A Laboratory Evaluation of the New Automated Pollen Sensor Beenose: Pollen Discrimination Using Machine Learning Techniques.
Sensors (Basel, Switzerland)(2023)
摘要
The monitoring of airborne pollen has received much attention over the last decade, as the prevalence of pollen-induced allergies is constantly increasing. Today, the most common technique to identify airborne pollen species and to monitor their concentrations is based on manual analysis. Here, we present a new, low-cost, real-time optical pollen sensor, called Beenose, that automatically counts and identifies pollen grains by performing measurements at multiple scattering angles. We describe the data pre-processing steps and discuss the various statistical and machine learning methods that have been implemented to distinguish different pollen species. The analysis is based on a set of 12 pollen species, several of which were selected for their allergic potency. Our results show that Beenose can provide a consistent clustering of the pollen species based on their size properties, and that pollen particles can be separated from non-pollen ones. More importantly, 9 out of 12 pollen species were correctly identified with a prediction score exceeding 78%. Classification errors occur for species with similar optical behaviour, suggesting that other parameters should be considered to provide even more robust pollen identification.
更多查看译文
关键词
machine learning,optical sensor,pollen monitoring,real time
AI 理解论文
溯源树
样例
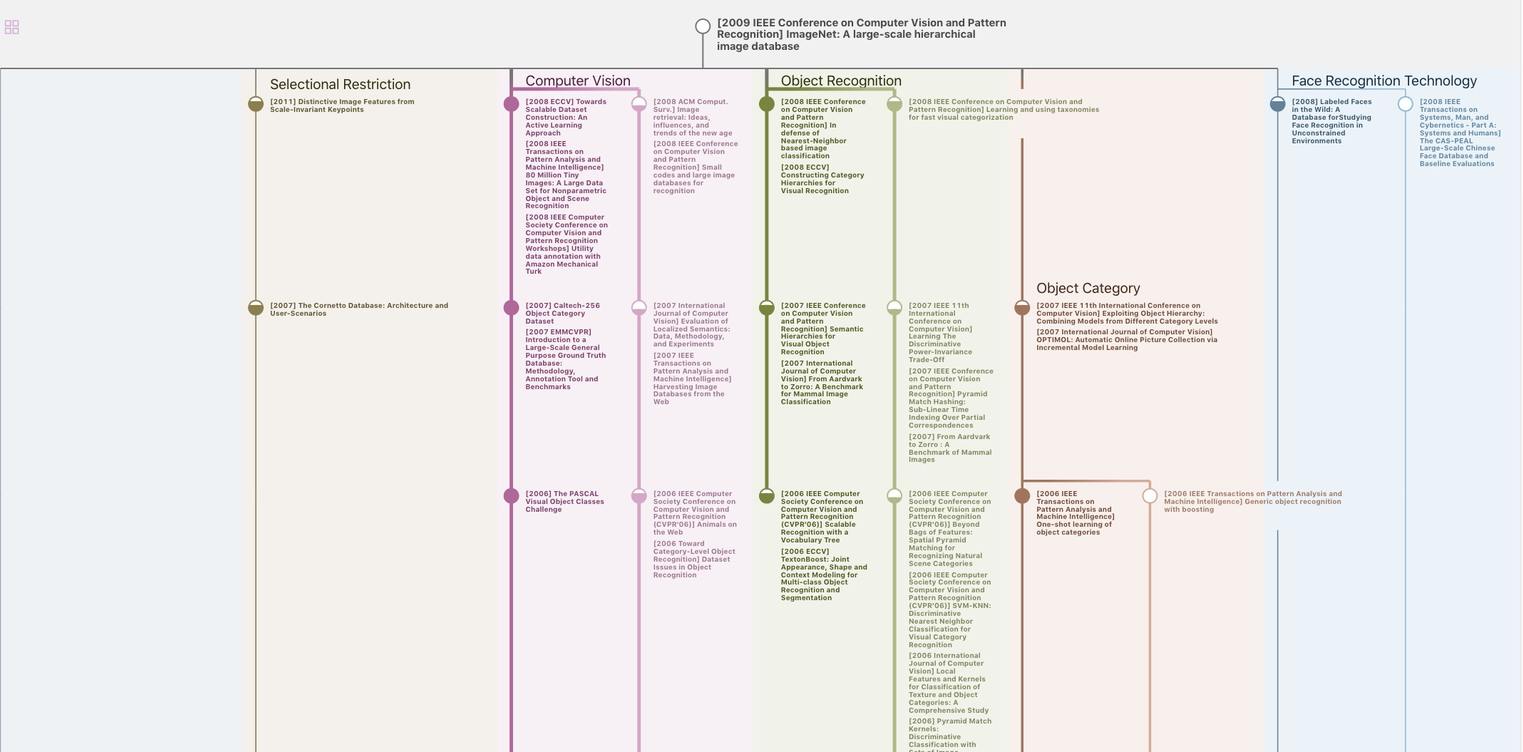
生成溯源树,研究论文发展脉络
Chat Paper
正在生成论文摘要