Adaptive Riemannian Metrics on SPD Manifolds
CoRR(2023)
摘要
Symmetric Positive Definite (SPD) matrices have received wide attention in machine learning due to their intrinsic capacity of encoding underlying structural correlation in data. To reflect the non-Euclidean geometry of SPD manifolds, many successful Riemannian metrics have been proposed. However, existing fixed metric tensors might lead to sub-optimal performance for SPD matrices learning, especially for SPD neural networks. To remedy this limitation, we leverage the idea of pullback and propose adaptive Riemannian metrics for SPD manifolds. Moreover, we present comprehensive theories for our metrics. Experiments on three datasets demonstrate that equipped with the proposed metrics, SPD networks can exhibit superior performance.
更多查看译文
关键词
spd manifolds
AI 理解论文
溯源树
样例
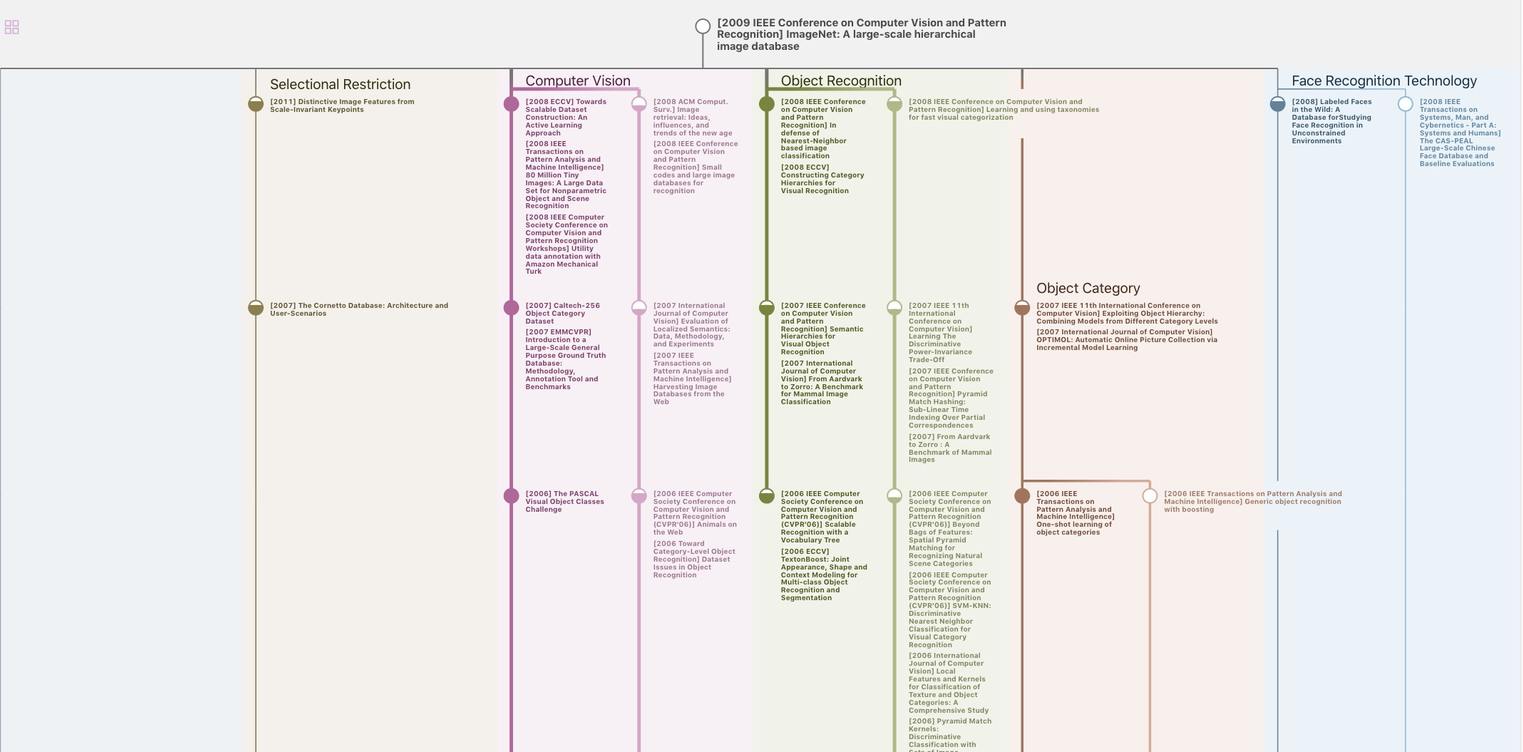
生成溯源树,研究论文发展脉络
Chat Paper
正在生成论文摘要