Data Augmentation for Neutron Spectrum Unfolding with Neural Networks
JOURNAL OF NUCLEAR ENGINEERING(2023)
关键词
detector response unfolding,neutron spectrum unfolding,machine learning,neural network,feature engineering
AI 理解论文
溯源树
样例
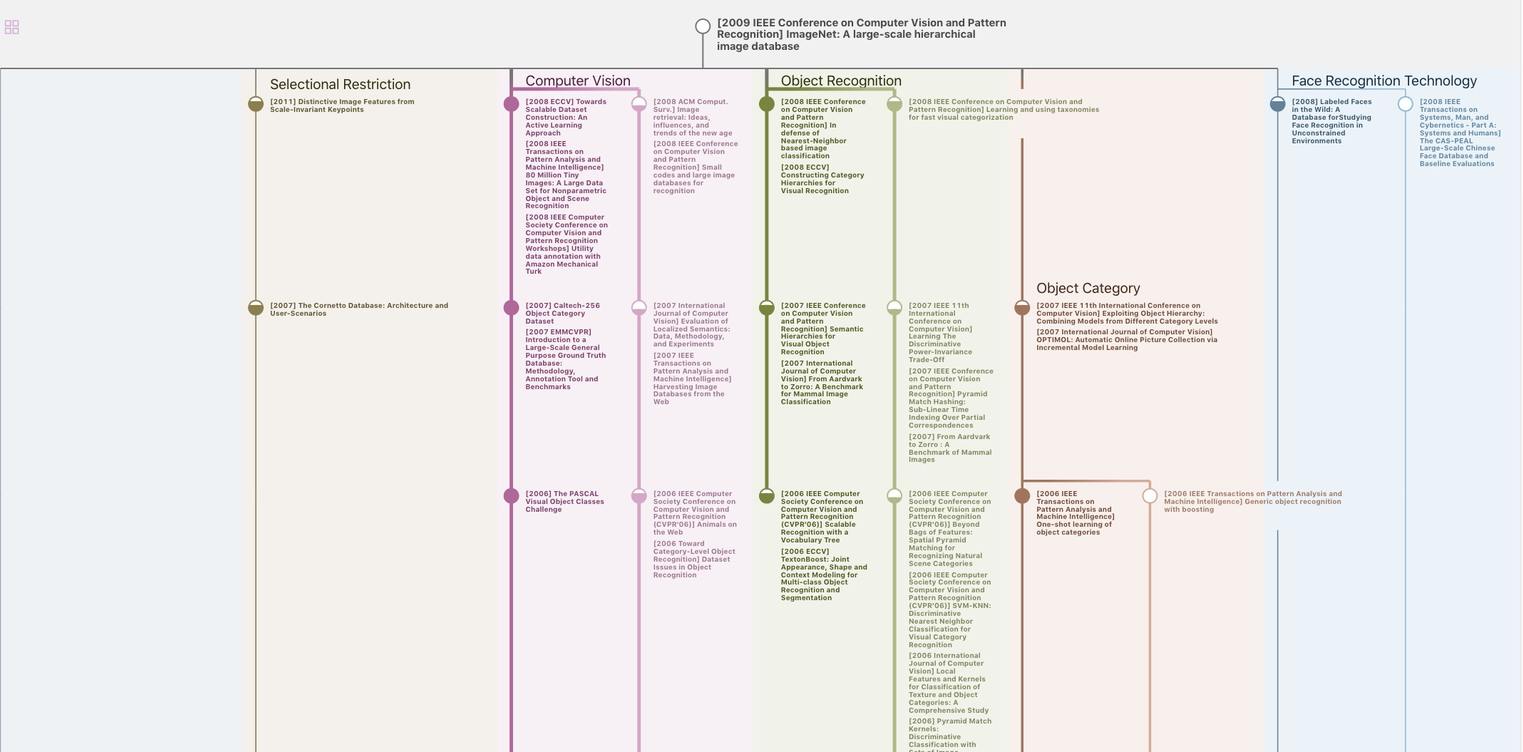
生成溯源树,研究论文发展脉络
Chat Paper
正在生成论文摘要