Rapid all-in-focus imaging via physical neural network optical encoding
Optics and Lasers in Engineering(2023)
摘要
Lightfield phase modulation has become an effective implementation for extending depth-of-field (DOF) of computational imaging. However, correct reconstruction of tiny details and color configuration with a fast capture is still challenging. Here, we demonstrate a high-dynamic all-focus imaging based on a proposed paradigm of constructing diffractive network encoding and electronic network decoding (DE-ED) conformation. With an efficient collaboration of physical diffractive layers and electronic convolutional layers, this learning-based model demonstrates significantly enhanced generalization and data-fitting capabilities. In experiments, the proposed method exhibits an obvious superiority in high-dynamic adaptation, autofocusing, and denoise compared with conventional methods. Specifically, an ultrahigh capture frame rate with ST <1/3000 s can be precisely adapted with only ∼5.6 mm diameter of imaging aperture under natural illumination. Several videos for proposed high-dynamic reconstruction show this method's time-efficiency and consistency. These results highlight some unique advantages of the constructed hybrid opto-electronic network model based on data-driven end-to-end learning for next-generation innovative computational imaging.
更多查看译文
关键词
Computational imaging,Optical neural network,All-in-focus reconstruction,Opto-electronic hybrid system
AI 理解论文
溯源树
样例
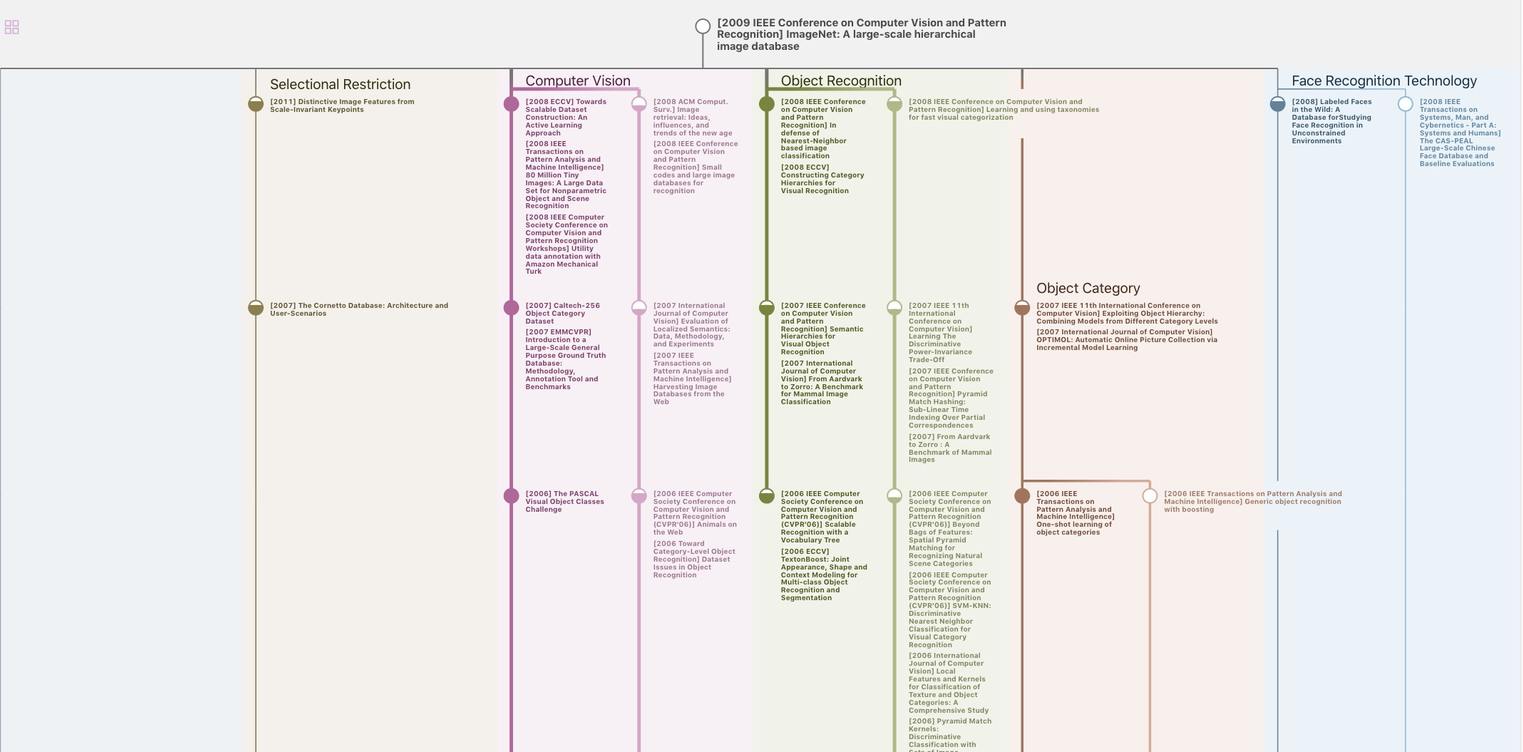
生成溯源树,研究论文发展脉络
Chat Paper
正在生成论文摘要