Lifting Uniform Learners via Distributional Decomposition
PROCEEDINGS OF THE 55TH ANNUAL ACM SYMPOSIUM ON THEORY OF COMPUTING, STOC 2023(2023)
摘要
We show how any PAC learning algorithm that works under the uniform distribution can be transformed, in a blackbox fashion, into one thatworks under an arbitrary and unknown distributionD. The efficiency of our transformation scales with the inherent complexity of D, running in poly(n, (md)(d)) time for distributions over {+/- 1}(n) whose pmfs are computed by depth-.. decision trees, where m is the sample complexity of the original algorithm. For monotone distributions our transformation uses only samples from D, and for general ones it uses subcube conditioning samples. A key technical ingredient is an algorithm which, given the aforementioned access to D, produces an optimal decision tree decomposition of D: an approximation of D as a mixture of uniform distributions over disjoint subcubes. With this decomposition in hand, we run the uniform-distribution learner on each subcube and combine the hypotheses using the decision tree. This algorithmic decomposition lemma also yields new algorithms for learning decision tree distributions with runtimes that exponentially improve on the prior state of the art-results of independent interest in distribution learning.
更多查看译文
关键词
PAC Learning,Semi-supervised learning,Decision tree decomposition
AI 理解论文
溯源树
样例
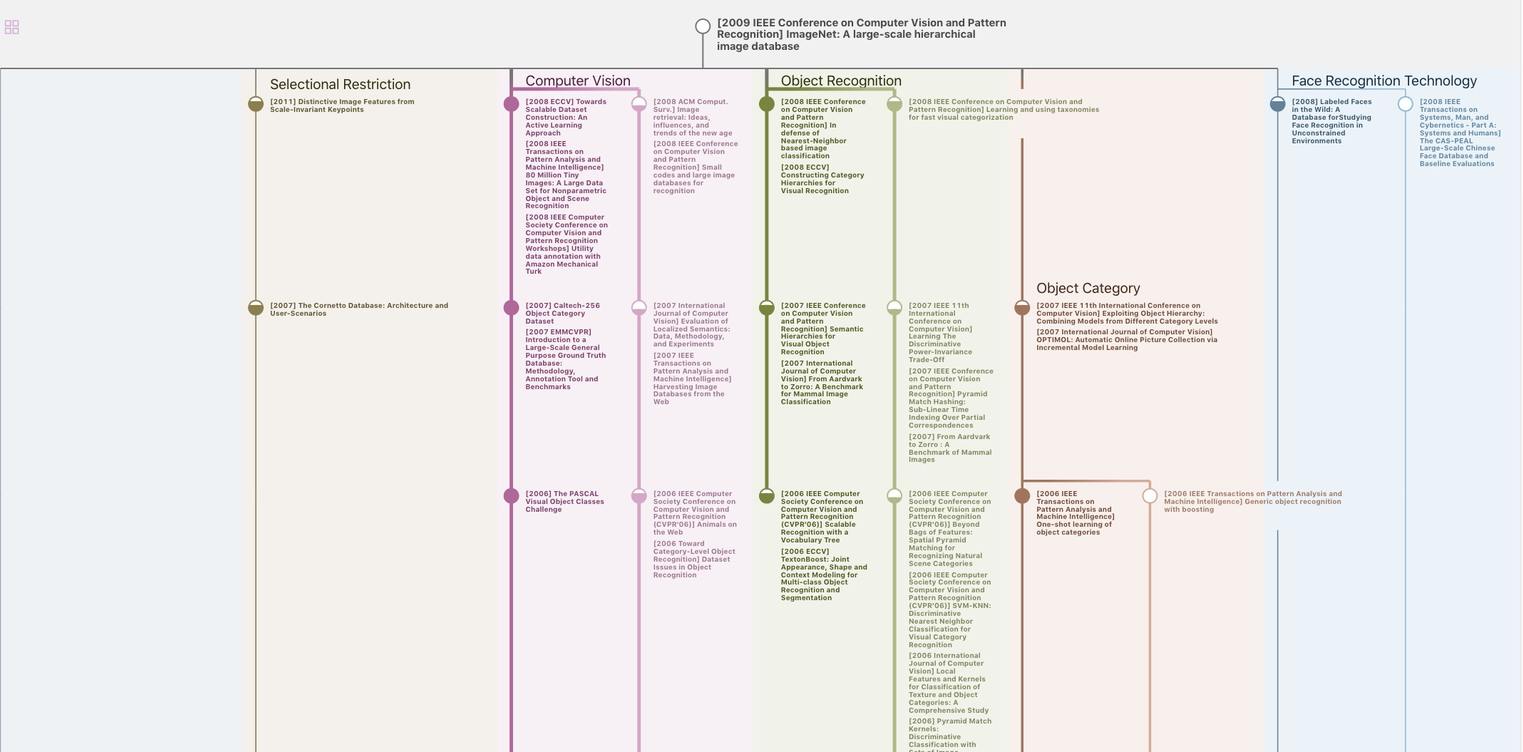
生成溯源树,研究论文发展脉络
Chat Paper
正在生成论文摘要