A Quantum-Classical Hybrid Solution for Deep Anomaly Detection.
Entropy (Basel, Switzerland)(2023)
摘要
Machine learning (ML) has achieved remarkable success in a wide range of applications. In recent ML research, deep anomaly detection (AD) has been a hot topic with the aim of discriminating among anomalous data with deep neural networks (DNNs). Notably, image AD is one of the most representative tasks in current deep AD research. ML's interaction with quantum computing is giving rise to a heated topic named quantum machine learning (QML), which enjoys great prospects according to recent academic research. This paper attempts to address the image AD problem in a deep manner with a novel QML solution. Specifically, we design a quantum-classical hybrid DNN (QHDNN) that aims to learn directly from normal raw images to train a normality model and then exclude images that do not conform to this model as anomalies during its inference. To enable the QHDNN to perform satisfactorily in deep image AD, we explore multiple quantum layer architectures and design a VQC-based QHDNN solution. Extensive experiments were conducted on commonly used benchmarks to test the proposed QML solution, whose results demonstrate the feasibility of addressing deep image AD with QML. Importantly, the experimental results show that our quantum-classical hybrid solution can even yield superior performance to that of its classical counterpart when they share the same number of learnable parameters.
更多查看译文
关键词
deep learning,image anomaly detection,quantum hybrid deep neural network,quantum machine learning
AI 理解论文
溯源树
样例
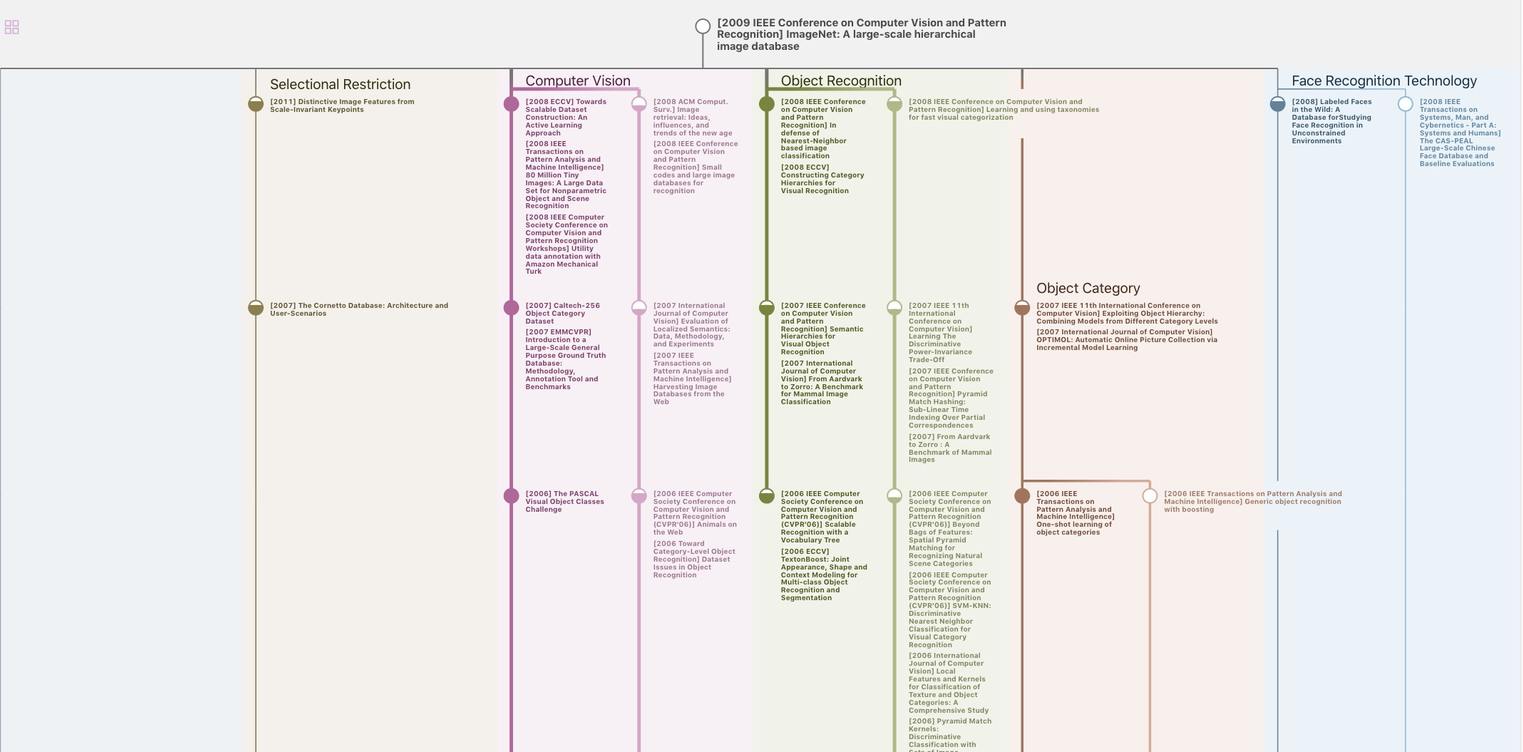
生成溯源树,研究论文发展脉络
Chat Paper
正在生成论文摘要