Zero-Shot Composed Image Retrieval with Textual Inversion
2023 IEEE/CVF International Conference on Computer Vision (ICCV)(2023)
摘要
Composed Image Retrieval (CIR) aims to retrieve a target image based on a query composed of a reference image and a relative caption that describes the difference between the two images. The high effort and cost required for labeling datasets for CIR hamper the widespread usage of existing methods, as they rely on supervised learning. In this work, we propose a new task, Zero-Shot CIR (ZS-CIR), that aims to address CIR without requiring a labeled training dataset. Our approach, named zero-Shot composEd imAge Retrieval with textuaL invErsion (SEARLE), maps the visual features of the reference image into a pseudo-word token in CLIP token embedding space and integrates it with the relative caption. To support research on ZS-CIR, we introduce an open-domain benchmarking dataset named Composed Image Retrieval on Common Objects in context (CIRCO), which is the first dataset for CIR containing multiple ground truths for each query. The experiments show that SEARLE exhibits better performance than the baselines on the two main datasets for CIR tasks, FashionIQ and CIRR, and on the proposed CIRCO. The dataset, the code and the model are publicly available at https://github.com/miccunifi/SEARLE .
更多查看译文
关键词
Image Retrieval,Content-Based Image Retrieval,Image Captioning,Cross-Modal Retrieval,Feature Matching
AI 理解论文
溯源树
样例
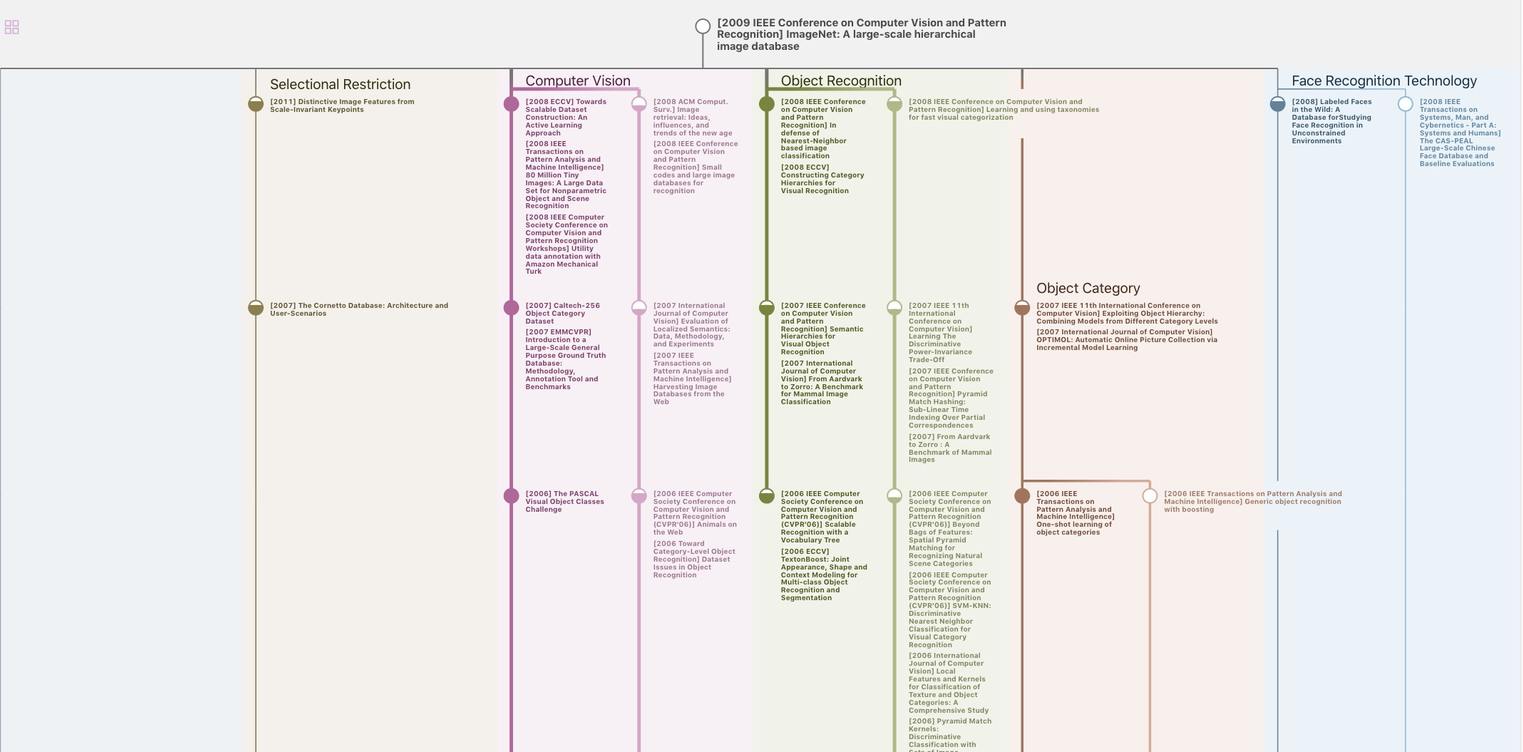
生成溯源树,研究论文发展脉络
Chat Paper
正在生成论文摘要