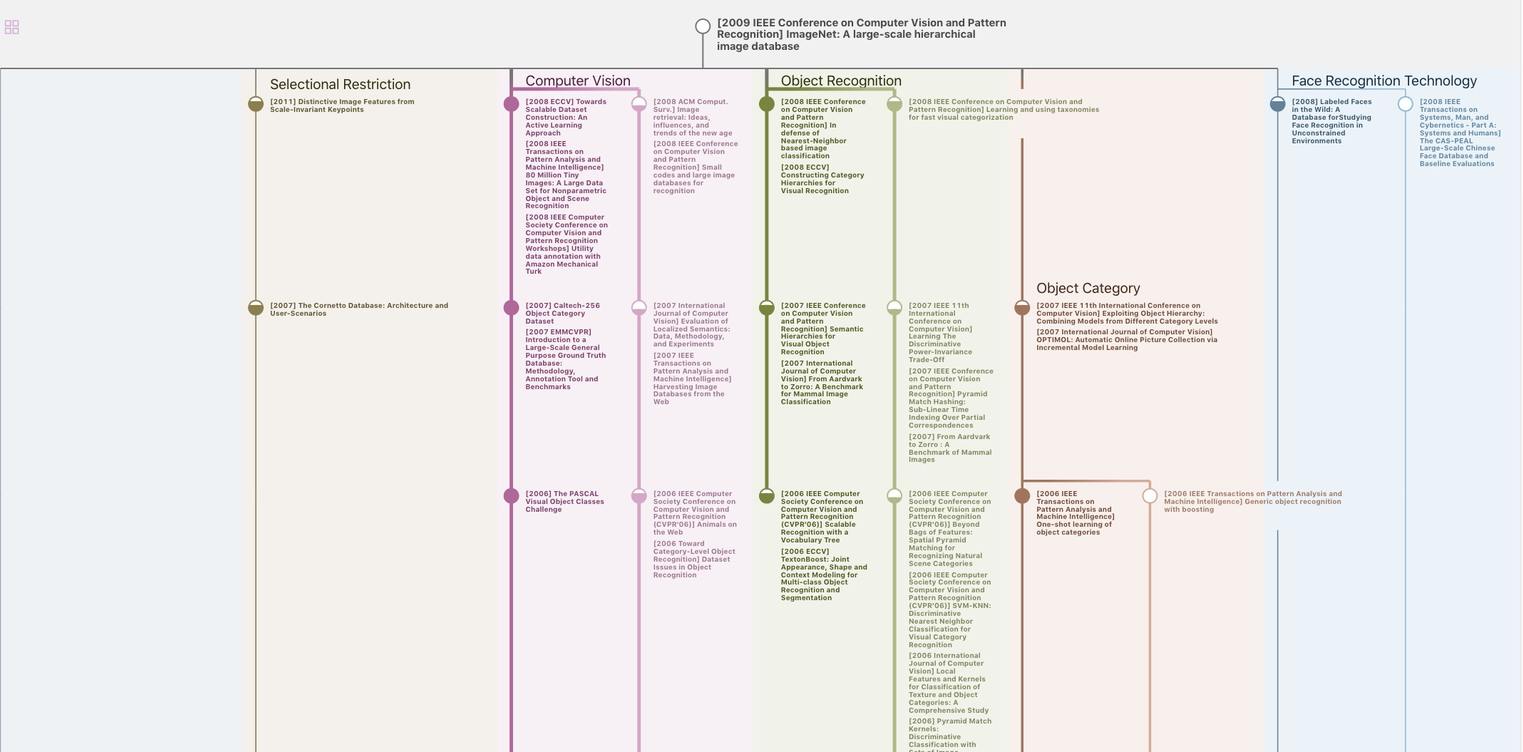
Generate MRT to find the research sequence of this paper
Chat Paper
Summary is being generated by the instructions you defined