Applying Monte Carlo Dropout to Quantify the Uncertainty of Skip Connection-Based Convolutional Neural Networks Optimized by Big Data
Electronics(2023)
摘要
Although Deep Learning (DL) models have been introduced in various fields as effective prediction tools, they often do not care about uncertainty. This can be a barrier to their adoption in real-world applications. The current paper aims to apply and evaluate Monte Carlo (MC) dropout, a computationally efficient approach, to investigate the reliability of several skip connection-based Convolutional Neural Network (CNN) models while keeping their high accuracy. To do so, a high-dimensional regression problem is considered in the context of subterranean fluid flow modeling using 376,250 generated samples. The results demonstrate the effectiveness of MC dropout in terms of reliability with a Standard Deviation (SD) of 0.012-0.174, and of accuracy with a coefficient of determination (R-2) of 0.7881-0.9584 and Mean Squared Error (MSE) of 0.0113-0.0508, respectively. The findings of this study may contribute to the distribution of pressure in the development of oil/gas fields.
更多查看译文
关键词
deep learning,Monte Carlo dropout,reliability,regression,fluid flow modeling,mixed GMsFEM,standard deviation
AI 理解论文
溯源树
样例
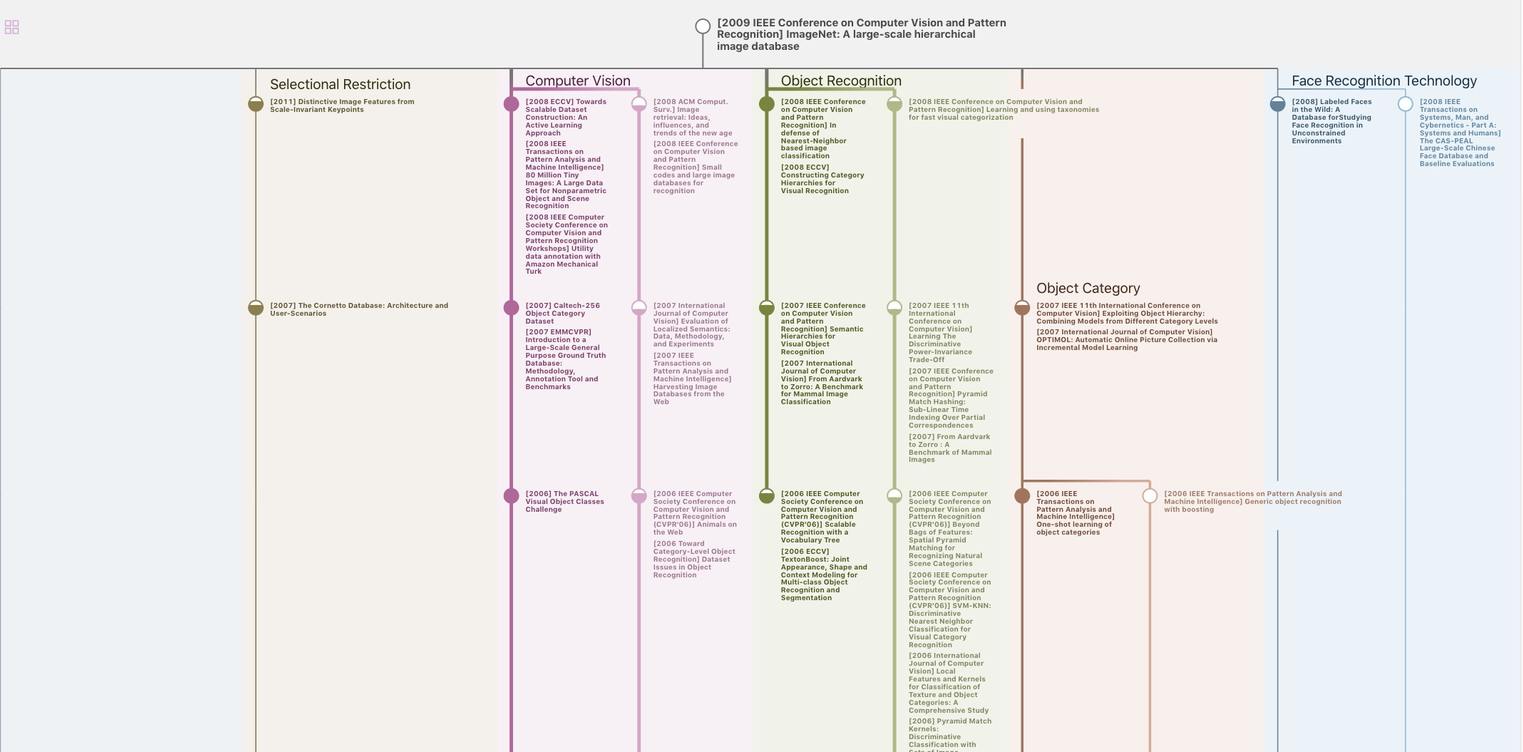
生成溯源树,研究论文发展脉络
Chat Paper
正在生成论文摘要