Zero-shot Entailment of Leaderboards for Empirical AI Research
2023 ACM/IEEE JOINT CONFERENCE ON DIGITAL LIBRARIES, JCDL(2023)
Abstract
We present a large-scale empirical investigation of the zero-shot learning phenomena in a specific recognizing textual entailment (RTE) task category, i.e., the automated mining of leaderboards for Empirical AI Research. The prior reported state-of-the-art models for leaderboards extraction formulated as an RTE task in a non-zero-shot setting are promising with above 90% reported performances. However, a central research question remains unexamined: did the models actually learn entailment? Thus, for the experiments in this paper, two prior reported state-of-the-art models are tested out-of-the-box for their ability to generalize or their capacity for entailment, given leaderboard labels that were unseen during training. We hypothesize that if the models learned entailment, their zero-shot performances can be expected to be moderately high as well-perhaps, concretely, better than chance. As a result of this work, a zero-shot labeled dataset is created via distant labeling, formulating the leaderboard extraction RTE task.
MoreTranslated text
Key words
Natural-Language-Inference,Entailment,Leaderboard,InformationExtraction
AI Read Science
Must-Reading Tree
Example
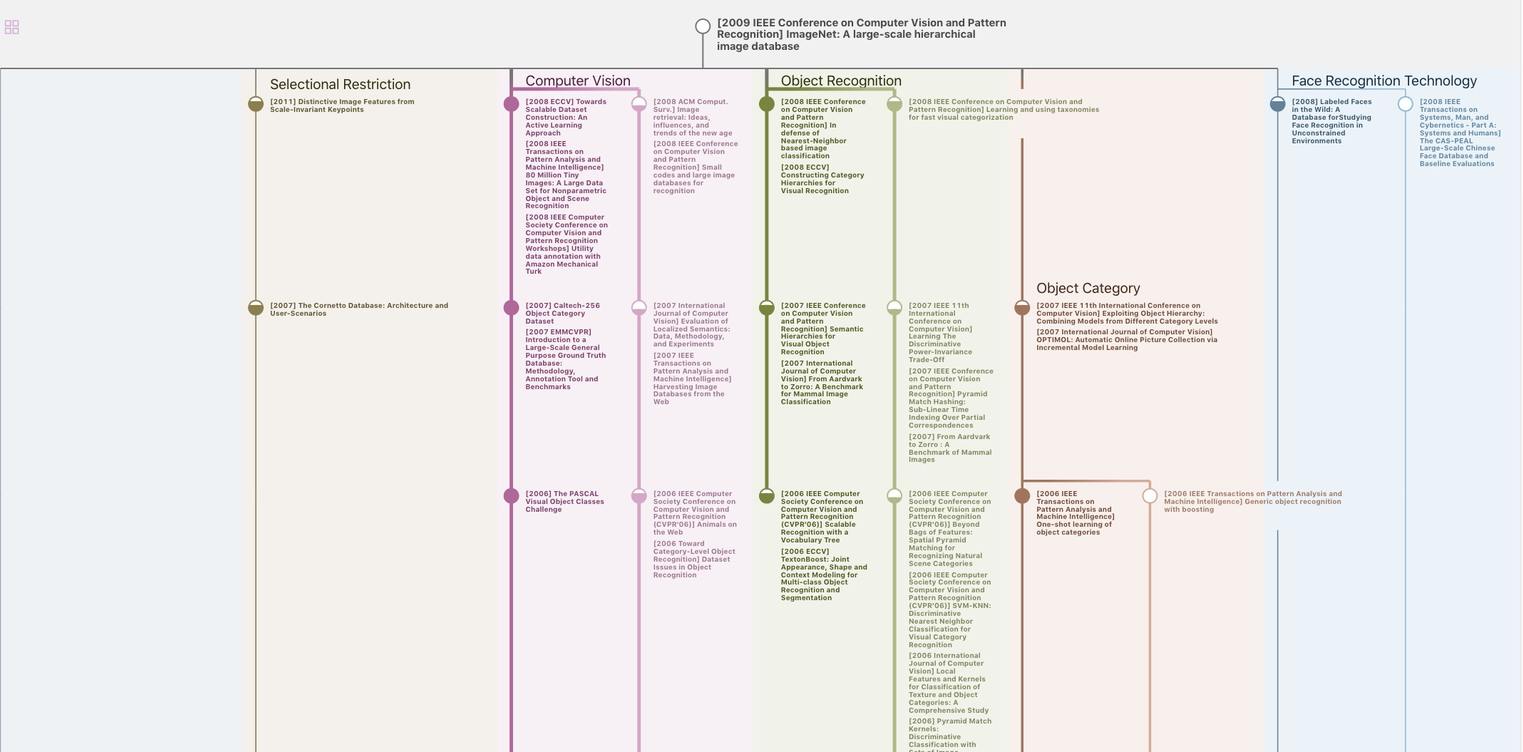
Generate MRT to find the research sequence of this paper
Chat Paper
Summary is being generated by the instructions you defined