Improved deep convolutional dictionary learning with no noise parameter for low-dose CT image processing
JOURNAL OF X-RAY SCIENCE AND TECHNOLOGY(2023)
Abstract
Background: Low-Dose computed tomography (LDCT) reduces radiation damage to patients, however, the reconstructed images contain severe noise, which affects doctors' diagnosis of the disease. The convolutional dictionary learning has the advantage of the shift-invariant property. The deep convolutional dictionary learning algorithm (DCDicL) combines deep learning and convolutional dictionary learning, which has great suppression effects on Gaussian noise. However, applying DCDicL to LDCT images cannot get satisfactory results. Objective: To address this challenge, this study proposes and tests an improved deep convolutional dictionary learning algorithm for LDCT image processing and denoising. Methods: First, we use a modified DCDicL algorithm to improve the input network and make it do not need to input noise intensity parameter. Second, we use DenseNet121 to replace the shallow convolutional network to learn the prior on the convolutional dictionary, which can obtain more accurate convolutional dictionary. Last, in the loss function, we add MSSIM to enhance the detail retention ability of the model. Results: The experimental results on the Mayo dataset showthat the proposed model obtained an average value of 35.2975 dB in PSNR, which is 0.2954 - 1.0573 dB higher than the mainstream LDCT algorithm, indicating the excellent denoising performance. Conclusion: The study demonstrates that the proposed new algorithm can effectively improve the quality of LDCT images acquired in the clinical practice.
MoreTranslated text
Key words
Low-dose computed tomography,DenseNet,deep learning,convolutional dictionary learning
AI Read Science
Must-Reading Tree
Example
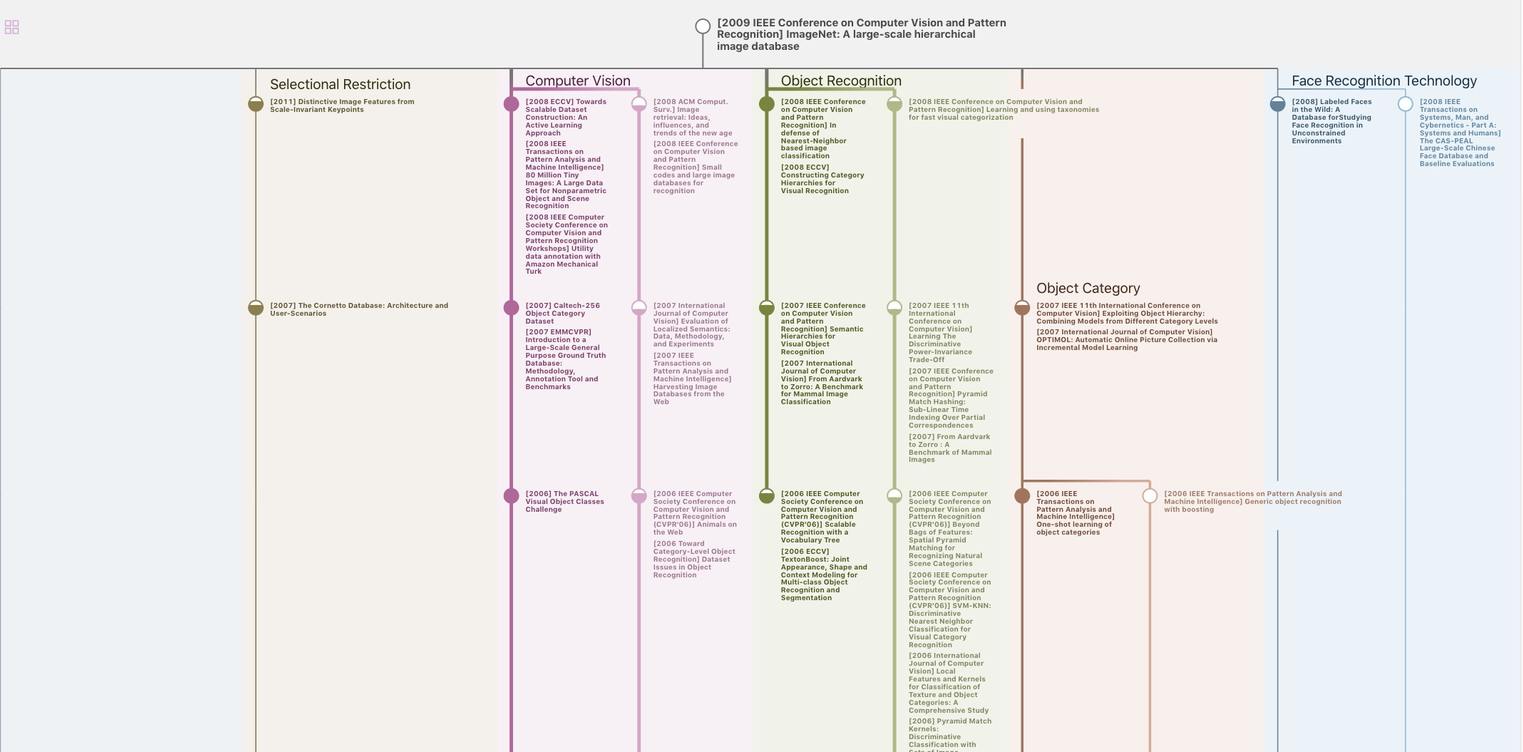
Generate MRT to find the research sequence of this paper
Chat Paper
Summary is being generated by the instructions you defined