Dynamic Correlation Adjacency-Matrix-Based Graph Neural Networks for Traffic Flow Prediction.
Sensors (Basel, Switzerland)(2023)
摘要
Modeling complex spatial and temporal dependencies in multivariate time series data is crucial for traffic forecasting. Graph convolutional networks have proved to be effective in predicting multivariate time series. Although a predefined graph structure can help the model converge to good results quickly, it also limits the further improvement of the model due to its stationary state. In addition, current methods may not converge on some datasets due to the graph structure of these datasets being difficult to learn. Motivated by this, we propose a novel model named Dynamic Correlation Graph Convolutional Network (DCGCN) in this paper. The model can construct adjacency matrices from input data using a correlation coefficient; thus, dynamic correlation graph convolution is used for capturing spatial dependencies. Meanwhile, gated temporal convolution is used for modeling temporal dependencies. Finally, we performed extensive experiments to evaluate the performance of our proposed method against ten existing well-recognized baseline methods using two original and four public datasets.
更多查看译文
关键词
dynamic adjacency matrix,graph neural networks,multivariate time series,traffic prediction
AI 理解论文
溯源树
样例
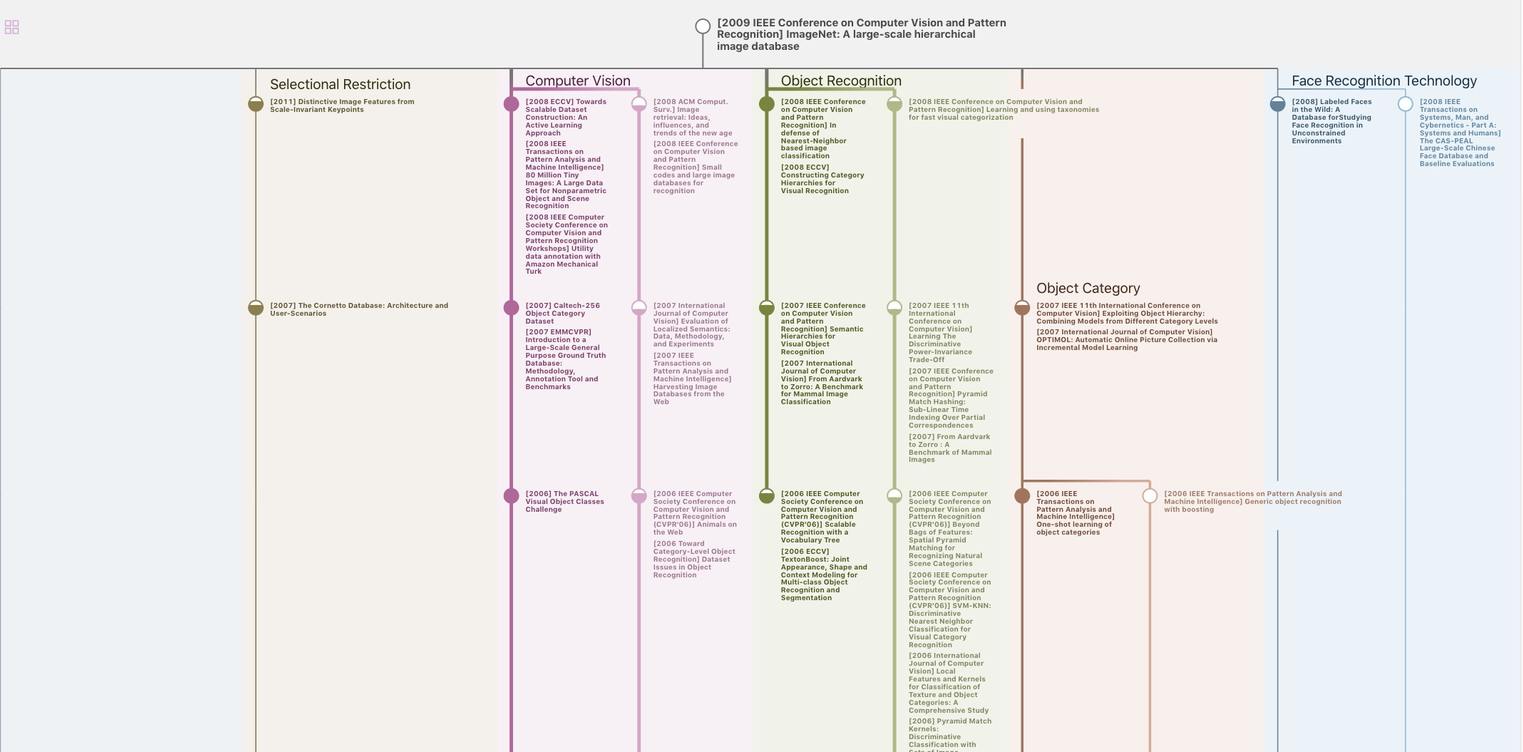
生成溯源树,研究论文发展脉络
Chat Paper
正在生成论文摘要