Prediction of acute hypertensive episodes in critically ill patients
Artificial Intelligence in Medicine(2023)
摘要
Prevention and treatment of complications are the backbone of medical care, particularly in critical care settings. Early detection and prompt intervention can potentially prevent complications from occurring and improve outcomes. In this study, we use four longitudinal vital signs variables of intensive care unit patients, focusing on predicting acute hypertensive episodes (AHEs). These episodes represent elevations in blood pressure and may result in clinical damage or indicate a change in a patient's clinical situation, such as an elevation in intracranial pressure or kidney failure. Prediction of AHEs may allow clinicians to anticipate changes in the patient's condition and respond early on to prevent these from occurring. Temporal abstraction was employed to transform the multivariate temporal data into a uniform representation of symbolic time intervals, from which frequent time-intervals-related patterns (TIRPs) are mined and used as features for AHE prediction. A novel TIRP metric for classification, called coverage, is introduced that measures the coverage of a TIRP's instances in a time window. For comparison, several baseline models were applied on the raw time series data, including logistic regression and sequential deep learning models, are used. Our results show that using frequent TIRPs as features outperforms the baseline models, and the use of the coverage, metric outperforms other TIRP metrics. Two approaches to predicting AHEs in real-life application conditions are evaluated: using a sliding window to continuously predict whether a patient would experience an AHE within a specific prediction time period ahead, our models produced an AUC-ROC of 82%, but with low AUPRC. Alternatively, predicting whether an AHE would generally occur during the entire admission resulted in an AUC-ROC of 74%.
更多查看译文
关键词
Intensive care units,Time intervals,Temporal patterns,Outcome prediction
AI 理解论文
溯源树
样例
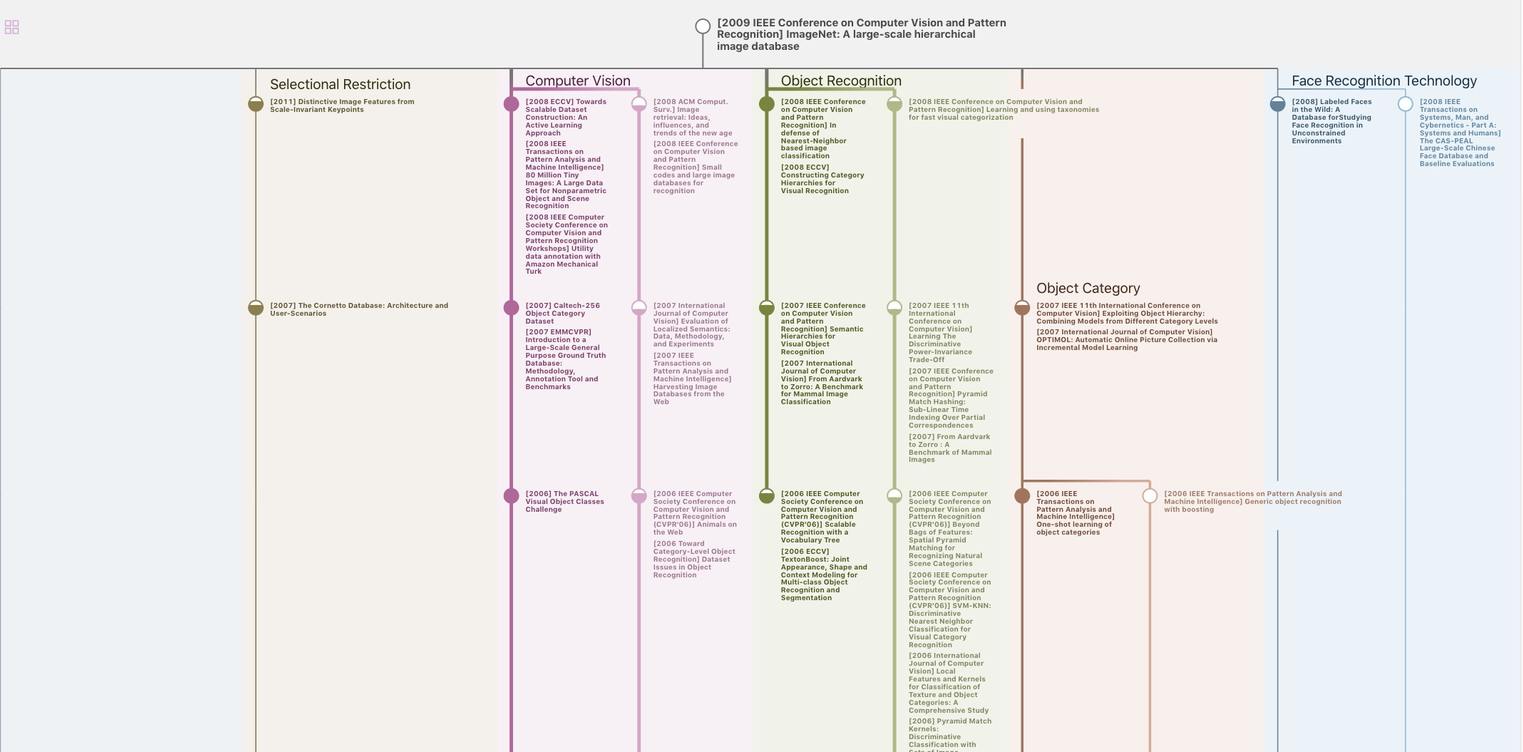
生成溯源树,研究论文发展脉络
Chat Paper
正在生成论文摘要