Pushing the Envelope for Depth-Based Semi-Supervised 3D Hand Pose Estimation with Consistency Training
CoRR(2023)
摘要
Despite the significant progress that depth-based 3D hand pose estimation methods have made in recent years, they still require a large amount of labeled training data to achieve high accuracy. However, collecting such data is both costly and time-consuming. To tackle this issue, we propose a semi-supervised method to significantly reduce the dependence on labeled training data. The proposed method consists of two identical networks trained jointly: a teacher network and a student network. The teacher network is trained using both the available labeled and unlabeled samples. It leverages the unlabeled samples via a loss formulation that encourages estimation equivariance under a set of affine transformations. The student network is trained using the unlabeled samples with their pseudo-labels provided by the teacher network. For inference at test time, only the student network is used. Extensive experiments demonstrate that the proposed method outperforms the state-of-the-art semi-supervised methods by large margins.
更多查看译文
关键词
3d,depth-based,semi-supervised
AI 理解论文
溯源树
样例
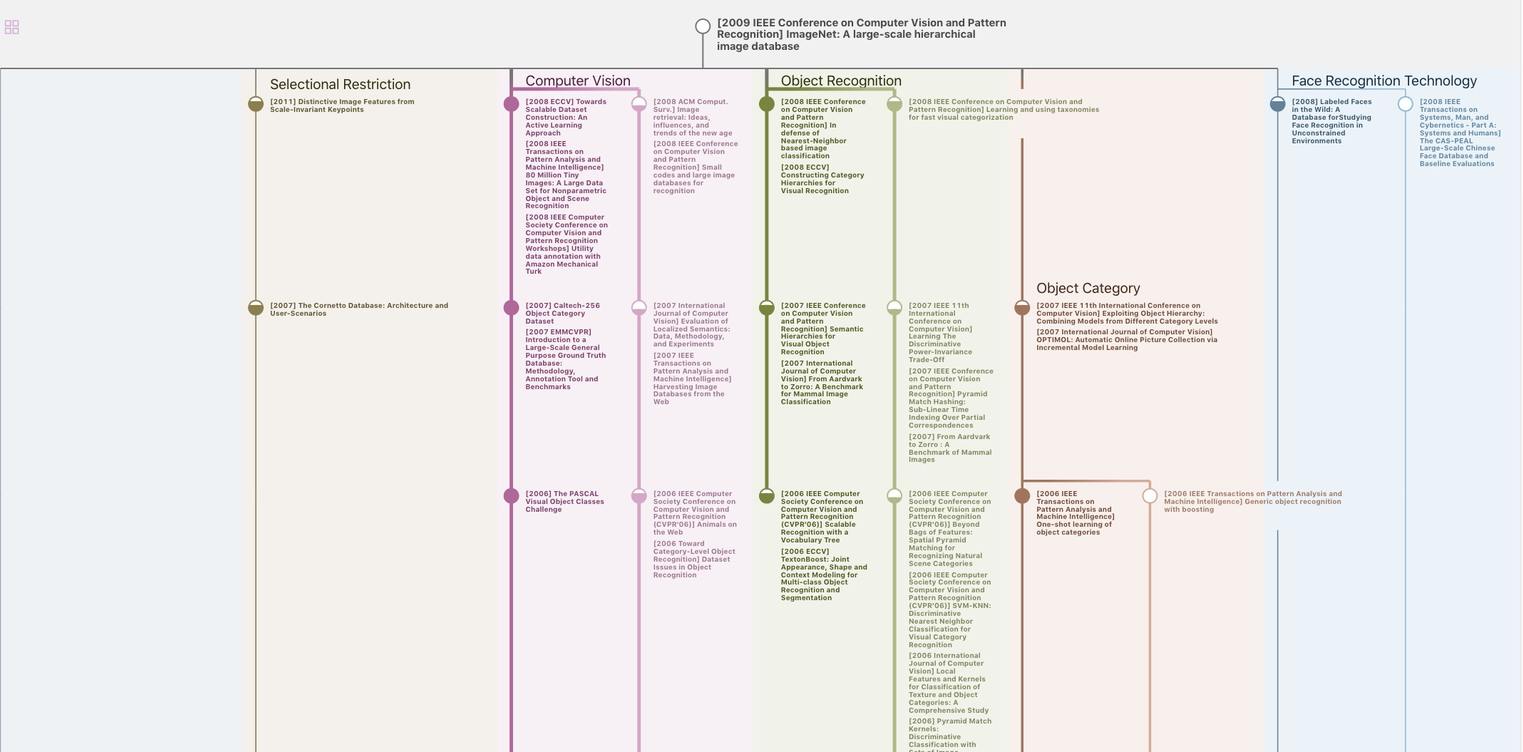
生成溯源树,研究论文发展脉络
Chat Paper
正在生成论文摘要