Embedding Contextual Information through Reward Shaping in Multi-Agent Learning: A Case Study from Google Football
CoRR(2023)
摘要
Artificial Intelligence has been used to help human complete difficult tasks in complicated environments by providing optimized strategies for decision-making or replacing the manual labour. In environments including multiple agents, such as football, the most common methods to train agents are Imitation Learning and Multi-Agent Reinforcement Learning (MARL). However, the agents trained by Imitation Learning cannot outperform the expert demonstrator, which makes humans hardly get new insights from the learnt policy. Besides, MARL is prone to the credit assignment problem. In environments with sparse reward signal, this method can be inefficient. The objective of our research is to create a novel reward shaping method by embedding contextual information in reward function to solve the aforementioned challenges. We demonstrate this in the Google Research Football (GRF) environment. We quantify the contextual information extracted from game state observation and use this quantification together with original sparse reward to create the shaped reward. The experiment results in the GRF environment prove that our reward shaping method is a useful addition to state-of-the-art MARL algorithms for training agents in environments with sparse reward signal.
更多查看译文
关键词
Multi-Agent Reinforcement Learning, reward shaping, Google Research Football
AI 理解论文
溯源树
样例
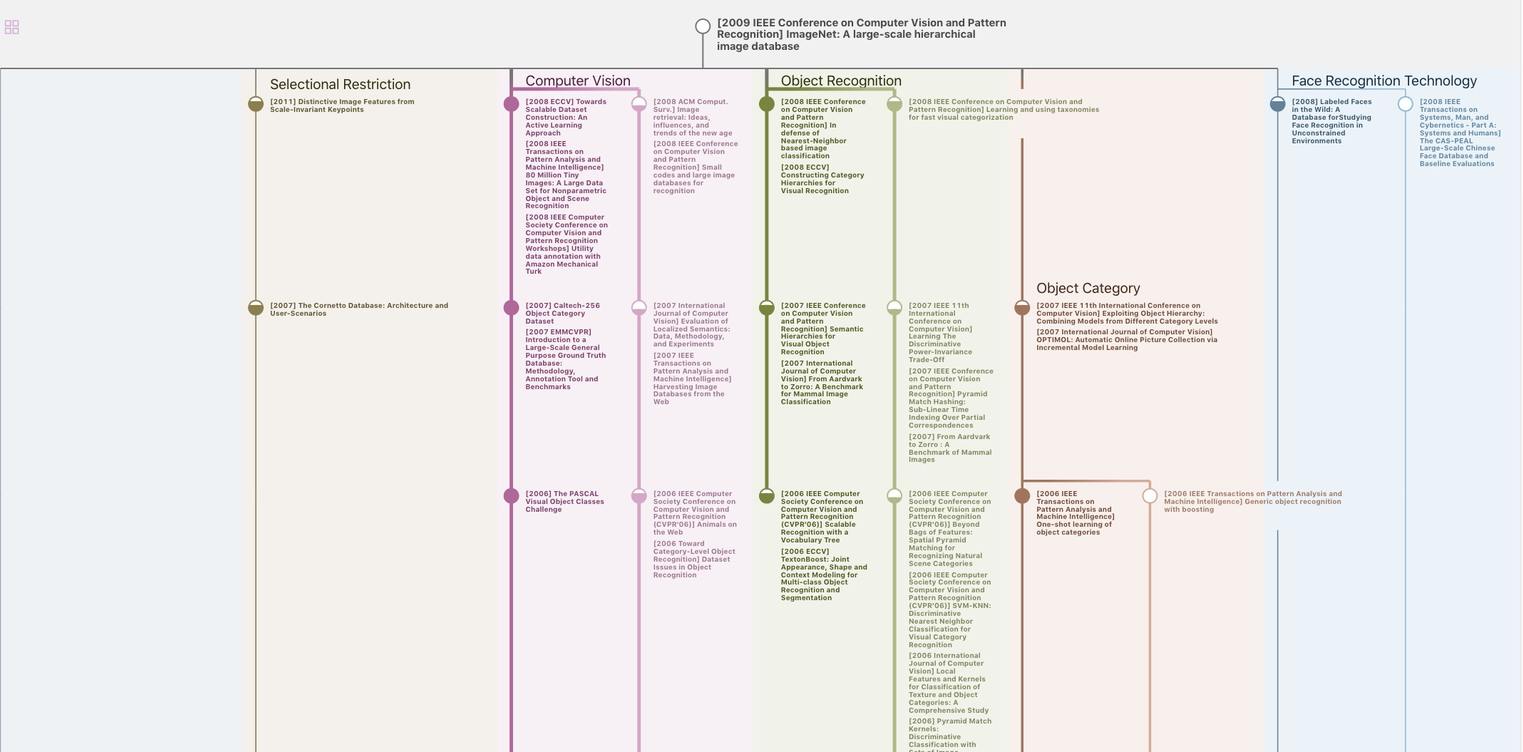
生成溯源树,研究论文发展脉络
Chat Paper
正在生成论文摘要