A multi-site tide level prediction model based on graph convolutional recurrent networks
Ocean Engineering(2023)
摘要
Predicting regional tide levels is vital for engineering and catastrophe avoidance along the shore. Data-driven method is capable of fast prediction of tide levels. However, current data-driven algorithms only make predictions for individual tide stations, rather than aiming to a regional network system of tide stations. This paper proposes a model based on graph convolutional recurrent networks to predict tidal levels at regional multiple tide stations. The model captures spatial and temporal features from historical tide level and meteorological data. Future tidal levels for multiple tide stations are the model's output. In this work, 48-year historical data from five tidal stations in Pearl River Delta were utilized for model training and evaluation. The results show that: (1) The model outperforms five commonly used baseline models in terms of evaluation metrics RMSE and MAE, and is able to predict future tide levels at multiple tide stations; (2) Short-term forecasts (1 and 3 h) are more accurate than long-term forecasts (12 h); (3) The model retains a high degree of accuracy for short-term predictions and satisfactory accuracy for long-term prediction during typhoons. The method provides a new instrument for regional prediction of tide levels and forecasting of storm surges.
更多查看译文
关键词
Multi-site tide,Tide level prediction,Machine learning,Graph convolutional recurrent networks
AI 理解论文
溯源树
样例
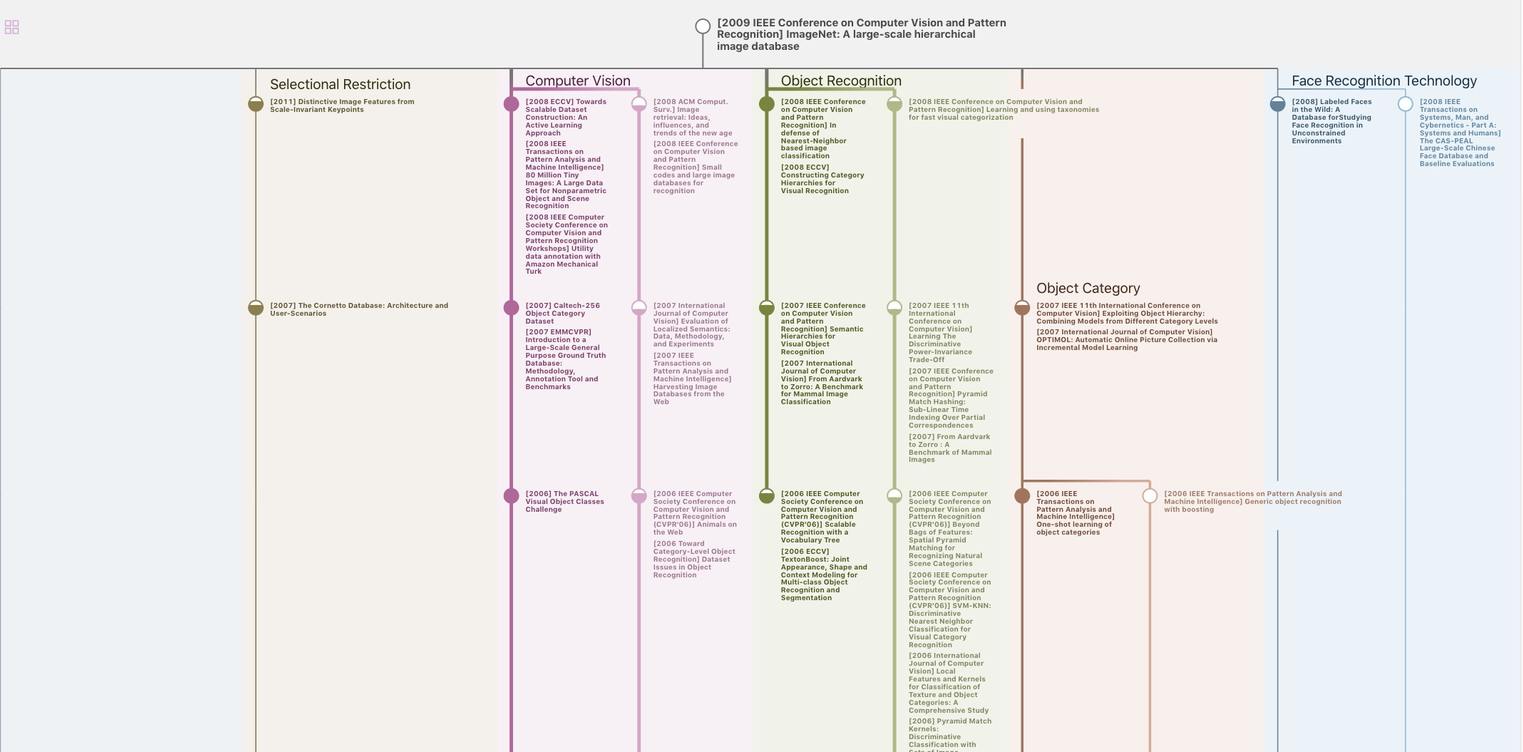
生成溯源树,研究论文发展脉络
Chat Paper
正在生成论文摘要