Text classification on heterogeneous information network via enhanced GCN and knowledge
Neural Computing and Applications(2023)
摘要
Graph convolutional networks-based text classification methods have shown impressive success in further improving the classification results by considering the structural relationship between words and texts. However, existing GCN-based text classification methods tend to ignore the semantic representation of the node and the global structural information among nodes. Besides, only the word granularity information within the text, i.e., endogenous source, is used to represent the text. Furthermore, the existing graph convolutional network approaches are faced with major challenges to handle large and dense graphs, i.e., neighbor explosion and noisy inputs. To address these shortcomings, this paper proposes an inductive learning-based text classification method that utilizes representation learning on heterogeneous information networks and exogenous knowledge. Firstly, a weighted heterogeneous information network for text (HINT) is constructed by introducing exogenous knowledge, in which the node types cover text, entities and words. The unstructured text is represented as a structured heterogeneous information network, which expands the granularity of text features and makes full use of the exogenous structural information and explicit semantic information to enhance the interpretability of text information. Besides, we also enhanced the graph neural network against the challenges of neighbor explosion and noisy inputs derived from HINT using two strategies: graph sampling and Dropedge, for semi-supervised learning with improved classification performance. The effectiveness of our model is demonstrated by examining four publicly available text classification datasets. Based on experimental results, our approach achieves state-of-the-art performance on the text classification datasets.
更多查看译文
关键词
Text classification,Graph convolutional networks,Knowledge graph,Heterogeneous information network,Pre-trained model
AI 理解论文
溯源树
样例
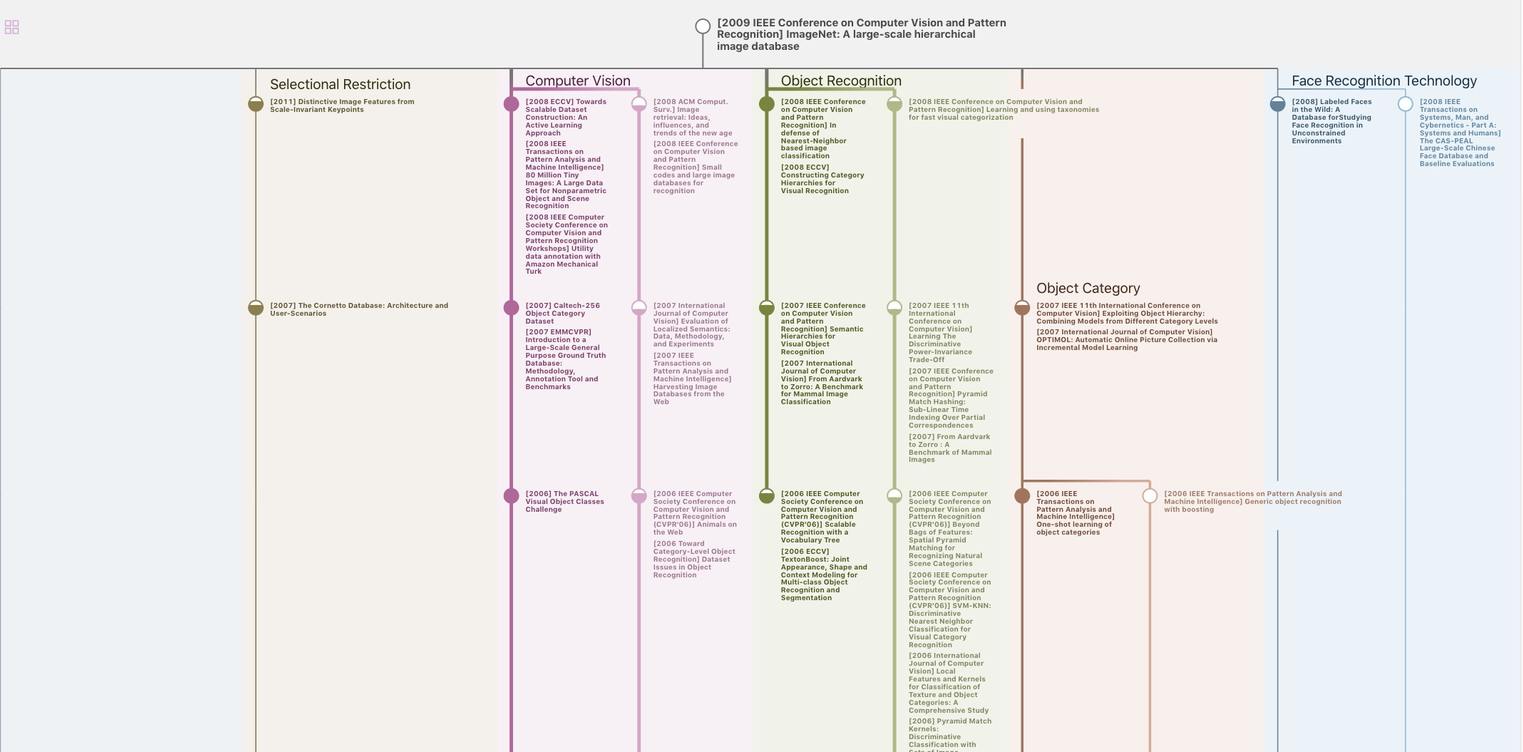
生成溯源树,研究论文发展脉络
Chat Paper
正在生成论文摘要