Super-resolution of three-dimensional temperature and velocity for building-resolving urban micrometeorology using physics-guided convolutional neural networks with image inpainting techniques
Building and Environment(2023)
Abstract
This study proposes a convolutional neural network (CNN) that enhances the resolution of instantaneous snapshots of three-dimensional air temperature and wind velocity fields around buildings in urban areas. The CNN not only increases the resolution of flow fields but also recovers the missing data associated with changes in resolution-dependent building shapes. The proposed CNN incorporates gated convolution, which is an image inpainting technique that infers missing pixels, to improve accuracy. The CNN performance has been verified via supervised learning utilizing building-resolving micrometeorological simulations around Tokyo Station in Japan. The CNN has successfully reconstructed the temperature and velocity fields around the high-resolution buildings, despite the missing data at lower altitudes due to the coarseness of the low-resolution buildings. This result implies that near-surface flows can be inferred from flows above buildings. This hypothesis has been assessed through numerical experiments in which all inputs below a certain height are set as missing values. This research suggests that airflows around buildings can be efficiently estimated by combining neural network inferences and low-resolution fluid simulations.
MoreTranslated text
Key words
Super-resolution,Image inpainting,Convolutional neural network,Building-resolving micrometeorological model,Large eddy simulation
AI Read Science
Must-Reading Tree
Example
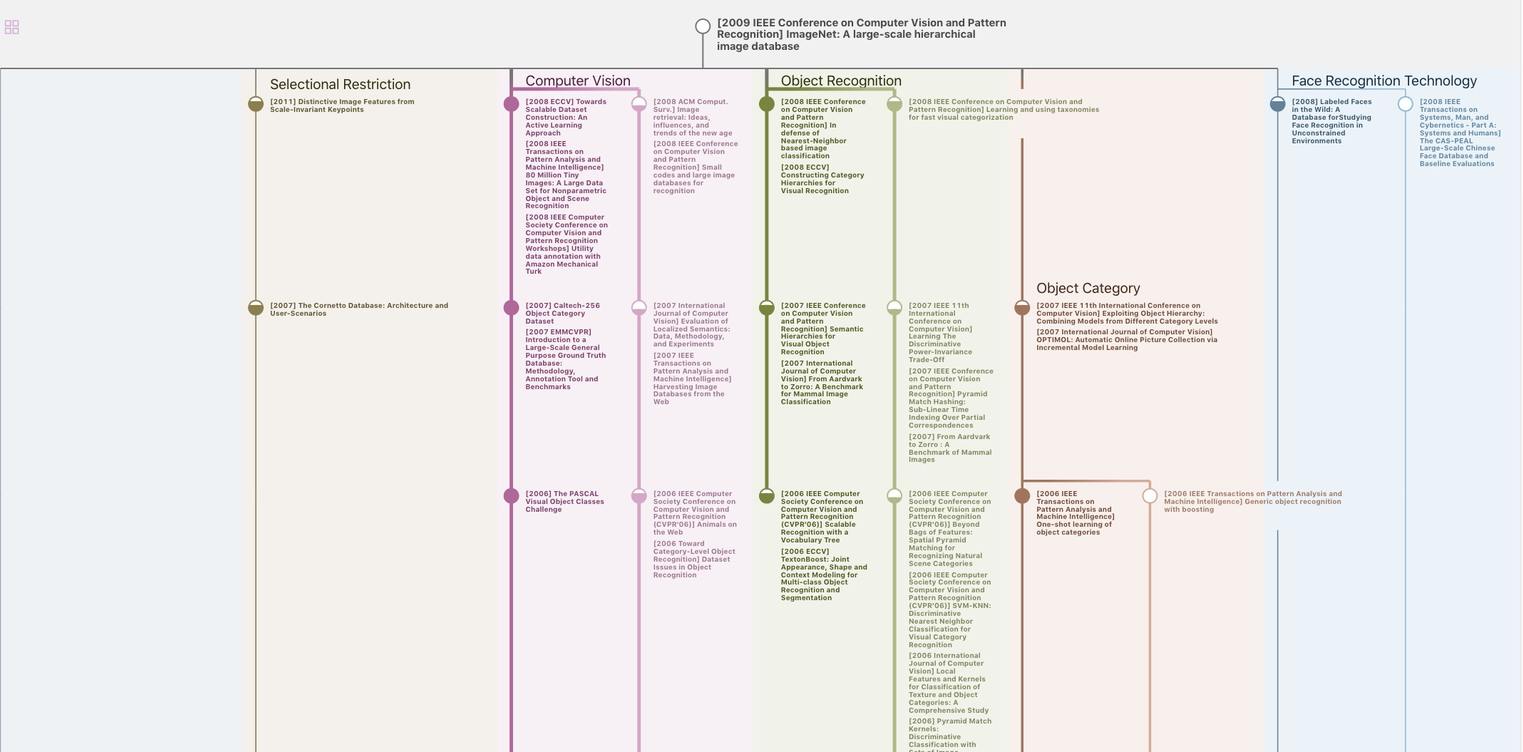
Generate MRT to find the research sequence of this paper
Chat Paper
Summary is being generated by the instructions you defined