Easy and fast prediction of green solvents for small molecule donor-based organic solar cells through machine learning.
Physical chemistry chemical physics : PCCP(2023)
摘要
Solubility plays a critical role in many aspects of research (drugs to materials). Solubility parameters are very useful for selecting appropriate solvents/non-solvents for various applications. In the present study, Hansen solubility parameters are predicted using machine learning. More than 40 machine models are tried in the search for the best model. Molecular descriptors and fingerprints are used as inputs to get a comparative view. Machine learning models trained using molecular descriptors have shown higher prediction ability than the model trained using molecular fingerprints. Machine learning models trained using molecular descriptors have shown their potential to be easy and fast models compared to the density functional theory (DFT)/thermodynamic approach. Machine learning creates a "black box" connection to the properties. Therefore, minimal computational cost is required. With the help of the best-trained machine learning model, green solvents are selected for small molecule donors that are used in organic solar cells. Our introduced framework can help to select solvents for organic solar cells in an easy and fast way.
更多查看译文
关键词
organic solar cells,green solvents,machine learning,donor-based
AI 理解论文
溯源树
样例
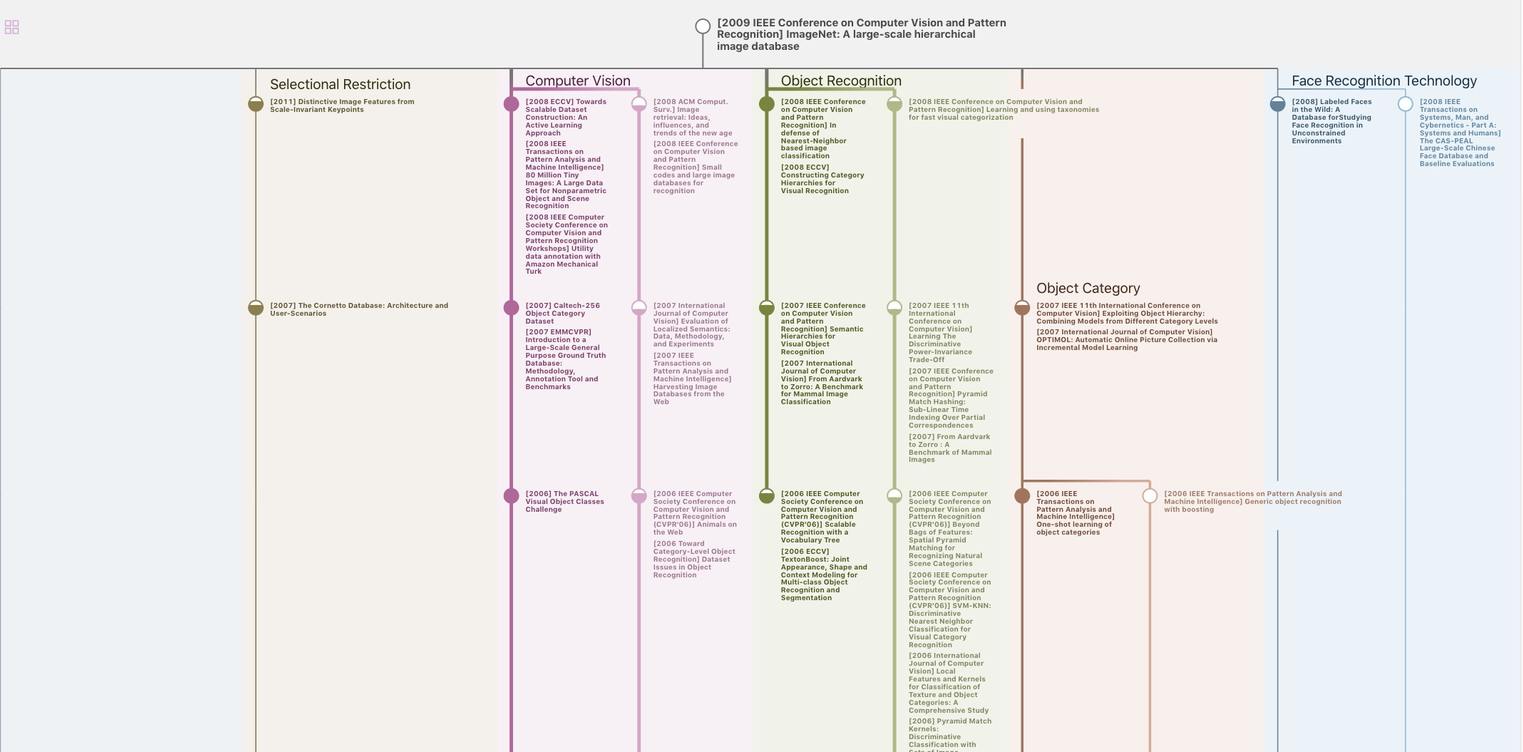
生成溯源树,研究论文发展脉络
Chat Paper
正在生成论文摘要