Probing optimisation in physics-informed neural networks
CoRR(2023)
摘要
A novel comparison is presented of the effect of optimiser choice on the accuracy of physics-informed neural networks (PINNs). To give insight into why some optimisers are better, a new approach is proposed that tracks the training trajectory curvature and can be evaluated on the fly at a low computational cost. The linear advection equation is studied for several advective velocities, and we show that the optimiser choice substantially impacts PINNs model performance and accuracy. Furthermore, using the curvature measure, we found a negative correlation between the convergence error and the curvature in the optimiser local reference frame. It is concluded that, in this case, larger local curvature values result in better solutions. Consequently, optimisation of PINNs is made more difficult as minima are in highly curved regions.
更多查看译文
关键词
neural networks,optimisation,physics-informed
AI 理解论文
溯源树
样例
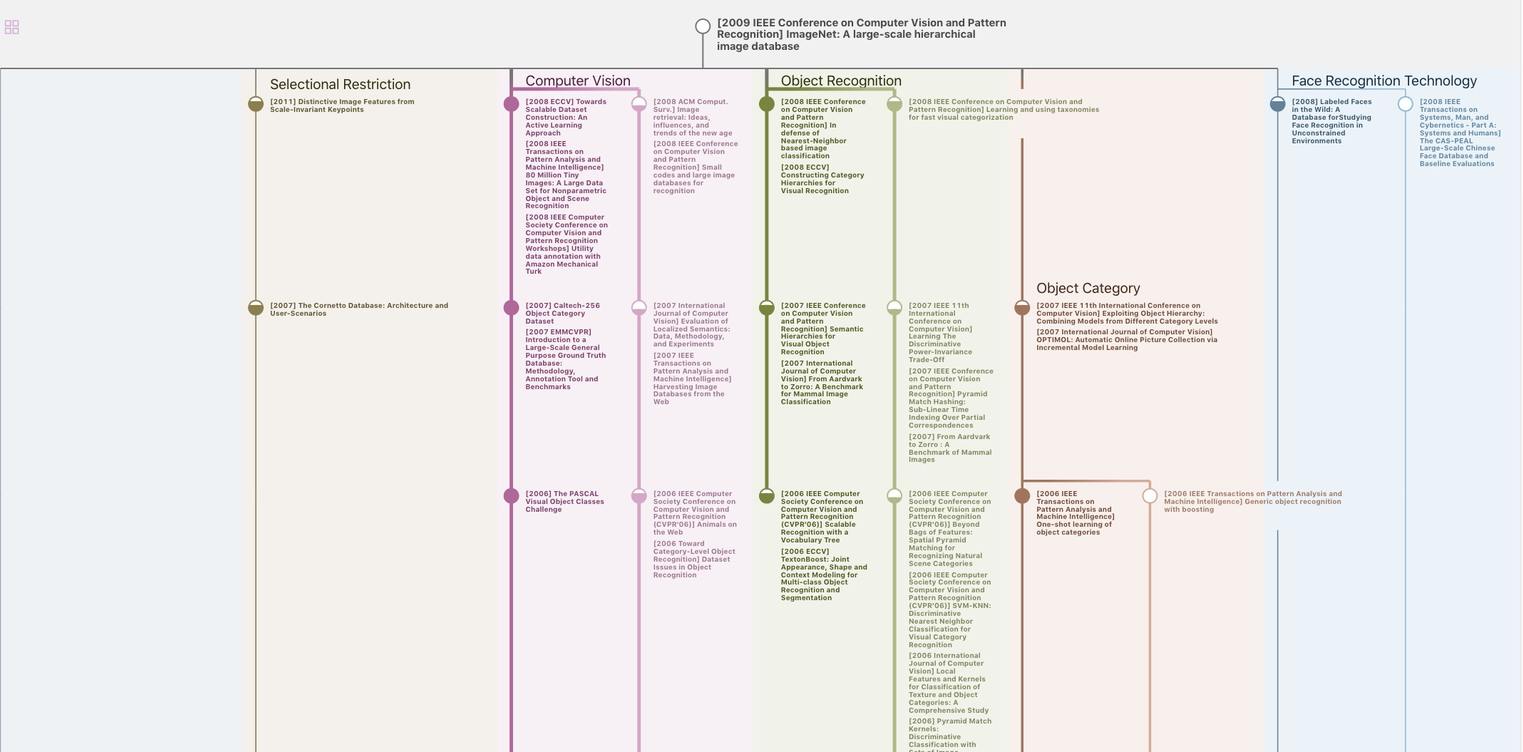
生成溯源树,研究论文发展脉络
Chat Paper
正在生成论文摘要