MAHALO: Unifying Offline Reinforcement Learning and Imitation Learning from Observations
CoRR(2023)
摘要
We study a new paradigm for sequential decision making, called offline Policy Learning from Observation (PLfO). Offline PLfO aims to learn policies using datasets with substandard qualities: 1) only a subset of trajectories is labeled with rewards, 2) labeled trajectories may not contain actions, 3) labeled trajectories may not be of high quality, and 4) the overall data may not have full coverage. Such imperfection is common in real-world learning scenarios, so offline PLfO encompasses many existing offline learning setups, including offline imitation learning (IL), ILfO, and reinforcement learning (RL). In this work, we present a generic approach, called Modality-agnostic Adversarial Hypothesis Adaptation for Learning from Observations (MAHALO), for offline PLfO. Built upon the pessimism concept in offline RL, MAHALO optimizes the policy using a performance lower bound that accounts for uncertainty due to the dataset's insufficient converge. We implement this idea by adversarially training data-consistent critic and reward functions in policy optimization, which forces the learned policy to be robust to the data deficiency. We show that MAHALO consistently outperforms or matches specialized algorithms across a variety of offline PLfO tasks in theory and experiments.
更多查看译文
关键词
unifying offline reinforcement learning,imitation learning,reinforcement learning,observations
AI 理解论文
溯源树
样例
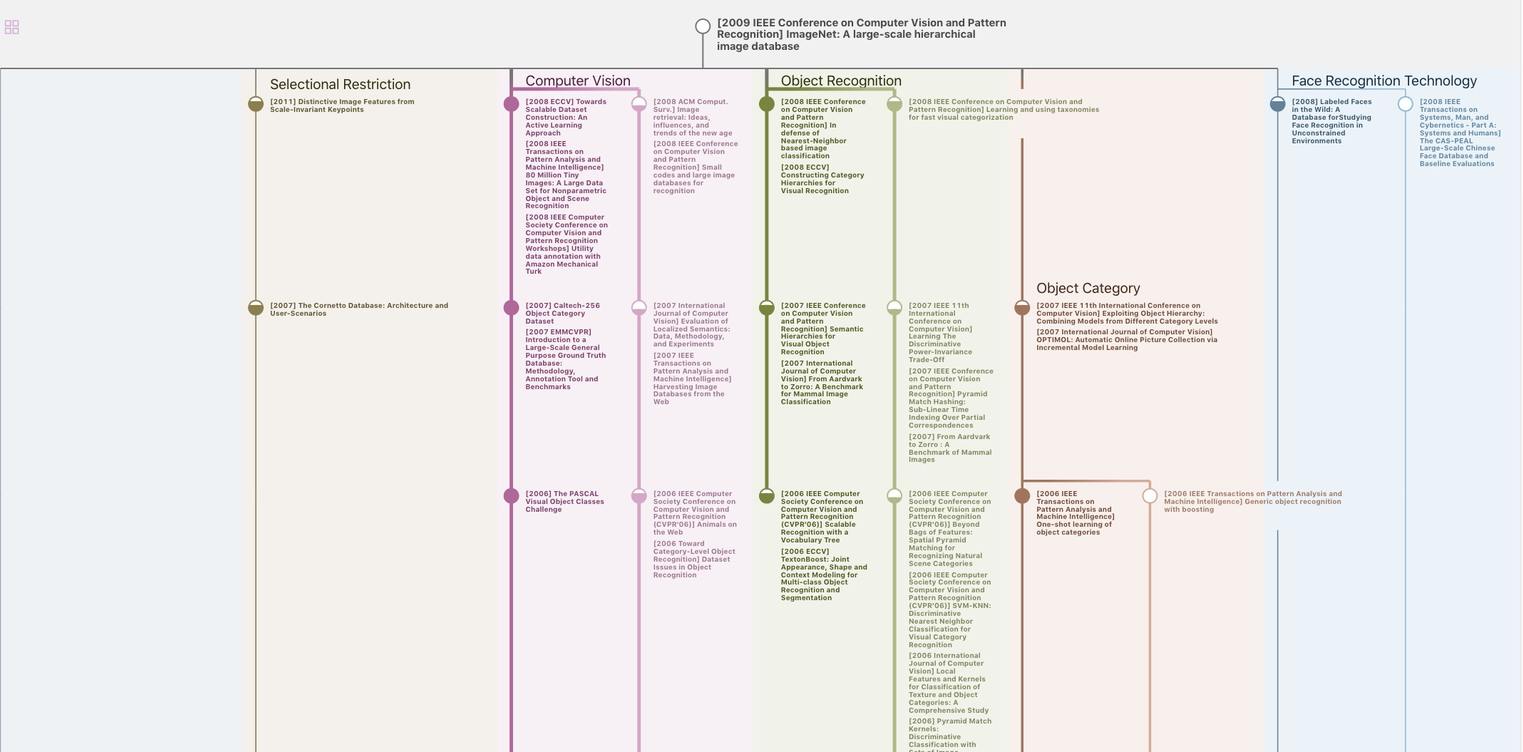
生成溯源树,研究论文发展脉络
Chat Paper
正在生成论文摘要