Exploring the Effect of High-frequency Components in GANs Training
ACM Transactions on Multimedia Computing, Communications, and Applications(2023)
摘要
Generative Adversarial Networks (GANs) have the ability to generate images that are visually indistinguishable from real images. However, recent studies have revealed that generated and real images share significant differences in the frequency domain. In this article, we argue that the frequency gap is caused by the high-frequency sensitivity of the discriminator. According to our observation, during the training of most GANs, severe high-frequency differences make the discriminator focus on high-frequency components excessively, which hinders the generator from fitting the low-frequency components that are important for learning images' content. Then, we propose two simple yet effective image pre-processing operations in the frequency domain for eliminating the side effects caused by high-frequency differences in GANs training: High-frequency Confusion (HFC) and High-frequency Filter (HFF). The proposed operations are general and can be applied to most existing GANs at a fraction of the cost. The advanced performance of the proposed operations is verified on multiple loss functions, network architectures, and datasets. Specifically, the proposed HFF achieves significant improvements of 42.5% FID on CelebA (128*128) unconditional generation based on SNGAN, 30.2% FID on CelebA unconditional generation based on SSGAN, and 69.3% FID on CelebA unconditional generation based on InfoMAXGAN. Furthermore, we also adopt HFF as the first attempt at data augmentation in the frequency domain for contrastive learning, achieving state-of-the-art performance on unconditional generation. Code is available at https://github.com/iceli1007/HFC-and-HFF.
更多查看译文
关键词
Generative Adversarial Networks,frequency,contrastive learning,data augmentation
AI 理解论文
溯源树
样例
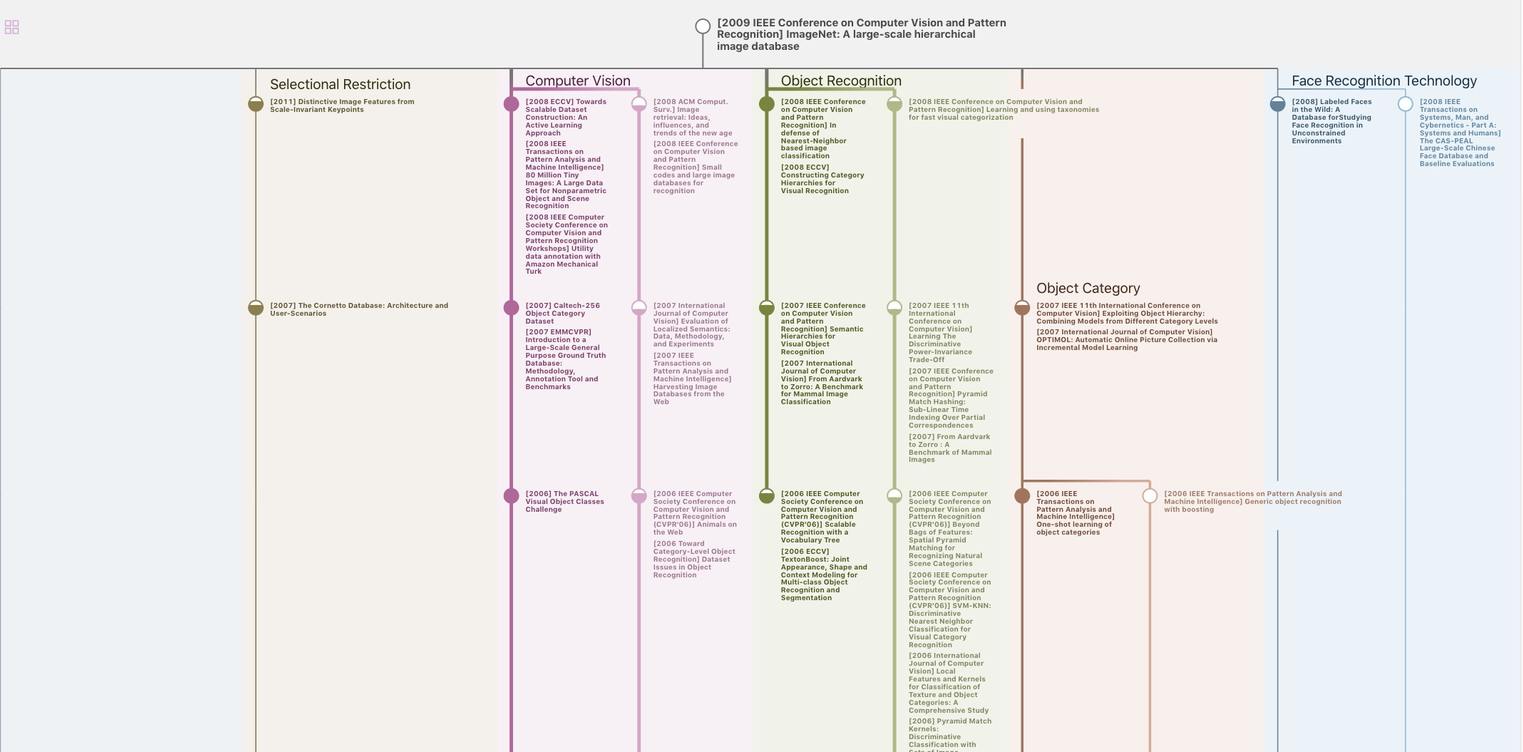
生成溯源树,研究论文发展脉络
Chat Paper
正在生成论文摘要