Toward Precision Medicine: Development and Validation of A Machine Learning Based Decision Support System for Optimal Sequencing in Castration-Resistant Prostate Cancer
CLINICAL GENITOURINARY CANCER(2023)
摘要
Selecting a patient-specific sequencing strategy to maximize survival outcomes is a clinically unmet need for patients with castration-resistant prostate cancer. We developed an artificial intelligence-based decision support system (DSS) using XGB models, which provides visualization of individualized survival outcomes with high predictive accuracy. Our DSS can be integrated into any electronic medical records system that uses coding algorithms. Introduction: Selecting a patient-specific sequencing strategy to maximize survival outcomes is a clinically unmet need for patients with castration-resistant prostate cancer (CRPC). We developed and validated an artificial intelligence-based decision support system (DSS) to guide optimal sequencing strategy selection. Patients and Methods: Clinicopatho-logical data of 46 covariates were retrospectively collected from 801 patients diagnosed with CRPC at 2 high-volume institutions between February 2004 and March 2021. Cox-propor tional hazards regression survival (Cox) modeling in extreme gradient boosting (XGB) was used to perform survival analysis for cancer-specific mortality (CSM) and overall mortality (OM) according to the use of abiraterone acetate, cabazitaxel, docetaxel, and enzalutamide. The models were further stratified into first-, second-, and third-line models that each provided CSM and OM estimates for each line of treatment. The performances of the XGB models were compared with those of the Cox models and random survival forest (RSF) models in terms of Harrell's C-index. Results: The XGB models showed greater predictive performance for CSM and OM compared to the RSF and Cox models. C-indices of 0.827, 0.807, and 0.748 were achieved for CSM in the first-, second-, and third-lines of treatment, respectively, while C-indices of 0.822, 0.813, and 0.729 were achieved for OM regarding each line of treatment, respectively. An online DSS was developed to provide visualization of individ-ualized survival outcomes according to each line of sequencing strategy. Conclusion: Our DSS can be used in clinical practice by physicians and patients as a visualized tool to guide the sequencing strategy of CRPC agents.
更多查看译文
AI 理解论文
溯源树
样例
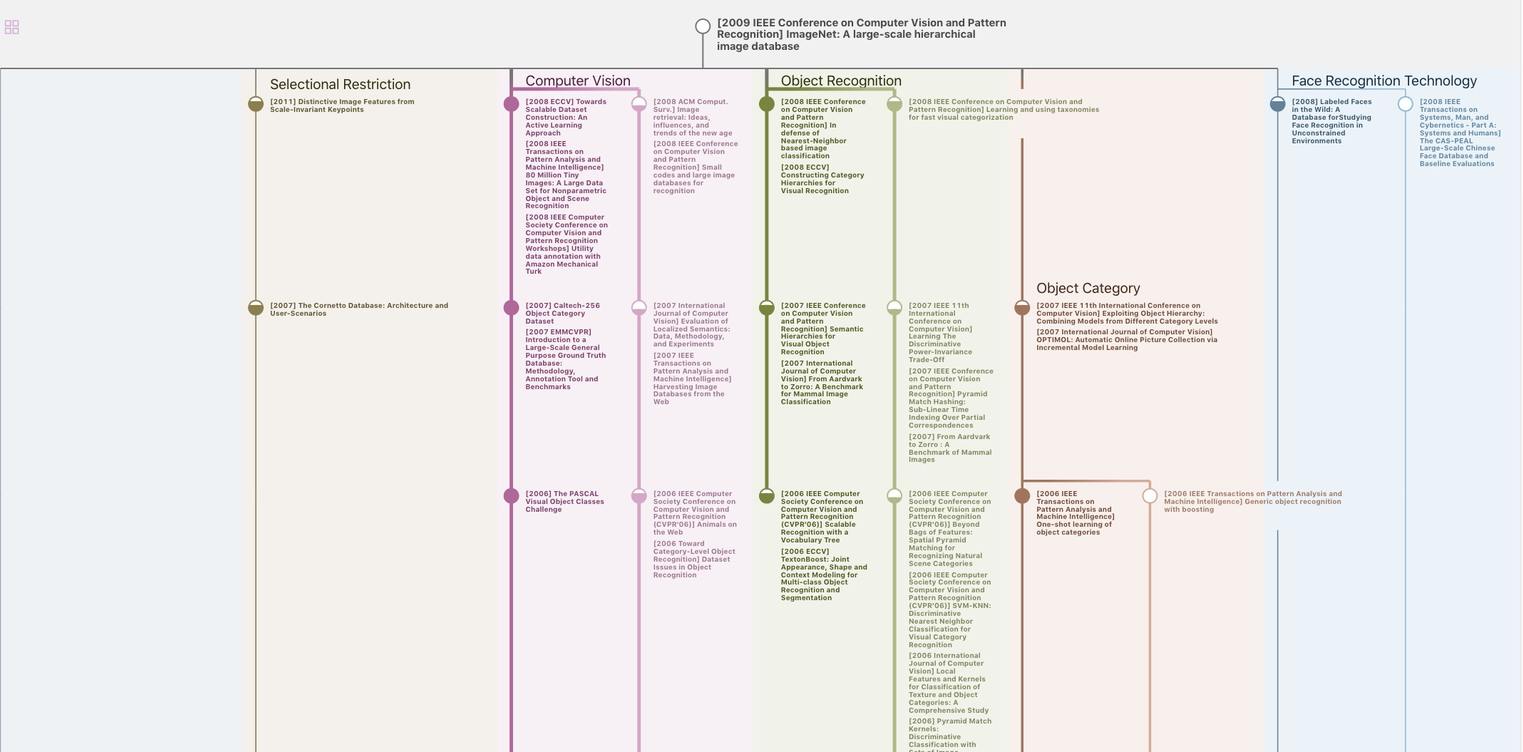
生成溯源树,研究论文发展脉络
Chat Paper
正在生成论文摘要