Multivariate brain-based dimensions of child psychiatric problems: degrees of generalizability.
medRxiv : the preprint server for health sciences(2023)
摘要
Multivariate machine learning techniques are a promising set of tools for identifying complex brain-behavior associations. However, failure to replicate results from these methods across samples has hampered their clinical relevance. This study aimed to delineate dimensions of brain functional connectivity that are associated with child psychiatric symptoms in two large and independent cohorts: the Adolescent Brain Cognitive Development (ABCD) Study and the Generation R Study (total =8,605). Using sparse canonical correlations analysis, we identified three brain-behavior dimensions in ABCD: attention problems, aggression and rule-breaking behaviors, and withdrawn behaviors. Importantly, generalizability of these dimensions was consistently observed in ABCD, suggesting robust multivariate brain-behavior associations. Despite this, generalizability in Generation R was limited. These results highlight that the of generalizability can vary depending on the external validation methods employed as well as the datasets used, emphasizing that biomarkers will remain elusive until models generalize better in true external settings.
更多查看译文
关键词
psychiatric problems,dimensions,child,brain-based
AI 理解论文
溯源树
样例
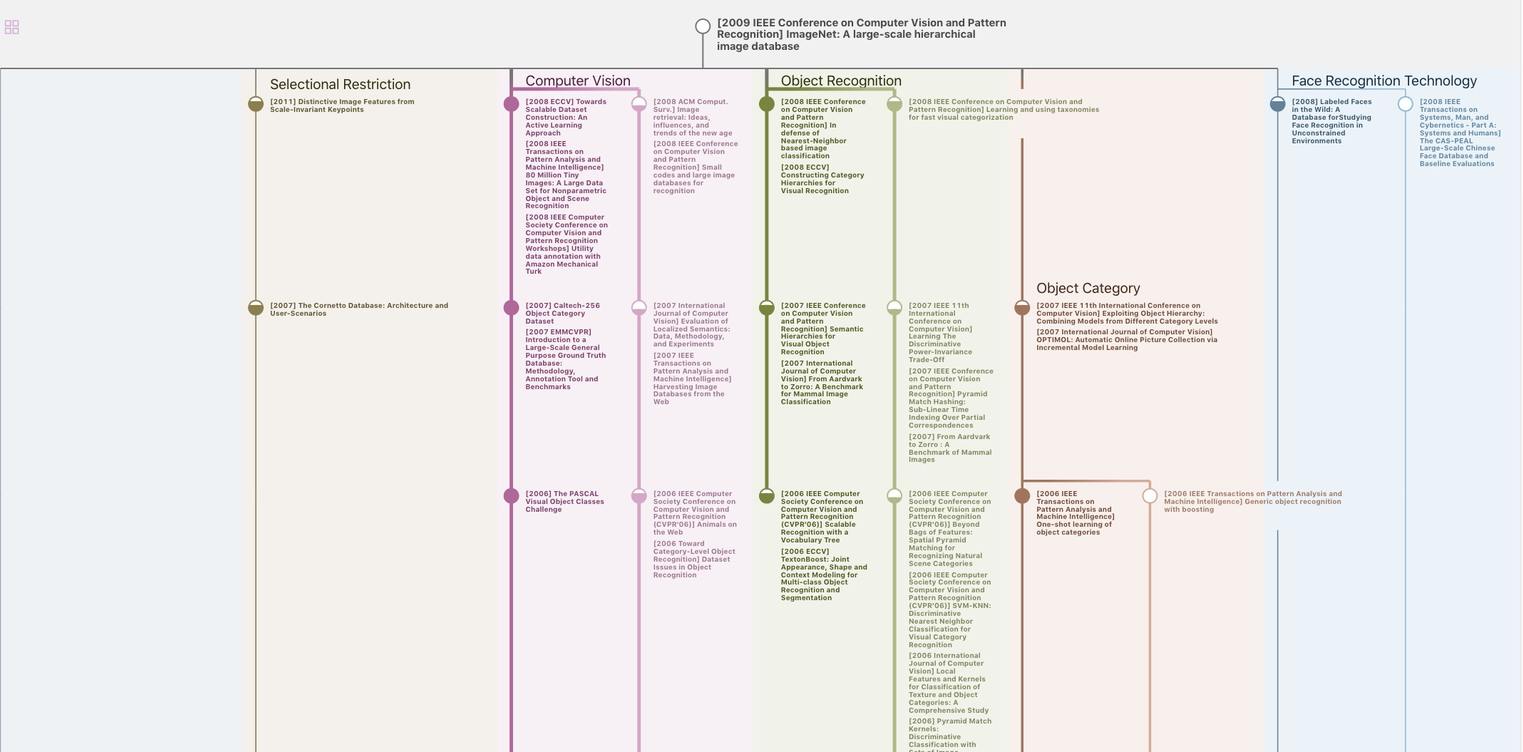
生成溯源树,研究论文发展脉络
Chat Paper
正在生成论文摘要