Deep convolutional neural networks using an active learning strategy for cervical cancer screening and diagnosis
Frontiers in Bioinformatics(2023)
Abstract
Cervical cancer (CC) is the fourth most common malignant tumor among women worldwide. Constructing a high-accuracy deep convolutional neural network (DCNN) for cervical cancer screening and diagnosis is important for the successful prevention of cervical cancer. In this work, we proposed a robust DCNN for cervical cancer screening using whole-slide images (WSI) of ThinPrep cytologic test (TCT) slides from 211 cervical cancer and 189 normal patients. We used an active learning strategy to improve the efficiency and accuracy of image labeling. The sensitivity, specificity, and accuracy of the best model were 96.21%, 98.95%, and 97.5% for CC patient identification respectively. Our results also demonstrated that the active learning strategy was superior to the traditional supervised learning strategy in cost reduction and improvement of image labeling quality. The related data and source code are freely available at https://github.com/hqyone/cancer_rcnn.
MoreTranslated text
Key words
cervical cancer screening,cervical cancer,deep convolutional neural networks,convolutional neural networks,active learning strategy
AI Read Science
Must-Reading Tree
Example
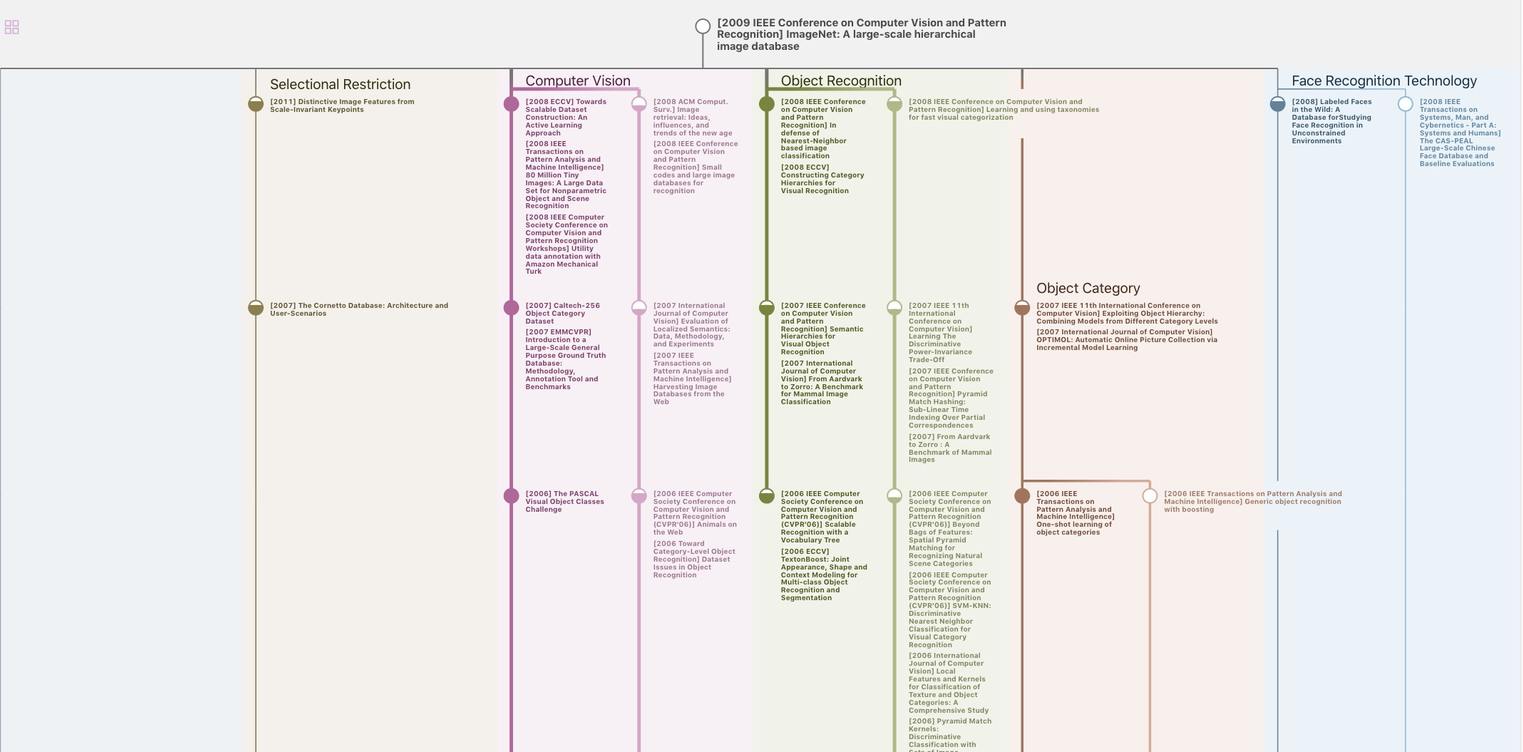
Generate MRT to find the research sequence of this paper
Chat Paper
Summary is being generated by the instructions you defined