Hierarchical reinforcement learning for transportation infrastructure maintenance planning
RELIABILITY ENGINEERING & SYSTEM SAFETY(2023)
摘要
Maintenance planning on bridges commonly faces multiple challenges, mainly related to complexity and scale. Those challenges stem from the large number of structural elements in each bridge in addition to the uncertainties surrounding their health condition, which is monitored using visual inspections at the element -level. Recent developments have relied on deep reinforcement learning (RL) for solving maintenance planning problems, with the aim to minimize the long-term costs. Nonetheless, existing RL based solutions have adopted approaches that often lacked the capacity to scale due to the inherently large state and action spaces. The aim of this paper is to introduce a hierarchical RL formulation for maintenance planning, which naturally adapts to the hierarchy of information and decisions in infrastructure. The hierarchical formulation enables decomposing large state and action spaces into smaller ones, by relying on state and temporal abstraction. An additional contribution from this paper is the development of an open-source RL environment that uses state-space models (SSM) to describe the propagation of the deterioration condition and speed over time. The functionality of this new environment is demonstrated by solving maintenance planning problems at the element-level, and the bridge-level.
更多查看译文
关键词
Maintenance planning,Reinforcement learning,RL environment,Deep Q-learning,Infrastructure deterioration,State-space models
AI 理解论文
溯源树
样例
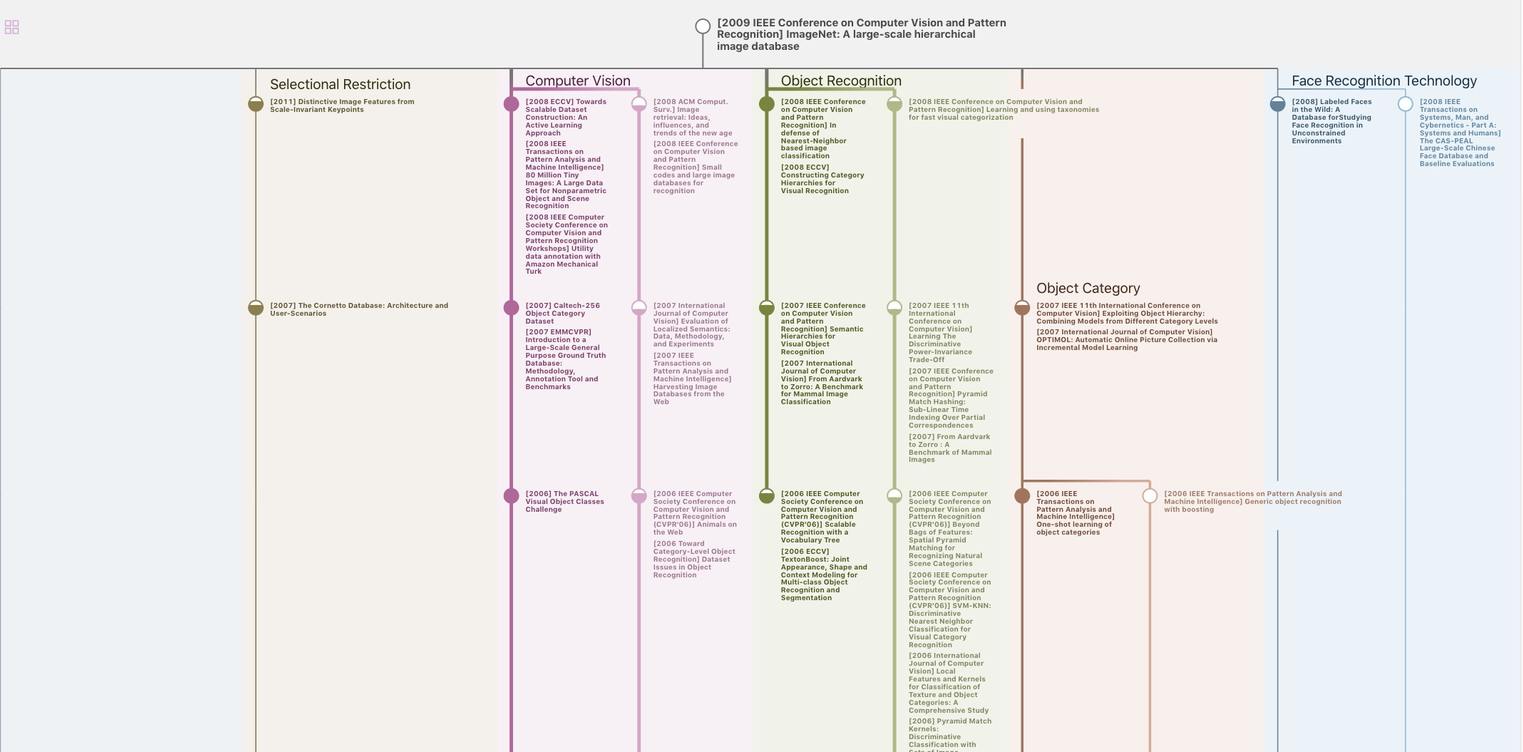
生成溯源树,研究论文发展脉络
Chat Paper
正在生成论文摘要